This report from The Brookings Institution’s Artificial Intelligence and Emerging Technology (AIET) Initiative is part of “AI and Bias,” a series that explores ways to mitigate possible biases and create a pathway toward greater fairness in AI and emerging technologies.
While Facebook profiles may not explicitly state users’ race or ethnicity, my research demonstrates that Facebook’s current advertising algorithms can discriminate by these factors. Based on research conducted in 2020 and 2021, I used Facebook’s advertising tools to test how advertisers can use their targeting options like “multicultural affinity” groups, Lookalike Audiences, and Special Ad Audiences to ensure their ads are reaching white, African American, Asian, or Hispanic users. What I found is that discrimination by race and ethnicity on Facebook’s platforms is a significant threat to the public interest for two reasons. First, it is a violation of the existing civil rights laws that protect marginalized consumers against advertising harms and discrimination by race and ethnicity, especially in the areas of housing, employment, and credit. Second, these same Facebook advertising tools can be used to disseminate targeted misinformation and controversial political messages to vulnerable demographic groups. To solve these concerns, regulators, advocacy groups, and industry must directly address these issues with Facebook and other advertising platforms to ensure that online advertising is transparent and fair to all Americans.
Racial Bias and Discrimination in Facebook Ad Targeting
Facebook’s long-standing complaints in advertising
Over the last 5 years, Facebook has faced repeated criticism, lawsuits, and controversies over the potential for discrimination on its ad platform. Journalists have demonstrated how easy it is to exclude users whom Facebook has algorithmically classified into certain racial or ethnic affinity groups from being targeted by housing or employment ads. Researchers have also demonstrated racial and ethnic biases in Facebook’s Lookalike Audience and Special Ad Audience algorithms, which identify new Facebook users similar to an advertiser’s existing customers. In response to these allegations, Facebook has been sued by the National Fair Housing Alliance, the ACLU, the Communications Workers of America, the U.S. Department of Housing and Urban Development (HUD), and others over issues of discrimination on its advertising platform and violations of civil rights laws such as the Fair Housing Act and the Civil Rights Act of 1964, which expresses intolerance of any types of racial discrimination.
There are also ongoing controversies over how Facebook’s platform can be used by political actors, both foreign and domestic, to spread misinformation and target racial and ethnic minorities in the 2016 and 2020 election cycles. In July 2020, a high-profile boycott of Facebook’s advertising platform to “Stop Hate for Profit” was organized by civil rights and advocacy groups including the NAACP, the Anti-Defamation League, Color of Change, and other organizations over misinformation and civil rights violations. These groups called on major corporations to stop advertising on Facebook for the entire month of July. More than 1,000 large companies including Microsoft, Starbucks, Target, and others participated in the boycott.
On July 8, 2020, Facebook released its own civil rights audit conducted by Laura Murphy, former Director of the ACLU Legislative Office, and attorneys at the law firm Relman Colfax. The audit criticized Facebook for having “placed greater emphasis on free expression” over the “value of non-discrimination.” Seeking to make concrete steps towards reducing discrimination, on August 11, 2020, Facebook announced that it would retire its controversial “multicultural affinity” groups that allowed advertisers to target users whom Facebook has categorized as “African American (US),” “Asian American (US),” or “Hispanic (US – All).”
has Facebook sufficiently addressed discriminatory ad harms?
No.
Not every case of advertising discrimination may be illegal. Keeping this in mind, I devised a research study and tested the degree to which the different tools on Facebook’s ad platform can—intentionally or not—carry out racial and ethnic discrimination, following the Fair Housing Act’s criteria which makes it illegal to publish an ad that indicates “any preference, limitation, or discrimination” based on race or ethnicity.
