Last weekend, Tori Saylor, Michigan Governor Gretchen Whitmer’s deputy digital director, watched as President Donald Trump used yet another rally to attack her boss. She knew what would come next: “I see everything that is said about and to her online. Every single time the President does this at a rally, the violent rhetoric towards her immediately escalates on social media. It has to stop. It just has to,” Saylor tweeted.
Saylor was describing a dynamic that has now become familiar to researchers of online speech: Offensive speech on the internet tends to arise in response to political events on the ground. After Trump has attacked his opponents at a rally or other event, his online followers have, in some cases, taken that as a cue to attack those same opponents. For the president, it provides a useful amplifying tool. For the opponents being targeted, it represents a nightmare of online harassment.
But what about Trump’s online speech? Just as he targets his opponents in rallies and speeches, he also takes to Twitter to dole out criticism and ad hominem attacks. Here, we examine three recent tweets from the president and whether his tweets have a similarly negative impact on the quality of other online speech. These three tweets offer a case study in how elite speech online can impact the incidence of harmful speech. The tweets in question are not obviously threatening in nature—they fall into a well-documented trend of Trump attacking politicians on Twitter while remaining in-bounds of platforms’ content moderation policies. But that does not mean that they do not impact the overall quality of online discourse. Our findings highlight the challenges platforms face as they define their content moderation guidelines and systems in the lead-up to, and aftermath of, the election.
Consider the following presidential tweets:
…today that they foiled a dangerous plot against the Governor of Michigan. Rather than say thank you, she calls me a White Supremacist—while Biden and Democrats refuse to condemn Antifa, Anarchists, Looters and Mobs that burn down Democrat run cities…
— Donald J. Trump (@realDonaldTrump) October 9, 2020
…I’m playing for your guns, and I’m playing for your values. For all the Federal Employees in Virginia, remember, it was me that got you the Federal Pay Raises, not Sleepy Joe Biden. I’ll be having a Big Rally in Virginia, to be announced soon! https://t.co/WwzdPhDkAZ
— Donald J. Trump (@realDonaldTrump) September 18, 2020
So weird to watch @FoxNews interviewing only failed Dems, like Represenative Tim Ryan of Ohio, who got zero percent in his recent presidential run, and following Sleepy Joe’s train to wherever. What a difference from the past – But we will win anyway!!!
— Donald J. Trump (@realDonaldTrump) September 30, 2020
Collecting and classifying tweets
For each of the three politicians mentioned in Trump’s tweets, we complete a three-step analysis on a random sample of tweets. (Everyday, the NYU Center for Social Media and Politics collects a 10% random sample of Twitter, which amounts to tens of millions of daily tweets.) First, we use keywords to identify the corpus of tweets associated with each of the three politicians Trump mentioned. For each politician, we used the politician’s name, Twitter handle, and title. To be included in the corpus for Virginia Gov. Ralph Northam, for example, a tweet had to include “Governor of Virginia,” “Governor of VA,” “@GovernorVA,” “Northam,” or “Ralph Northam.” Next, we estimate the levels of two characteristics in that corpus: severe toxicity and threats. We do so by using Perspective, an open source API created by Jigsaw and Google’s Counter Abuse Technology team to enable the classification of harmful speech online. Perspective defines severe toxicity as “very hateful, aggressive, disrespectful” speech, and threats as describing “an intention to inflict pain, injury, or violence against an individual or group.” Third, we construct an interrupted time series with a 24-hour moving average, which enables us to isolate the impact of Trump’s tweet on the levels of severe toxicity and threats. Taken together, this analysis provides a birds-eye view of how Trump’s recent tweets impact the larger online discourse around the political figures he attacked.
It should be noted that identifying types of problematic speech online poses significant definitional and measurement challenges for governments, platforms, and researchers. Here, we use a method that that relies on pre-trained data and an open API, enabling relatively quick and low-cost analysis.
Follow the leader
In the immediate aftermath of Trump’s tweets, levels of severe toxicity and threats increased in all three cases. Notably, the largest increase in both categories is found in the tweets about Northam. This is perhaps unsurprising, as Trump’s tweet attacking Governor Northam seems the most extreme. However, after the sudden spike in toxicity and threats in the three corpora, the levels of both forms of harmful speech return to near pre-tweet baselines.
