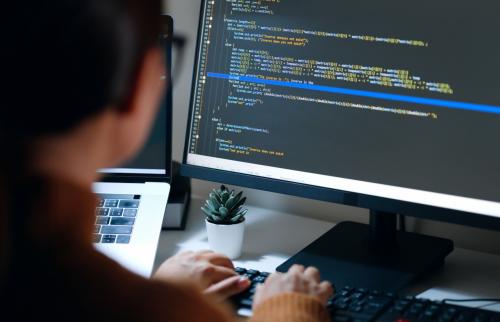
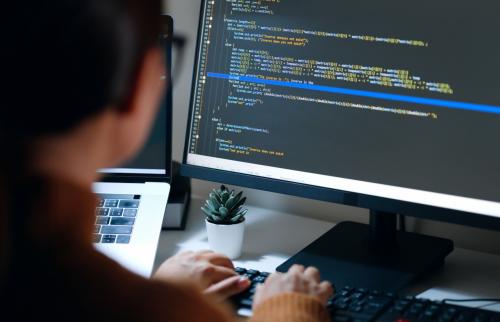
10:00 am EDT - 11:00 am EDT
Past Event
By nature, machine-learning algorithms rely upon the differential treatment of online users which are customized and optimized to their unique digital footprints. Yet, such treatment can embolden online behavioral biases, including ad targeting or the denial of loans and other financial services. From targeted advertising to more predatory eligibility determinations, consumers tend to be the subjects of machine-learning algorithms, with limited agency and feedback on the predictive accuracy of the computational models.
Senior Fellow and Director of the Center for Technology Innovation Nicol Turner Lee argues in a newly released book chapter, “Mitigating Algorithmic Biases through Incentive-Based Rating Systems,” that consumers’ feedback needs to be collected by developers and other stakeholders that license algorithms to ensure the trustworthiness of artificial intelligence (AI) systems. Modeled in part after the U.S. federal government’s Energy Star program, her work introduces a new incentive-based rating system to drive more informed consumer choices in their use of AI systems and improve upon the efficacy and inclusiveness of these models, especially among stakeholders desiring to reduce reputational harms due to flawed and biased systems.
On October 17, the Center for Technology Innovation at Brookings hosted a panel exploring how incentive-based rating systems, reputation badges, and other consumer-facing callouts can improve the trustworthiness of AI systems, while encouraging more participatory engagement in the design and execution of AI models.
Viewers submitted questions for speakers by emailing [email protected] or via Twitter at @BrookingsGov by using #OnlineBiasRatings.
Moderator
Panelist
Tania Babina, Anastassia Fedyk
July 1, 2025
John Villasenor
June 30, 2025
Darrell M. West
June 24, 2025