At the heart of the health-care, decisionmaking, and supply-chain problems exposed by SARS-CoV-2 (COVID-19) is an unquenchable thirst for data—data to inform health professionals caring for COVID-19 patients and data to guide the decisions of policymakers and the public. Such data is available and used today in dashboards, surveillance systems, disease forecasting models, and at the point of care. However, anyone who works with or relies upon it would acknowledge that the methods for collecting, sharing, and transforming that data into actionable information are incomplete, fragile, lack standardization, and are vulnerable to cyber threats and disinformation. In many cases, such data is late, incomplete, and error-prone.
We—the health community—must strengthen our ability to leverage data in managing the health of individuals and populations. We have to do this in order to predict, prevent, detect, and respond to health threats and achieve global health security. We needed this ability before the pandemic; it became imperative during the pandemic; and we assuredly need it after this pandemic—to better protect lives during the next one. The health industry must apply systems engineering principles and best practices to the development of a comprehensive data ecosystem—a holistic, requirements-driven, risk-based approach in contrast to today’s reductionist and siloed approach to health data. The goal is to establish a health-data ecosystem with security and privacy requirements designed in from the start that continuously and efficiently collects and distributes timely, accurate, and comprehensive data among interdependent entities spanning all levels of society, leaving the world better prepared to tackle the next health crisis.
Preparing for the next pandemic
Industries that have struggled with but ultimately overcome complex challenges using the principles of systems engineering offer a roadmap for the health industry to build a health-data ecosystem. Complex, high-risk industries, such as aviation, automobile manufacturing, and the financial sector have employed a requirements-driven systems engineering approach to create robust, resilient, and safe systems that constantly learn and refine themselves. The aviation sector, for example, relies on an interconnected set of systems and subsystems engineered to efficiently and safely provide services. While aviation is not free from inefficiencies or safety challenges, the sector has relied on systems engineering principles to integrate its disparate components and has matured remarkably since powered flight became possible.
The power of systems engineering principles stems from using interdisciplinary methods to integrate and manage interrelated subsystems across their life cycles so that these subsystems work in concert for a shared purpose. These interdisciplinary methods—which include areas such as requirements management, modeling and simulation, risk management, verification and validation plus a range of specialty fields that include human factors, test and evaluation, reliability and resilience engineering, and sustainability—drive systems engineers to conceive of solutions from a holistic, rather than reductionist perspective, to meet the needs of a diverse set of stakeholders.
The creation a of a high-performing data ecosystem built on systems engineering principles entails collecting and using data at the individual, population, and global levels. The term ecosystem is used to emphasize the analogy to ecosystems of the natural world, where components are interconnected and interdependent. A health-data ecosystem is founded on models, analytics, and visualizations to fuel data-driven decisions. A data ecosystem is a collection of disparate fit-for-purpose systems that capture, analyze, and, where appropriate, distribute data and information across the ecosystem’s constituent systems. Network interconnections ensure that data and information are accessible to decisionmakers and stakeholders at all levels. By connecting disparate systems through this ecosystem, stakeholders benefit from the emergence of capabilities that would otherwise not exist if these constituent disparate systems were left as siloed entities. The ecosystem is not something that just “gets switched on” at a time of crisis; rather, it is always operational, surveilling for the next pandemic and managing data associated with other medical conditions that have global ramifications, such as cancer, diabetes, and cardiovascular disease.
What might such an ecosystem look like when deployed? Two current examples of tools that would inform it are the Johns Hopkins Precision Medicine Analytics Platform (PMAP) and the COVID-19 Dashboard by the JHU Coronavirus Research Center, for which we at the Applied Physics Laboratory created the data pipeline. The health industry lacks robust data in nominal, steady-state situations, a problem the COVID-19 pandemic has only exacerbated. Using analytic tools in its absence results in outputs that are rightly critiqued because of a lack of high confidence in metrics, timeliness, and trustworthiness, but the establishment of a health-data ecosystem would put tools in the hands of policymakers and scientists to gain insight.
Fundamentally, this health-data ecosystem would serve as the basis for a robust, resilient system that continuously allows stakeholders to operate at the highest levels of efficiency and safety. Similarly, this infrastructure would provide the input forecasting and prediction models need to enable decisions in the real world, allowing us to move away from research-focused models that have failed to adapt to operational realities. At the institutional level, the ecosystem would enable a wide variety of health sector stakeholders to effectively and efficiently manage care, safety, and quality.
