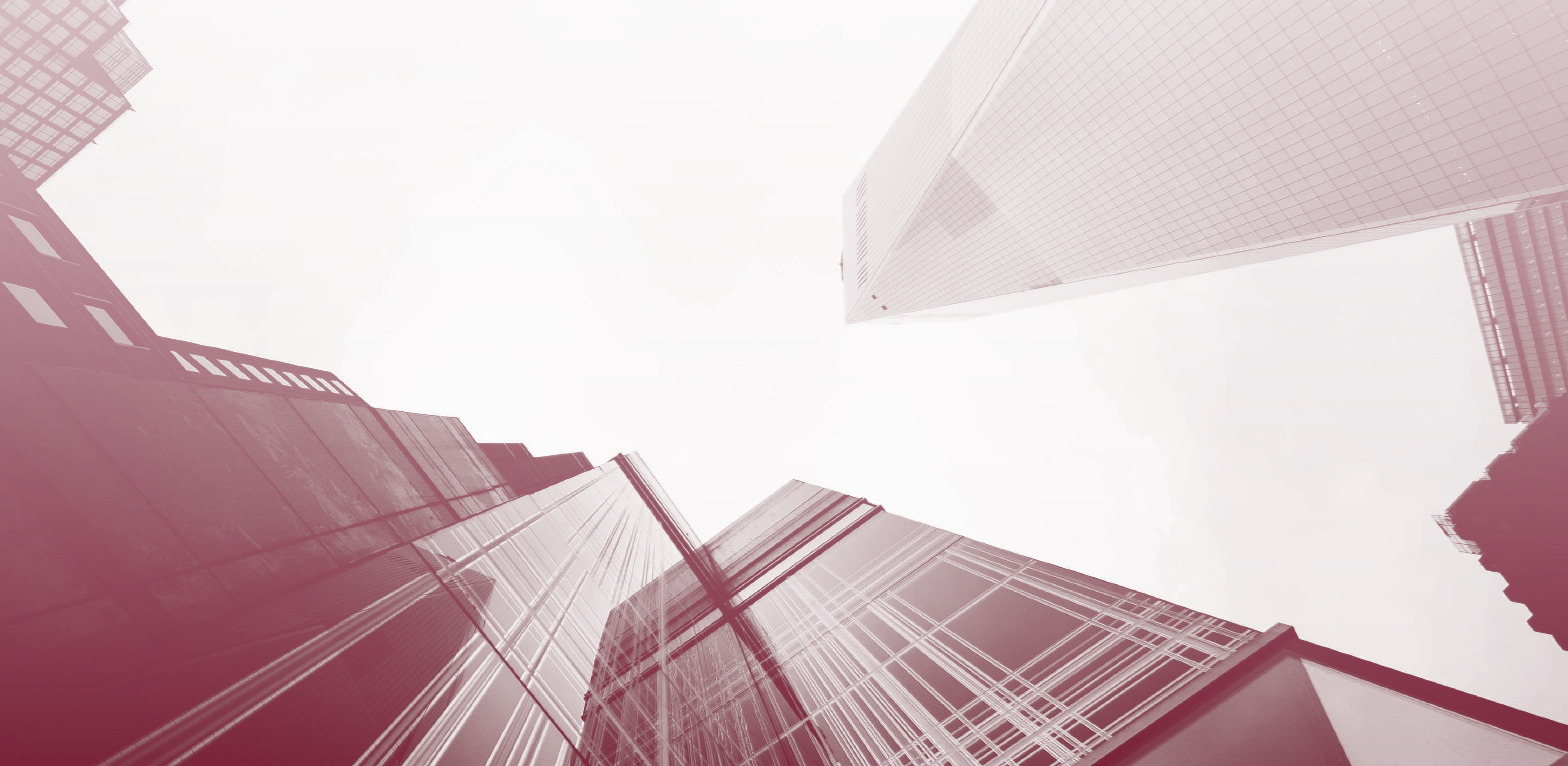
CHAPTER 6
DATA FLOWS AND CRITICAL TECHNOLOGIES
Optimizing North American supply chains in critical technologies: The USMCA digital advantage
Major developments in geopolitical relations since the updating of the North American Free Trade Agreement (NAFTA) through the United States- Mexico-Canada Agreement (USMCA) have put the regional agreement in a new context, magnifying the significance of its new digital trade chapter.
Briefly, the continuing expansion in the scope and quantity of data being captured with the ongoing digital transformation, the steep increase in the power of digital technologies such as artificial intelligence (AI) and machine learning (ML), and the convergence and integration of technologies across use cases (Park, 2019; Wheeler and Simpson, 2022) create new and pervasive governance challenges. Given this, governments worldwide are developing comprehensive and expansive regulatory frameworks—with limited real world experience and the absence of internationally agreed standards—in an area that remains under-theorized and under-developed in practical terms, particularly in regards to national security (see, e.g., Heath, 2021) and personal privacy (Acquisti et al., 2016). This creates uncertainty about the future scope of measures that might impinge on the free flow of data across borders and require some degree of data localization.