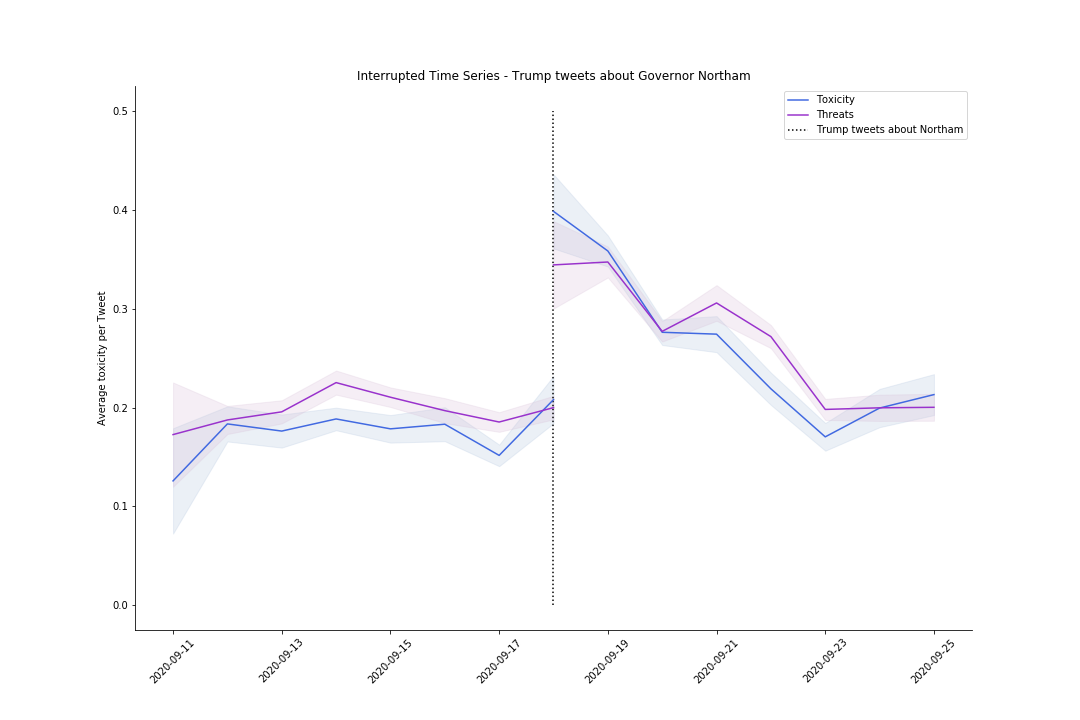
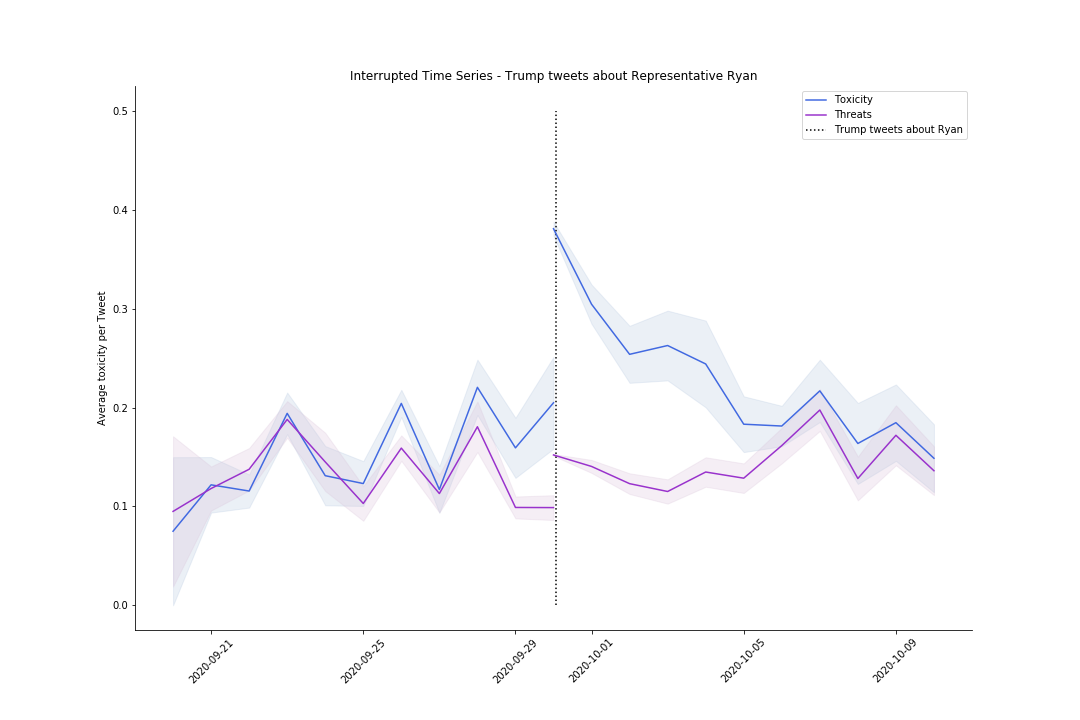
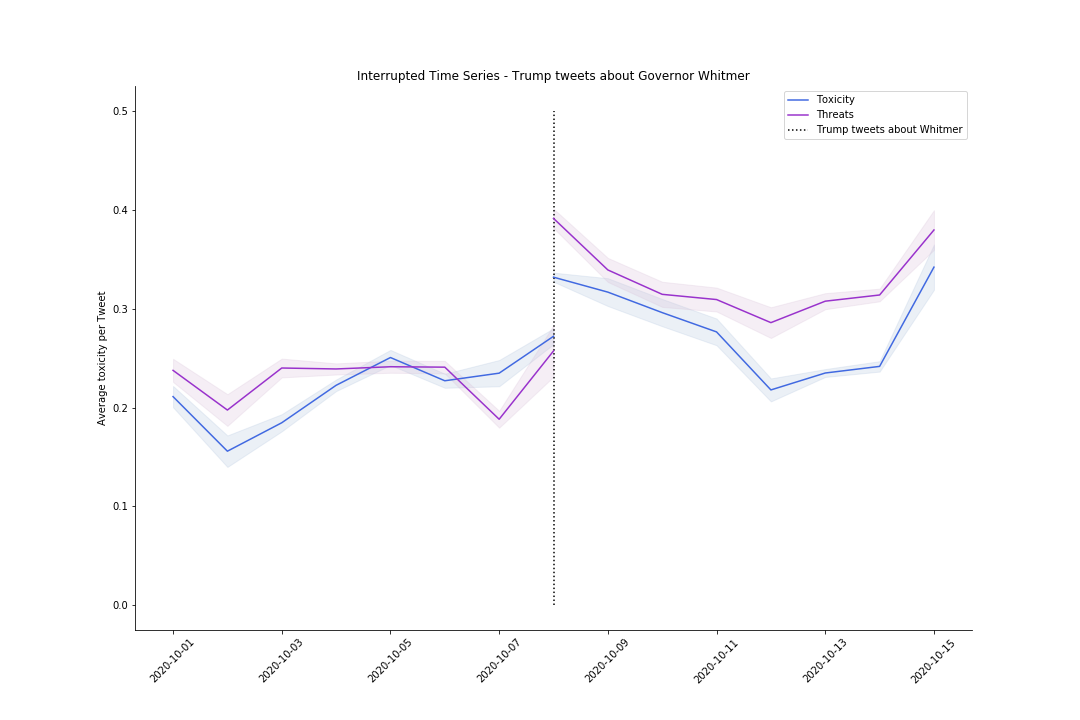
The notable exception during the period of interest is the case of Whitmer, where tweets mentioning her containing threatening and toxic language rise after Trump’s tweet, dip in the following days, only to increase again. This second increase could be due to heightened attention on Whitmer after the FBI foiled a kidnapping plot targeting her. While threats and toxic language generally decreased after the initial burst, more scholarship is needed to better understand the long-term impacts of targeted harassment towards politicians after they are attacked on Twitter.
What we see here closely aligns with recent research on Twitter, which has provided evidence for “bursty” patterns in which hate speech spikes in the aftermath of an event (either real world or online), before returning to a relatively low baseline. That research found that anti-Semitic speech on the platform spiked after Trump retweeted anti-Semitic content, then dropped to pre-tweet levels. The graphs documenting these bursts of hate speech, which include data from June 17, 2015 through June 15, 2017, look like readings from a seismometer—eruptions of hate speech on Twitter immediately after an event, followed by a return to a low steady state, with no systematic trend upwards over the period in question.
What this means for content moderation
The bursty pattern presents difficulties for content moderation policies and enforcement. First, this pattern creates a whack-a-mole dynamic in which harmful speech targeting a figure may appear in bursts, only to recede. (To be sure, there are important examples in which individuals or groups remain the targets of harmful speech over extended time periods.) And while Twitter estimates that 51% of tweets that violate the platform’s terms of service are automatically caught by its machine learning systems, this leaves 49% to be detected by human content moderators. Given the scale of the platform, this can amount to thousands if not millions of unflagged harmful tweets targeting an individual or group. Second, research suggests that women and people of color who are public figures are especially likely to be targeted by harmful speech. So while levels of harmful speech might return to an equilibrium after an initial spike, this does not undo the harm experienced by the recipients of the toxic and threatening speech, as well as others who were exposed to it.