To date, Facebook provides advertisers with four major ways to target advertisements:
- “Detailed Targeting” options are prepackaged groups of Facebook users who share common attributes based on Facebook’s data analysis of their behaviors online,
- “Custom Audiences” allow an advertiser to upload their own list of customers or individuals for Facebook to target,
- “Lookalike Audiences” allow an advertiser to reach people who Facebook determines to be similar to a designated Custom Audience, and
- “Special Ad Audiences” allow an advertiser to create a Lookalike Audience that finds people similar to their designated Custom Audience in online behavior without considering sensitive attributes like age, gender, or ZIP code, specifically for housing, employment, and credit ads which are regulated by anti-discrimination laws.
I conducted my study in January 2020 and again in January 2021, before and after the Stop Hate for Profit boycott in July 2020. As Facebook no longer offers multicultural affinity groups – “African American (US),” “Asian American (US),” and “Hispanic (US – All)” – as targeting options for advertisers in 2021, I instead examined the similar-sounding cultural interest groups that Facebook still offered as targeting options, such as “African-American Culture,” “Asian American Culture,” and “Hispanic American Culture.” In both years, I tested how many minority users could be targeted by these race- and ethnicity-related advertising options by Facebook.
Figure 1: Example of the difference in Facebook’s ad-targeting options, 2020 to 2021
Year Available | Targeting Option | Targeting Category Hierarchy | Description | Size |
---|---|---|---|---|
2020 | African American (US) | Behaviors > Multicultural Affinity | People who live in the United States whose activity on Facebook aligns with African American multicultural affinity | 87,203,689 |
2021 | African-American culture | Interests > Additional Interests | People who have expressed an interest in or like pages related to African-American culture | 79,388,010 |
Note: Facebook offered the “African American (US)” targeting option until August 2020, but it has continued to offer the “African-American Culture” targeting option in 2021. |
I also tested if Facebook’s other advertising tools, such as Lookalike Audiences and Special Ad Audiences, can discriminate by race and ethnicity. For these tests, I used the most recent North Carolina voter registration dataset, which has voter-provided race and ethnicity information. In the North Carolina voter data, there were approximately 4.0 million white, 1.2 million African American, 90,000 Asian, and 180,000 Hispanic active voters. I created different racially and ethnically homogenous sub-samples of voters with Facebook Custom Audiences and then asked Facebook to find additional similar users to target for ads using their Lookalike and Special Ad Audience tools.
While Facebook doesn’t permit direct demographic queries for a Lookalike or Special Ad audience, I was able to leverage the daily reach estimates of Facebook’s ad planning tool to indirectly observe the racial and ethnic breakdown of a given Lookalike or Special Ad audience amongst North Carolina voters. For example, a Lookalike audience based on 10,000 African American voters in 2021 had an overlap in estimated daily reach of 139,000 users with a sample of 1 million African American voters but only an overlap of 17,000 users with a sample of 1 million white voters. This indicates that African Americans were likely over-represented in the Lookalike audience, since when intersecting the Lookalike audience with a combined 2 million voter sample that was 50% African American and 50% white at baseline, I found that 89% of the Lookalike audience’s overlap was with African American voters and only 11% with white voters. I replicated a similar process for testing the demographics of Lookalike and Special Ad Audiences based on white, Asian, and Hispanic voters. More details on the methodology can be found here.