The health industry is a complex socioeconomic-technical enterprise with numerous disparate and intertwined entities, which is why it has been particularly difficult to institute the types of structural changes that benefit other sectors. Achieving better health outcomes from more efficient care using a health-data ecosystem is going to require concerted and collaborative efforts from a wide range of public and private entities. A requirements-driven systems engineering approach that couples systems thinking and the systems engineering life cycle is required to marshal this effort’s focus and energy.
The key first step in this approach is the designation of a lead organization to shepherd its design. An ideal lead organization would be a global non-profit, or coalition of global non-profits, that has rich, diverse, and far-reaching relationships with crucial governments, international organizations and technical partners. That lead organization could then leverage these relationships to build the necessary partnerships within and across nations. Additional key steps include identifying and prioritizing which aspects of the health-data ecosystem to address initially and which to address subsequently. Key challenges will include resource limitations, policy constraints, loss of focus, and integration with legacy capabilities. These challenges will be formidable; however, following systems engineering principles and best practices offers a proven pathway for minimizing risk, while producing a system that meets end-user needs and ultimately improves health and safety.
Mapping the health-data ecology
Individual. The comprehensive collection of physiological, biological, and environmental data begins at the individual level but must be coupled with social data to understand the risk and status of acute and chronic conditions. This information can be used by an individual to optimize her health, used by clinicians to improve individual care, aggregated and de-identified by public-health professionals to manage the health of populations, and combined with mobility data to understand the individual’s interactions within a defined population. Increasingly, people are wearing sensors to track their sleep, heart rates, activity, and other signals related to their health. As these devices improve and become more ubiquitous, we have the opportunity to collect, integrate, and analyze these disparate and unique data sources to create new knowledge that can be leveraged for the betterment of the health of all. Patient privacy must be protected, and it is envisioned that individual data would only be provided up to a level at which identification is necessary for a meaningful response or intervention, after which it would be de-identified and aggregated at a population level.
Local. The health of an individual relates to the health of local clusters of individuals. COVID-19 has reinforced the critical nature of this relationship. Understanding the interactions and interdependencies of the individual within the context of these subpopulations enables data-driven decisionmaking regarding resource utilization, risk-mitigation efforts, and interventions. Additionally, the ecosystem would allow institutions such as nursing homes, schools, and hospitals to define and refine data collection and utilization processes for routine operations before a crisis unfolds.
Individual data, whether personally derived or gathered in a clinical setting, is but one type of data that feeds into the larger picture. Other feeds could be related to equipment and supplies, staffing, infrastructure, or finances. During the current crisis, the lack of comprehensive knowledge yielded uncertainty in how to best respond, particularly with regard to closing/reopening schools and businesses, managing hospital capacity, patient care protocols, and distributing resources such as personal protective equipment.
At the local level, new and informative data streams such as socioeconomic information, local non-pharmaceutical interventions, and mobility data should be incorporated into the ecosystem. Such data would enable spatiotemporal analyses of health trends, situational awareness, and resiliency. The health-data ecosystem would allow data to flow both down to the individual level and up to higher levels to inform responses. It would establish a means for effective data collection and knowledge generation at the local level. This reduces ambiguity and increases the probability of identifying “hot spots,” giving agencies the ability to make timely, flexible, and appropriate decisions, such as limiting gathering sizes.
Regional. As in the 1918 influenza pandemic, COVID-19 initially spread rapidly in certain parts of the United States, such as Seattle and New York, and subsequently elsewhere. Not all cities, states, or regions were impacted to the same degree at the same time. Such latency should afford time for knowledge to grow, leading to proactive responses that will lessen the impact as time goes on. Deploying a health-data ecosystem would enable regional decisionmakers to share and leverage data and knowledge gained from actions taken in real-time to identify vulnerable and at-risk populations, establish policies to protect public health, implement regional travel and commerce solutions, and identify areas most in need of health care resources.