This uncertainty is particularly acute when it comes to the nexus of big data, machine learning, and AI. This area is critical to national security given the military advantages of dominating these new general-purpose technologies. It is also important to economic security given the prospects for capturing international economic rents and large social benefits that could potentially flow as these new technologies are deployed at scale bringing economies of scale to human capital-intensive services sectors (Ciuriak and Goff, 2022). Importantly, the scale and hyper-specialization of operations within the supply chain for these technologies is such that only a handful of global firms dominate particular niches, most of them outside of North America. This has sharpened sensitivity to supply chain risks given the current environment of intense geopolitical rivalry and concerted efforts to reduce risks and make supply chains more robust and secure.
The USMCA reforms play a particularly important role in this regard as the digital trade chapter establishes the basis for a strong and integrated information and technology space in North America by reducing regulatory uncertainty and investment risk. Analysis of the economic impact of the USMCA had already established that the reduction of uncertainty around data flows in the North American space represented the major source of economic gain from the reforms (USITC, 2019; Ciuriak 2020). The recent technological developments and the flaring of geopolitical rivalry give added— indeed transformative—significance to these aspects.
The altered technological context for the USMCA
The United States has long understood that technological leadership underpins its economic prosperity and security (see, e.g., Eaglen and Pollack, 2012; White House, 2017; 20). This understanding has been reflected in its trade agreements. Thus, the transition from the mature industrial economy to a knowledge-based economy (KBE) signalled by the steep upturn in the pace of patenting in the early 1980s was followed in short order by commitments to intellectual property (IP) standards in trade agreements (starting with the 1989 Canada-U.S. Free Trade Agreement and subsequently the 1995 World Trade Organization Agreement). Similarly, the transition to a data-driven economy (DDE) signalled by the steep upturn in the flow of data at the beginning of the 2010s was followed by the incorporation of data chapters in the 2016 Trans-Pacific Partnership Agreement and shortly thereafter in the USMCA.
The contest is clearly on, and U.S. trade policy has been swift to kick into gear—in this case, not through trade agreements but through trade sanctions that leverage U.S. control of a vast stock of technology assets that represents the substrate for the technologies of the MKC era. This has important implications for the USMCA impact.
These economic transitions, each of which can be traced to a set of related technological innovations (see Annex), changed the basis for international competitiveness based on dominance of the new forms of capital being created. In each case, the United States had first mover advantages and, in each case, early recognition and policy reaction enabled the United States to extend its global economic leadership even as its dominance in mature technologies was competed away.
Another major transition in technological conditions is now underway. Reflecting quantum leaps in the size and efficiency of specialized AI computer chips, orders of magnitude increases in the scale of the neural nets that train AI systems, and improvements in the training protocols (see Annex 1), AI systems are now routinely breaking through human benchmarks in knowledge-based tasks while demonstration projects such as ChatGPT are turning heads (and creating controversy). The palpable improvement in the quality of AI systems that these technological leaps have enabled is reflected in headlines that recognize 2022 as “The year AI became eerily human” (Verma, 2022).
The breakthroughs in powering up AI systems, however, are only the stage setters. Business innovations such as “Software as a Service” and “Platforms as a Service” have enabled AI development to be conducted on a massively parallel basis by literally hundreds of thousands of firms worldwide. As the myriad yet-unknown applications that are now being developed are deployed in the production of goods and services, they will form a growing stock of capital assets—“machine knowledge capital” or MKC—that will increasingly impact the international competitiveness of advanced economies built on the foundation of human knowledge capital.
This looming technological transition has geopolitical ramifications that will transform the context for the USMCA. China entered the KBE only around 2010, some 30 years behind the U.S., and, despite surging into the lead in international patent applications, still trails at a considerable distance in its international receipts for in-force IP.1 However, it entered the DDE more or less contemporaneously with the U.S. and leveraged the scale of its internal economy and rapid adoption of digital technologies to gain a sizeable lead on indicators such as e-commerce sales and mobile traffic data.2 As we enter the era of MKC, the United States enjoys a clear advantage in recognized AI startups (by a recent count, the U.S. has 13,398 AI startups to 1,936 in China according to Tracxn), but China’s Baidu reports some 180,000 firms as users of its AI development platform (Smith, 2022), which indicates considerable depth.
The contest is clearly on, and U.S. trade policy has been swift to kick into gear—in this case, not through trade agreements but through trade sanctions that leverage U.S. control of a vast stock of technology assets that represents the substrate for the technologies of the MKC era. This has important implications for the USMCA impact.
The USMCA digital advantage
The data intensity of the critical digital technologies
The AI sector, which includes computer services and digital platform services firms, is of course extremely data intensive. The same is true of the supporting economic infrastructure of advanced manufacturing, business services, and backbone infrastructure services. In fact, these sectors are close to twice as intensive in data flows as other economic sectors (Table 6).