Figure 2: Overlap of a Facebook Lookalike Audience based on African-Americans with race-based North Carolina voter samples
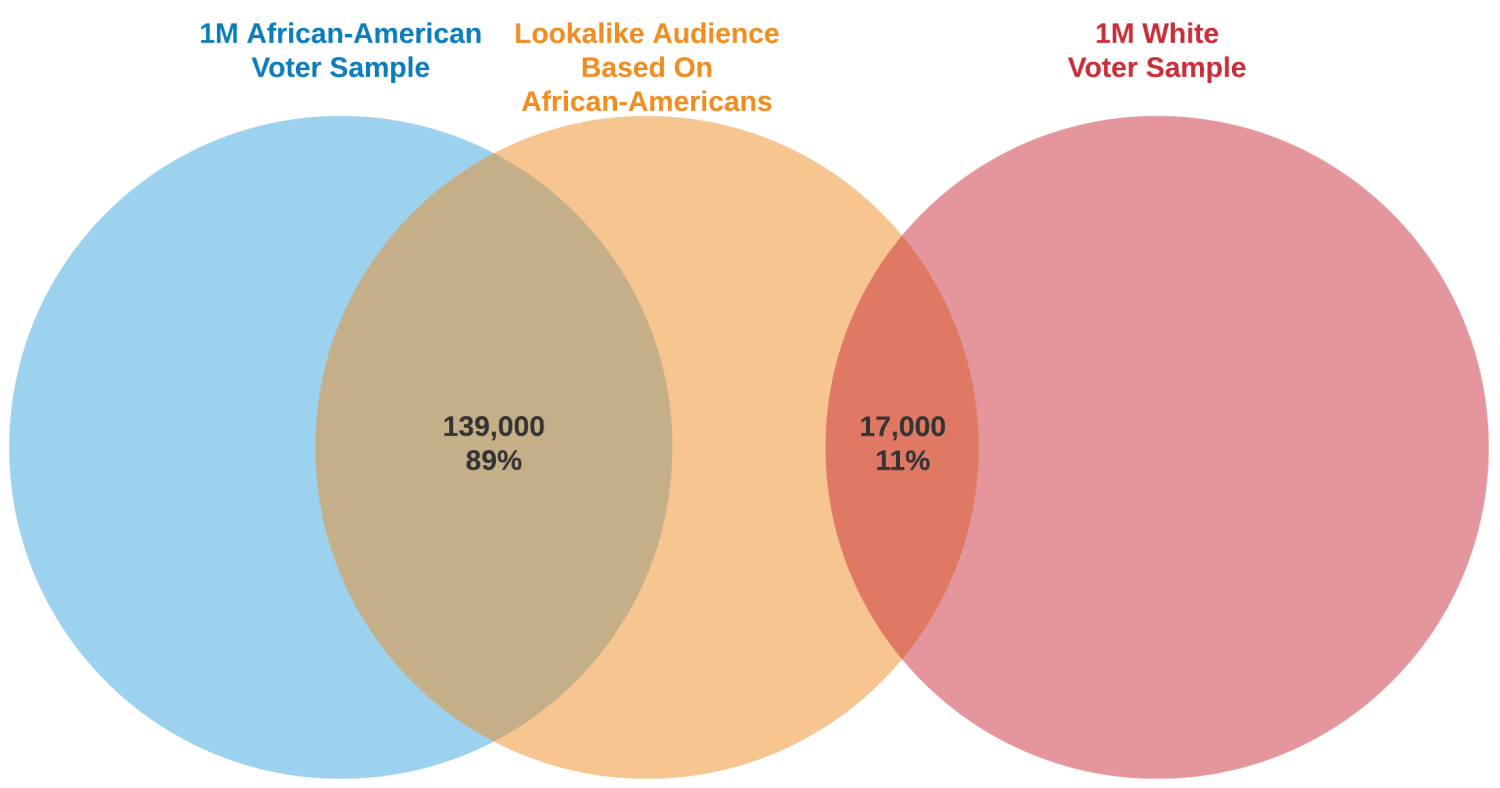
A major concern about algorithmic discrimination is whether computers are reinforcing the existing patterns of discrimination that humans have made in the United States. Social science researchers have found in employment, housing, lending, and other aspects of life, racial proxies such as one’s name and neighborhood can increase the degree to which human decision-makers discriminate. Thus, I used a frequently-cited computer algorithm trained on the names of 13 million voters in Florida to identify commonly used names for each demographic group and U.S. Census data to identify ethnic enclave ZIP codes. Then, I tested if using voter samples based on commonly-used racial proxies such as names and ZIP codes can affect the degree of bias in the resulting Lookalike and Special Ad Audiences. For example, would a Lookalike audience based on African American voters with common African American names and who live in majority African American ZIP codes be even more likely to over-represent African Americans above the 50% baseline?