National. The national level is far removed from the individual level, but it is informed by the largest possible amount of individual data. This separation makes it even more important to have a health-data ecosystem that supports seamless awareness across all levels. Data-informed national policy can have sweeping and widespread implications, leading to positive outcomes, whereas blind spots, ambiguity, and misinformation can erode effective outcomes and undermine the ability to adapt to rapidly evolving situations.
Global. In today’s highly interconnected world, cooperation and the sharing of data and knowledge across nations is critically important to halt the cascading effects of global pandemics. When effective, timely, and comprehensive data are collected at the national level, that information can be shared at the global level. Information shared at the global level can then inform approaches back at the national level to ensure supply chains, economies, public-health strategies, policies, and research are resilient and optimized.
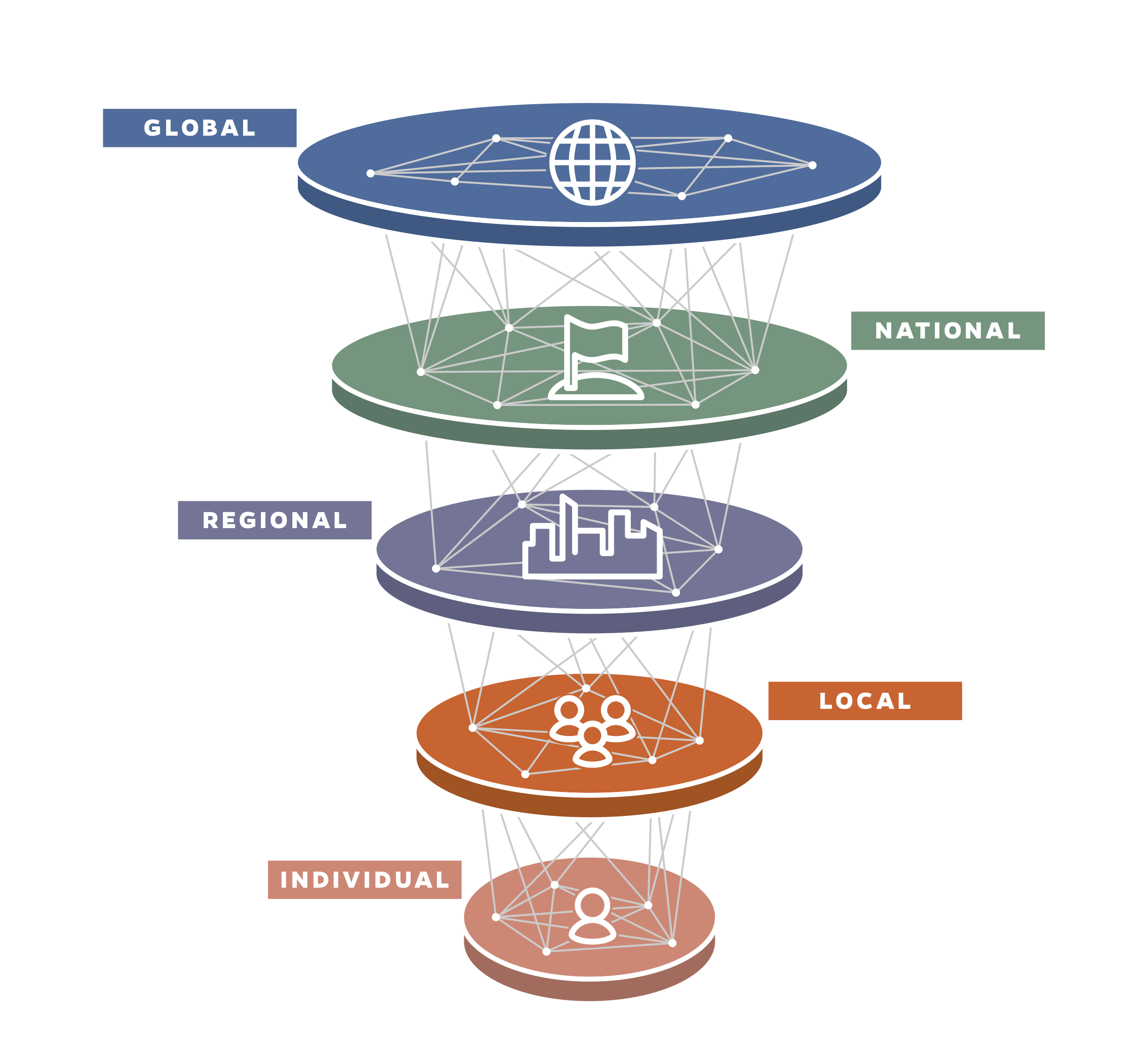
Technical approach
Once a robust data ecosystem is in place, technologies and analytic approaches can be overlaid to effectively utilize the data and drive decisions. A comprehensive data ecosystem must support many users who produce, consume, and analyze disparate data in different ways and want to answer unique questions with myriad analytics and visualizations. A modular, horizontally layered architecture would serve as generic model for such an ecosystem, and there are numerous real-world examples of this kind of architecture.
