As researchers grew to understand COVID-19 during the early days of the pandemic, many built AI algorithms to analyze medical images and measure the extent of the disease in a given patient. Radiologists proposed multiple different scoring systems to categorize what they were seeing in lung scans and developed classification systems for the severity of the disease. These systems were developed and tested in clinical practice, published in academic journals, and modified or revised over time. But the pressure to quickly respond to a global pandemic threw into stark relief the lack of a coherent regulatory framework for certain cutting-edge technologies, which threatened to keep researchers from developing new diagnostic techniques as quickly as possible.
Radiology has long been one of the most promising branches of AI in medicine, as algorithms can improve traditional medical imaging methods like computed tomography (CT), magnetic resonance imaging (MRI), and x-ray. AI offers computational capabilities to process images with greater speed and accuracy and can automatically recognize complex patterns in assessing a patient’s health. But since AI algorithms can continually learn from the medical images they review, the traditional approach to reviewing and approving upgrades to software that is used for diagnosing, preventing, monitoring, or treating diseases such as COVID-19 may not be appropriate. As the course of the public-health response to the pandemic is beginning to shift, radiology is continuing to advance our understanding of the disease (such as the mechanisms by which COVID patients suffer neurological issues like brain fog, loss of smell, and in some cases serious brain damage) and beginning to account for some of the costs of our response (such as the fact that the body’s response to vaccines may cause false positives for cancer diagnosis).
In a recent policy brief for Stanford University’s Institute for Human-Centered Artificial Intelligence, we explore the path forward in building an appropriate testing framework for medical AI and show how medical societies should be doing more to build trust in these systems. In suggesting changes for diagnostic AI to reach its full potential, we draw on proposals from the Food and Drug Administration in the United States, the European Union, and the International Medical Device Regulators Forum to regulate the burgeoning “software as a medical device” (SaMD) market. We recommend that policymakers and medical societies adopt stronger regulatory guidance on testing and performance standards for these algorithms.
Diagnosing the problem
AI-based diagnostic algorithms for medical imaging merit their own, new regulatory framework because current proposals fail to account for the differences between AI algorithms and medical devices of the past. As AI algorithms continually learn from the medical images they review, traditional approaches to reviewing and approving upgrades to these systems may not translate into a responsible development paradigm. Instead, regulators will need to implement a protocol for regulating the entire lifecycle of AI-based algorithms. So far, the European Union has codified requirements for developers’ clinical evaluation reports and specified how manufacturers are to prepare and follow-up with post-market planning. The United States Food and Drug Administration recently published a working model for software precertification programs. The International Medical Device Regulators Forum has developed a host of suggestions for SaMD applications including recommendations to create separate risk categories tracking the degree of regulatory scrutiny a given program deserves, principles for creating quality management systems, principles for evaluation of clinical effectiveness, and recommendations for how clinical evaluation reports are to be drafted.
While these three proposals represent valuable first steps, they include a number of shortcomings, such as confusing the algorithm with the task it is designed to perform, relying on definitions of medical tasks that lack rigor, and using lax specifications that make it harder to directly compare similar algorithms, with resulting unpredictability in model performance. These proposals also fail to address the conflicts of interest at play for manufactures who develop, test, and mark devices, as well as the lack of resources to assess the performance of medical AI devices. Though the diagnostic task and the algorithm performing the task are closely linked, activities such as defining what constitutes pneumonia on a chest radiograph and the program that applies that definition in its automated analysis of x-rays are too frequently treated as one and the same. Similarly, because measurement and classification schemes like those developed for stroke imaging or for characterizing a patient’s blood flow were designed by a manufacturer without input from standard-setting bodies, existing task definitions frequently lack rigor, leading to the need for agreed-upon standards to facilitate widespread adoption.
Because diagnostic tasks were not originally intended to be performed by non-physicians, SaMD system developers are frequently left to interpret definitions and instructions without a firm grasp on the domain. Even when physicians perform this activity, their interpretations are likely to vary. This lack of deep expertise and consistency can lead to a lack of clarity with the underlying medical definitions, which hampers regulators’ ability to directly compare algorithms that ostensibly perform the same diagnostic task. Thus, the medical community should assume responsibility for standardizing, maintaining, and updating the nomenclature of each classification or measurement task. Once this is done, developers should be required to use those community standards and keep their algorithms up to date as standards evolve.