Facebook still has a discrimination problem by race and ethnicity on its advertising platform
My research study of Facebook’s ad platform in 2020 and 2021 had three major findings. Facebook’s ad platform still offers multiple ways for discrimination by race and ethnicity despite the historic boycott it faced in 2020, and bad actors can exploit these vulnerabilities in the new digital economy.
Finding 1: The racial and ethnic targeting options by Facebook were even more accurate in 2021 than in 2020.
In 2021, certain racial and ethnic cultural interest groups that Facebook still offered to advertisers were even more accurate in targeting minority users than the old “multicultural affinity” groups Facebook retired in August 2020. For example, in 2020, 150,000 African American voters in North Carolina could be reached by Facebook’s “African American (US)” targeting option each day, which is slightly more than the 142,000 who can be reached by Facebook’s “African-American Culture” targeting option in 2021. However, there was a dramatic decrease in the number of white voters who can be reached by the same targeting options, from 428,000 in 2020 to just 109,000 in 2021. This means that in 2020, Facebook’s “African American (US)” targeting option was likely to reach nearly three times as many white users as African American users, while in 2021, Facebook’s “African-American Culture” targeting option became significantly more accurate in reaching nearly a same number of African American users while targeting 75% fewer white users.
Figure 3: Number of North Carolina voters by race reached by Facebook’s African American related targeting options, 2020 and 2021
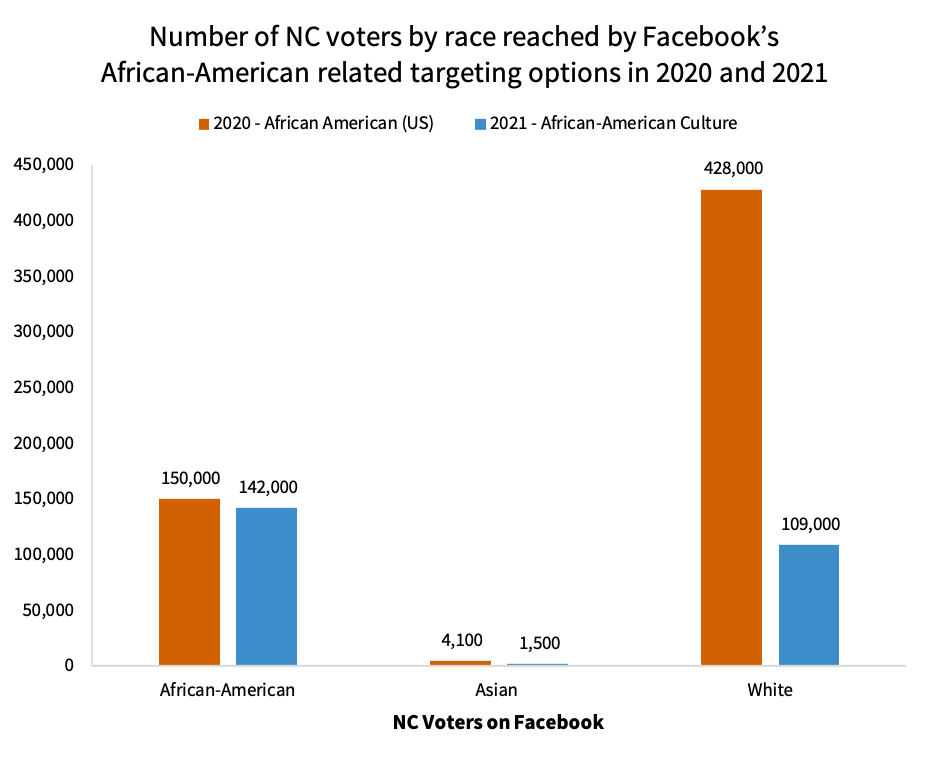
Finding 2: Facebook’s algorithms to find new audiences for an advertiser permit strong racial and ethnic biases, which includes the algorithm that Facebook explicitly designed to avoid discrimination.