Health-related examples include those mentioned earlier: the Johns Hopkins Precision Medicine Analytics Platform (PMAP) and the JHU COVID-19 Dashboard. PMAP supports researchers and clinicians in realizing the potential power of precision medicine to improve the health of individuals. The platform does this by providing an analytic framework that enables grouping like-individuals into subpopulations, thereby providing insight into an individual’s likely health trajectory and possible interventions. Whereas PMAP operates at the individual and population levels of the health-data ecosystem, the COVID-19 Dashboard spans several levels by tapping into data sources from around the world to report on global, regional, and local disease burdens. Common key attributes of these real-world examples of horizontal architectures include access to disparate data sources; a focus on engineering robust data curation layers and models; analytics and visualization at scale; security and privacy controls and data provenance to ensure trust; models designed to support real-world operational decisions; and modularity to enable replacement of legacy capabilities with rapidly integrated advanced capabilities.
The health-data ecosystem should strive to achieve the full data science maturity, moving beyond retrospective and toward prospective analyses. How do we get there? In the field of data science, analytics can be described as descriptive, predictive, and prescriptive. Descriptive analytics involve understanding the data and can include statistical measures or characteristics such as completeness and timeliness. Moving from retrospective to prospective analyses can be achieved with predictive analytics. Predictive analytics use past data to make future predictions. In the current COVID-19 pandemic, the data science community is focused on predictive analytics but continues to struggle with the need for additional data to improve these models. Prescriptive analytics, the third type, move beyond predictive to address human intervention and provide decision options. Our vision for a health-data ecosystem is to reach a maturity that enables prescriptive analytics for all entities at all levels.
To ensure data integrity and confidentiality, the ecosystem would incorporate privacy and security requirements from the earliest phases of design. The originator of data would authorize the ways in which it is shared. Individual data would be provided only when needed and anonymized and aggregated when possible. By satisfying privacy and security requirements, networks of interconnected systems could safely transfer data and information between the different components and levels of the ecosystem.
The COVID-19 pandemic subjected society to extraordinary challenges. The issues experienced in one sector of society had a profound impact on all others because of the interconnected and interdependent nature of today’s world. There is, however, hope for the near term and future. Successes in non-health-care fields highlight the value of systems engineering, and anecdotal examples of the application of systems engineering to health care serve as proof-of-principle models for scaling beyond one-off solutions. Our future responses to COVID-19-like crises, as well as our ability to provide safe and efficient care that leads to consistent positive outcomes for all individuals, depends on a new approach using systems engineering to develop a robust health-data ecosystem.
Sheri Lewis is a public health and disease surveillance expert and the Johns Hopkins Applied Physics Laboratory’s (APL) deputy mission area executive for national health. She oversees the APL JHU COVID-19 Dashboard team, as well as other data and technology programs to improve health systems integration, U.S. global health engagement and emergent threat response.
Alan Ravitz is the chief engineer in APL’s National Health Mission Area with more than 30 years of experience in systems engineering, design, field testing, and analysis that extends across biomedical and health care systems. He is also the chair of the JHU Whiting School of Engineering’s Engineering for Professionals – Healthcare Systems Engineering program.
Aaron Katz leads the Large Scale Analytic Systems Group at APL, working primarily in the areas of software engineering, data mining, machine learning, and public health informatics.
John Piorkowski is the chief artificial intelligence architect in APL’s Asymmetric Operations Sector, providing technical oversight and technical staff management for national security efforts including in cyber security and public health.
Commentary
A health-data ecosystem to protect against public-health threats
May 6, 2021