Recent research has emphasized the importance of political elites in shaping online discussion, such as Trump’s central role in pushing false narratives around Antifa and voting by mail. As shown here, Trump’s tweets attacking politicians, while not especially noxious by online standards, can lead to a sudden increase in toxic and threatening speech. This builds on a larger literature that emphasizes the role of elites in setting discursive norms, especially around harmful language. Out of concern that citizens need to be able to examine the public statements of political and other public officials, Twitter and other social media platforms have generally committed to protecting elite speech. But when elite speech that is itself not acutely harmful causes a rise in harmful speech, that presents a major challenge for content moderation teams concerned about maintaining civility on their platforms.
While Trump poses especially difficult challenges for platform content moderation, he is far from alone in inspiring harmful speech among his followers. The norms around online political speech that Trump has helped establish are likely to stay with us for the foreseeable future, as will questions about how to best balance the public interest in not censoring elite speech while promoting healthier discourse online.
Megan Brown is a Research Scientist at the NYU Center for Social Media and Politics.
Zeve Sanderson is the Executive Director of the NYU Center for Social Media and Politics.
Google and Twitter provide financial support to the Brookings Institution, a nonprofit organization devoted to rigorous, independent, in-depth public policy research.
The Brookings Institution is committed to quality, independence, and impact.
We are supported by a diverse array of funders. In line with our values and policies, each Brookings publication represents the sole views of its author(s).
Commentary
How Trump impacts harmful Twitter speech: A case study in three tweets
October 22, 2020