Facebook’s Lookalike and Special Ad Audiences can be biased by race and ethnicity in both 2020 and 2021 depending on the demographics of a customer list that an advertiser provides to Facebook. For example, in 2020, a Lookalike audience based on African American voters in North Carolina had a sample share of 83% African Americans, which increased to 89% in 2021. Thus, these Lookalike Audiences significantly over-represented African Americans above the expected 50% baseline sample share (Figure 4). Similarly, Lookalike Audiences based on white voters had a sample share of 73% whites in 2020 and 71% in 2021, significantly over-representing whites above the 50% baseline. Other tests found that Lookalike Audiences based on Asian or Hispanic voters would also significantly over-represent Asians or Hispanics above the expected baseline share.
I also found a high degree of racial and ethnic bias in testing the Special Ad Audience tool that Facebook designed to explicitly avoid using sensitive demographic attributes when finding similar users to an advertiser’s customer list. For example, in 2020, a Special Ad audience based on African American voters had a sample share of 83% African Americans, which decreased slightly to 76% in 2021 (Figure 4). Special Ad Audiences based on white voters had a similarly high sample share of 83% whites in 2020 and 81% in 2021. Finally, I also found that Special Ad Audiences based on Hispanic voters significantly over-represented Hispanics in both 2020 and 2021. This finding is especially problematic since it means that advertisers for housing, credit, and employment can use the Special Ad audience tool that Facebook has created for these legally protected sectors to pursue discrimination by race and ethnicity. This also undermines the goals of the 2019 legal settlement between Facebook and the ACLU, which required Facebook to create an alternative ad targeting solution for these sectors where an advertiser “cannot target ads based on Facebook users’ age, gender, race, or categories that are associated with membership in protected groups, or based on ZIP code or a geographic area”.
Finding 3: Racial proxies such as names and ZIP codes can increase the bias of Facebook’s ad targeting algorithms.
In both 2020 and 2021, the degree of racial and ethnic bias in Facebook’s Lookalike and Special Ad Audiences is higher when the ad targeting algorithm is trying to find users similar to individuals with racially stereotypical traits such as their name or neighborhood. For example, a Lookalike audience based on African American voters with commonly-given African American names and who live in majority African American ZIP codes had a very high sample share of 93% African Americans in 2020, which increased to 94% in 2021 (Figure 4). In the most extreme case, in 2021, I found that a Lookalike audience based on Asian voters with commonly-given Asian names and who live in popular Asian ZIP codes had a sample share of 100% Asians.
I also found that using racially stereotypical names and ZIP codes can embolden the opportunities for more precise discrimination in the use of Special Ad Audiences. For example, in 2020, a Special Ad audience based on African American voters with commonly-given African American names and who live in majority African American ZIP codes had a sample share of 97% African Americans, which was even higher than the 83% sample share observed when testing the Special Ad audience based on a generic sample of African American voters (Figure 4).