A parallel issue reflects a well-known problem with many other AI-based systems, in that these algorithms sometimes behave in unpredictable ways when used in settings different from the one in which they were tested or when new data is of a different quality from its validation testing. Similarly, the resources used to assess algorithm performance are not universally available and performance can vary substantially when reliability and safety assurances are lacking. These challenges are magnified by a range of conflicts of interest in the testing and validation procedure, such as manufacturers carefully curating—or even manipulating or augmenting—testing data to present for regulatory scrutiny.
Empowering medical societies
While identifying patients with signs of COVID-19 in their lungs may sound relatively straightforward, in reality, researchers and public health officials are confronted by a variety of scales to evaluate the likelihood and severity of the disease based on CT scans. Some of those scales range from 0 to 4, some from 0 to 6, and some from 0 to 25, which could be incorporated into an AI-based algorithm for the classification of image findings of COVID-19. These scoring mechanisms are based on extensive clinical experience and research and provide a window into how gaps in the evaluation frameworks for medical AI systems might be filled. Below we outline concrete steps policymakers should consider.
First and foremost, we should always distinguish the algorithm from the definition of the diagnostic task. If possible, these definitions should be based on commonly accepted standards, which are likely best developed by medical societies. We propose that definitions contain the following four elements: a background review of relevant information and medical objectives; a thorough description of the task that includes clinical assessment criteria, measurement definitions and descriptions, and the full universe of potential classification categories; detailed image labeling instructions for the task in question; and illustrated examples and relevant counterexamples for developers building these systems. It should be left to medical experts to specify additional companion references. In some cases, developers may need to propose and publish their own task definitions. True standardization will require the cooperative management of the ecosystem of related task definitions from medical professional societies rather than piecemeal evaluation or specification of definitions.
Second, measures of algorithmic performance cannot be based solely on accuracy. It is important to expand the definition of such performance to include elements like reliability, applicability, self-awareness of limitations, and more. In addition, algorithms should be subject to thorough evaluation before clinical deployment as well as continuous monitoring throughout the life cycle. Current SaMD systems have an alarming tendency to miss problems. Adding performance metrics beyond accuracy, such as transparency, use of fail-safes, and auditability will help those using and managing these systems to objectively assess the reliability of the algorithms and identify problems when they arise.
Standardizing the evaluation process
The third measure we advocate for is dividing the evaluation of medical AI systems into five discrete steps: diagnostic task definition, capacity of the algorithm to perform in a controlled environment, evaluation of effectiveness in the real world compared to performance in a controlled environment, validation of effectiveness in the local setting at each installed site, and durability testing and monitoring to ensure the algorithm performs well over time. After identifying the diagnostic task, assessing how capable a system is in performing its defined task in a controlled environment and comparing it with other competitors is a natural next step as illustrated in the figure below.