Figure 4: Sample share of African-American voters in Lookalike Audiences (top) and Special Ad audiences (bottom) based on lists of North Carolina voters with different traits
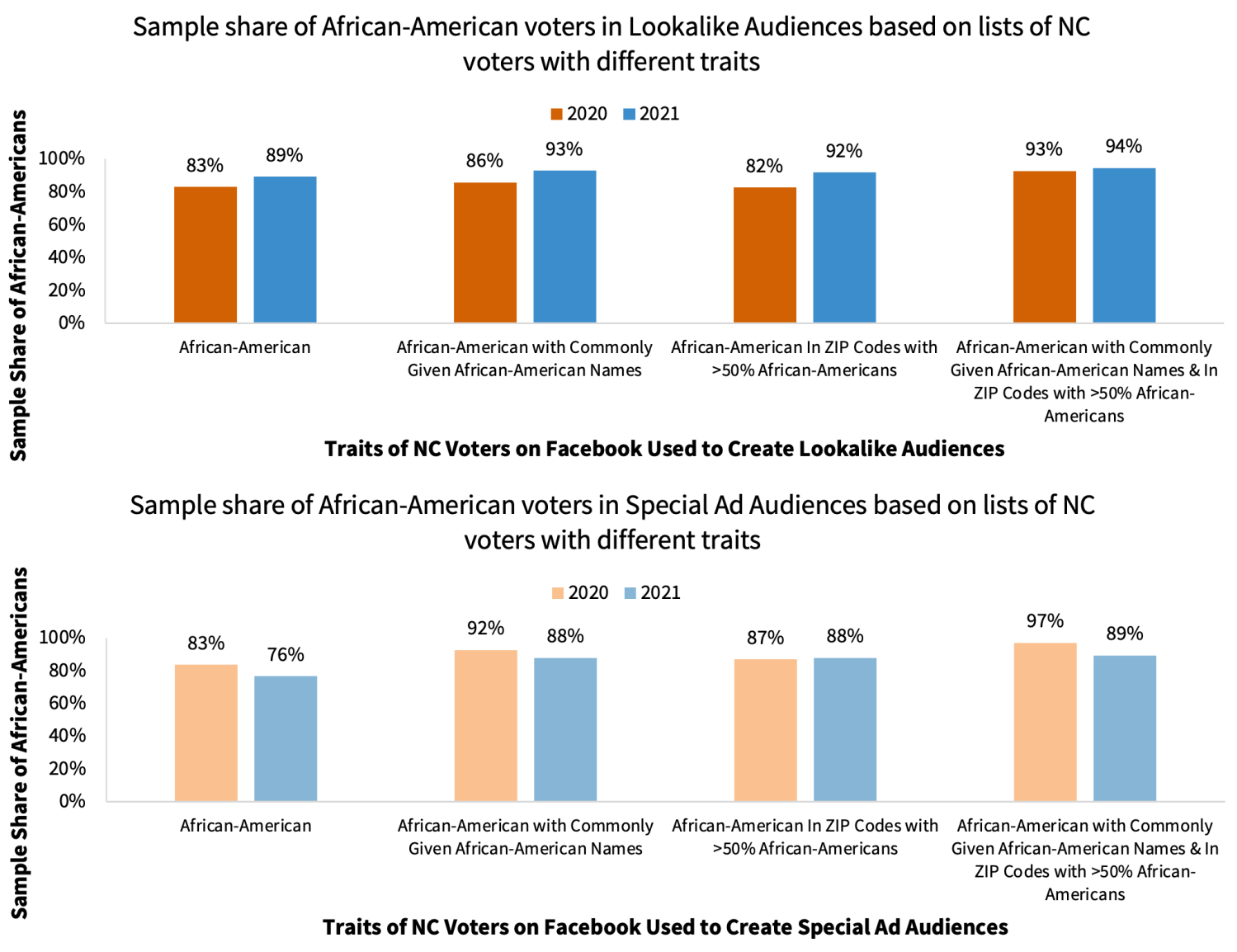
What’s causing these research findings?
Based on algorithmic discrimination research, it’s likely that a combination of multiple causes contributed to the biased outcomes observed in this study. One of the primary factors is that computer algorithms tend to replicate existing patterns and behaviors that already exist in society. Facebook’s Lookalike and Special Ad Audience algorithms are using the enormous amounts of data that Facebook has about its users in order to identify which users are most similar to one another and thus most likely to respond well to the same type of ads. When it comes to demographic groups, researchers have found that racial and ethnic groups tend to behave differently from each other online and that Americans tend to have very racially homogenous friend networks. Thus, users within a racial or ethnic group may appear more alike to one another in the eyes of Facebook’s algorithms than users in different groups to each other. However, this doesn’t mean that Facebook should facilitate the discriminatory targeting of racial and ethnic minorities with ads, nor that commonly-used racial proxies such as name or ZIP code should also influence the digital world created by Facebook’s advertising algorithms. After all, hard-fought civil rights laws in the U.S. have intentionally elevated society’s interest in reducing discrimination above the private interest of landlords, employers, and lenders to maximize profits through possible discrimination. It would be taking a step backwards to make new allowances for discrimination on Facebook simply because the decision-maker is now a computer instead of a human.
Given these findings, policy makers have a role in mitigating the biases being activated by companies like Facebook and other advertisers reliant on online tools.
Policymakers, regulators, and civil society groups should demand and require greater transparency by Facebook and its advertisers
Clearly, at the heart of the problems, is the lack of transparency by Facebook to the public and to its advertisers about how its ad platform can potentially discriminate by race and ethnicity. This may be exploited by discriminatory advertisers while undermining the goals of non-discriminatory ones.
For example, discriminatory advertisers may already know that the “African-American Culture” targeting option contains fewer white users than the “African American (US)” option Facebook removed in 2020. Discriminatory advertisers may also be using similar proxy variable techniques to the ones tested in this study based on racially stereotypical names and ZIP codes to create biased Lookalike and Special Ad Audiences. On the other hand, non-discriminatory advertisers may be unintentionally choosing similar targeting settings as discriminatory ones but are unaware of how Facebook’s ad platform is carrying out racially and ethnically biased targeting on their behalf.
Facebook can reverse this lack of transparency by more corporate accountability, transparency statement, disclosure to advertisers, and robust anti-discrimination engineering. However, it’s vitally important that regulators, advocacy groups, and industry groups can respond to improve upon such outcomes.
Recommendation 1: Require Facebook and advertisers to release their ad targeting data.
Advertising platforms like Facebook should provide greater transparency about the way that advertisers are using their tools to target ads. Regulators can require Facebook’s Ad Library for political, housing, employment, and credit-related ads to show the relevant metadata for establishing a “robust causal link” for racial or ethnic discrimination lawsuits. This is especially important since the Department of Housing and Urban Development’s (HUD) “Implementation of the Fair Housing Act’s Disparate Impact Standard” published on September 24, 2020 now requires a plaintiff to present evidence of a “robust causal link” in order to bring a disparate impact discrimination lawsuit in the first place. Facebook has begun to share relevant metadata for political ads with approved researchers in 2021, but doesn’t currently release any ad targeting metadata for housing, employment, and credit-related ads.
Recommendation 2: Conduct regular algorithmic bias auditing of Facebook’s ad platform.
Future civil rights audits of Facebook’s platform should test its technologies for algorithmic bias based on race, ethnicity, gender, sexual orientation, and other protected classes. In July 2020, Facebook released its first civil rights audit primarily focused on political speech, misinformation, and legal issues during the Stop Hate for Profit boycott. The civil rights groups organizing the boycott called for regular audits of Facebook’s platform. These future civil rights audits can build on the technical efforts by myself and other researchers. Ideally, if provided even deeper access to Facebook’s data and systems, these audits can go even further in examining why these racial and ethnic biases exist and how to address them. Since 2014, many large U.S. tech companies such as Facebook, Apple, Google, Microsoft, Amazon, Twitter, and others have participated in the related norm of releasing annual workforce diversity reports. Federal regulators such as the Federal Trade Commission (FTC) can also audit Facebook’s ad platform for discrimination and use their enforcement powers to address violations of Section 5 of the FTC Act, the Fair Credit Reporting Act, and the Equal Credit Opportunity Act.
Recommendation 3: Design anti-discrimination solutions using the more effective “fairness through awareness” approach.