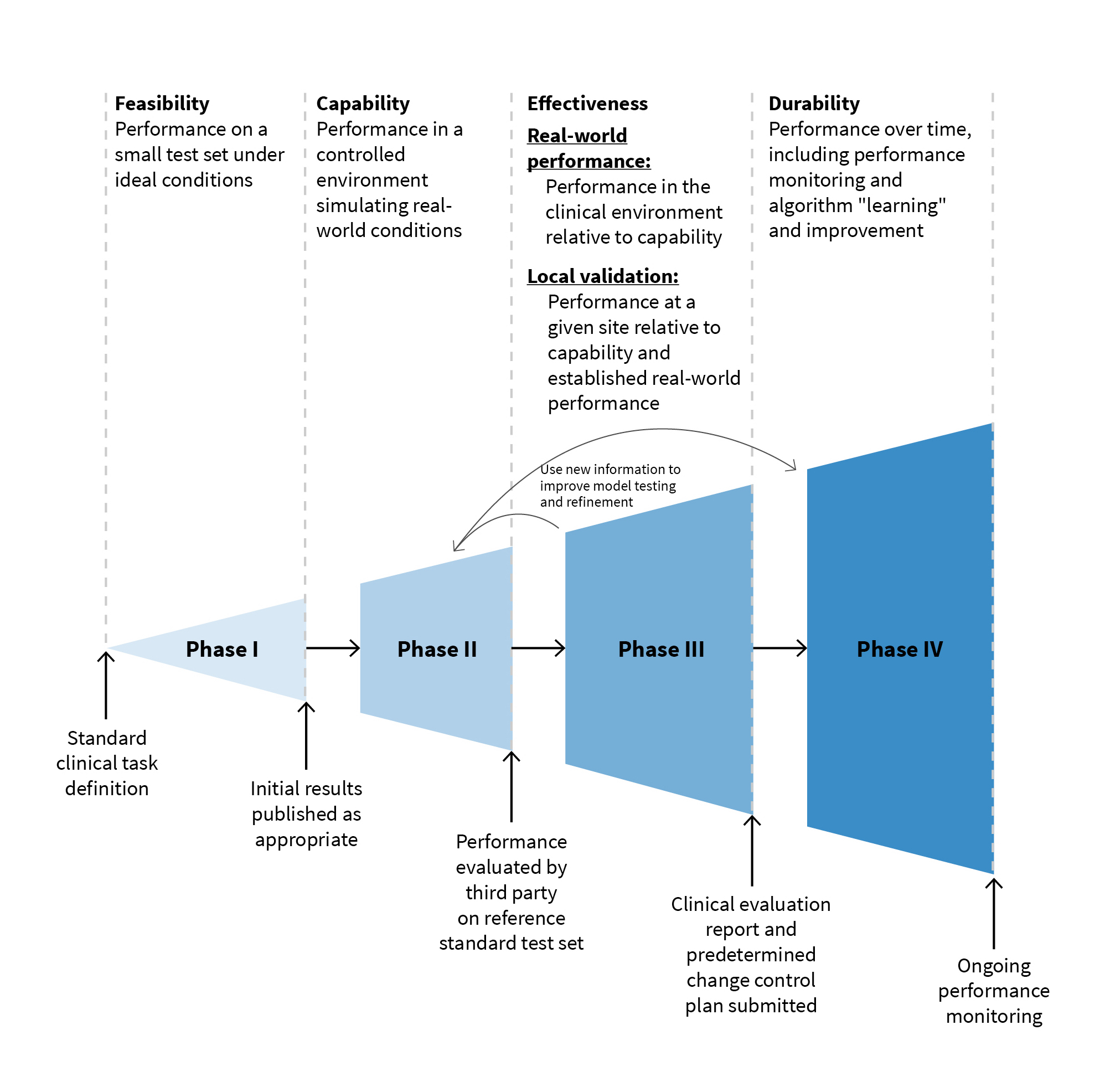
Developers can then compare their algorithm’s lab-tested capability with real-world performance before developing a more specific sense of real-world effectiveness in localized trials that don’t depend on a small number of closely monitored sites. Then and only then can developers accurately gauge how well the system performs over time, both in terms of accuracy and execution.
Fourth, independent assessment of these diagnostic systems by third-party evaluators should be encouraged. This independent assessment would address the conflicts of interest inherent in developing, evaluating, and marketing an AI-based SaMD application. These assessments could be performed by clinical research organizations, research laboratories, or other entities involved in the development and maintenance of reference data sets.
Lastly, we recommend that this phased development and evaluation process be incorporated into manufacturers’ development process just as the pharmaceutical industry has performed drug development studies reviewed by the FDA.This approach has been used in traditional software development as well and subdivides the development process into feasibility, capability, effectiveness, and durability phases as we recommend above.
These five strategies collectively can help overcome the gaps we have identified on the road to effective regulation and will help increase trust and engagement on the part of government regulators, medical societies, and developers alike. Taken together, these steps have the potential to build a degree of trust that is lacking at present.
We anticipate the growth of these systems will drive the emergence of a substantial body of research rivaling the literature about algorithm development itself. Given the dramatic acceleration in diagnostic activity that SaMD applications will enable once automated diagnoses can be made at scale, the urgency in this branch of research is only likely to increase pressure on regulators over time.
We are only now at the beginning stages of seeing how AI can enhance the quality of human life and health. Ensuring that diagnostic algorithms perform effectively both in controlled environments and in real-world settings will ensure safety and transparency of and trust in these applications, facilitating broad adoption in this technology that could improve health outcomes for millions, not just in the United States, but around the world. We expect regulatory frameworks will continue to evolve and become more comprehensive, more anticipatory, and more inclusive of imaging professionals and quality and safety experts.
David Larson is a professor of radiology at Stanford Medical Center where he also serves as the vice chair for education and clinical operations.
Daniel L. Rubin is a professor of biomedical data science and of radiology, director of biomedical informatics for the Stanford Cancer Institute, and faculty affiliate of Stanford’s Institute for Human-Centered Artificial Intelligence.
Curtis Langlotz is a professor of radiology, chair of the Health Policy Committee of Stanford’s Institute for Human-Centered Artificial Intelligence (HAI), and director of Stanford’s Center for Artificial Intelligence in Medicine and Imaging.
Commentary
Building better diagnostic standards for medical AI
September 30, 2021