Technology and technology policy need to move beyond “fairness through unawareness”—the idea that discrimination is prevented by eliminating the use of protected class variables or close proxies—in order to actually address algorithmic discrimination. For example, Facebook created the Special Ad Audiences tool as an alternative to Lookalike Audiences in order to explicitly not use sensitive attributes such as “age, gender or ZIP code” when considering which users are similar enough to the source audience to get included. However, my research demonstrates that Special Ad Audiences based on African Americans or whites can be biased towards the race that is more dominant in the customer list used to create the audience, just like the corresponding Lookalike Audiences.
Statistics research has labeled this phenomenon as the Rashomon or the multiplicity effect. Given a large dataset with many variables, there exists a large number of potential models that can perform approximately to equally as well as a prohibited model that uses protected class variables. Thus, even though the Special Ad Audiences algorithm for finding similar users to a customer list does not use demographic attributes in the same way as the Lookalike Audiences algorithm, the two algorithms may end up making functionally comparable decisions on which users are considered to be similar enough to get included.
There are public policy risks from continuing to implement a “fairness through unawareness” standard, which has been shown to be statistically ineffective. For example, the initially proposed language on August 19, 2019 by the Department of Housing and Urban Development (HUD) for its updated Disparate Impact Standard, would likely have wrongfully protected companies like Facebook from being sued for discrimination simply because its Special Ad Audience algorithm follows “fairness through unawareness” and does not rely on “factors that are substitutes or close proxies for protected classes under the Fair Housing Act.”
Instead, the more effective approach is to use “fairness through awareness” to design anti-discrimination tools, which could then accurately consider the experiences of different racial and ethnic groups on the platform. Thus, tech companies such as Facebook would need to learn the demographic information of their users. One way is to directly ask their users to provide their race and ethnicity on a voluntary basis, like with LinkedIn Self-ID. Another way is to use algorithmic or human evaluators to generate that information for their anti-discrimination testing tools. This was the approach taken by Airbnb’s Project Lighthouse, launched in 2020 to study the racial experience gap for guests and hosts on Airbnb. Project Lighthouse used a third-party contractor to assess the perceived race of an individual based on their name and profile picture. In December 2009, Facebook researchers used a related methodology by comparing the last names of Facebook users to the U.S. Census’ Frequently Occurring Surnames dataset in order to demonstrate how Facebook was becoming increasingly diverse over time by having more African American and Hispanic users.
The harms from discriminatory advertising on Facebook that target specific demographic groups are real. Facebook ads have reduced employment opportunities, sowed political division and misinformation, and even undermined public health efforts to respond to the COVID-19 pandemic. My research shows how Facebook continues to offer advertisers myriad tools to facilitate discriminatory ad targeting by race and ethnicity, despite the high-profile Stop Hate for Profit boycott of July 2020. To address this issue, regulators and advocacy groups can enforce and demand greater ad targeting transparency, more algorithmic bias auditing, and a “fairness through awareness” approach by Facebook and its advertisers.
In July 2020, Facebook Chief Operating Officer Sheryl Sandberg stated that its civil rights audit was “the beginning of the journey, not the end.” Faced with a long road ahead, it’s time for Facebook to continue down the path to create more equitable and less harmful advertising systems.
The Brookings Institution is a nonprofit organization devoted to independent research and policy solutions. Its mission is to conduct high-quality, independent research and, based on that research, to provide innovative, practical recommendations for policymakers and the public. The opinions expressed in this paper are solely those of the author in their personal capacity and do not reflect the views of Brookings or any of their previous or current employers.
Microsoft provides support to The Brookings Institution’s Artificial Intelligence and Emerging Technology (AIET) Initiative, and Amazon, Apple, Facebook, and Google provide general, unrestricted support to the Institution. The findings, interpretations, and conclusions in this report are not influenced by any donation. Brookings recognizes that the value it provides is in its absolute commitment to quality, independence, and impact. Activities supported by its donors reflect this commitment.