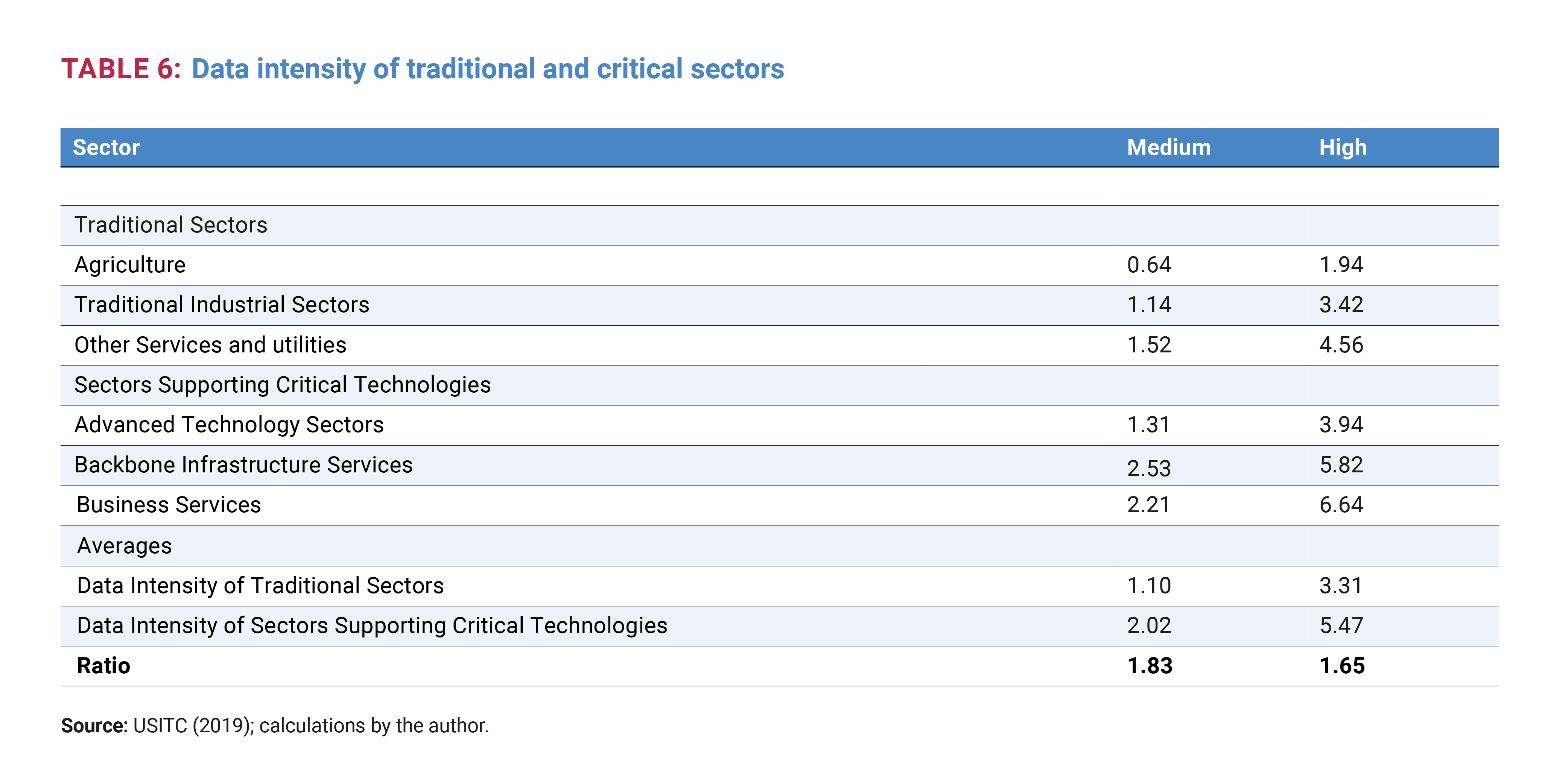
The USITC attributes a significant and positive impact on U.S. industries from the USMCA’s digital trade chapter, which requires the parties to ensure cross-border movement of data and prohibits restrictive data localization measures in the future, protects proprietary source codes and algorithms, and provides intermediary liability protection. These positive impacts derive entirely from a reduction of uncertainty about future regulatory interventions—in effect, the USMCA represents an insurance policy for industry that data flows within the North American economic space will continue to be unrestricted. This leads to the question of how valuable this insurance policy is.
Uncertainty as a preferential non-tariff measure
There is considerable evidence that uncertainty inhibits trade and investment (see e.g., Bloom et al., 2007; Handley and Limão, 2015; Novy and Taylor, 2020). This reality was underscored by recent “natural experiments”: the extended period of trade policy uncertainty created by the Brexit referendum, which impacted significantly on firms’ entry into cross-channel trade (Graziano et al. 2018, Crowley et al. 2018, and Bloom et al., 2019); and the renegotiation of the NAFTA (see e.g., Baker et al., 2019; Crowley, 2019; Jacks and Novy 2019), when trade policy uncertainty reverberated through supply chains (Blanchard, 2019) and reduced trade and investment (Fritz and Evenett, 2019; Jacks and Novy, 2019).
In the context in which the USMCA was negotiated, it was not initially clear how much benefit would come from the measures aimed at reducing data uncertainty. This was due to the fact that Canada and Mexico had already signed onto comparable measures in the Comprehensive and Progressive Partnership for Trans-Pacific Trade and commitments on regulatory frameworks tend to be applied multilaterally (Ciuriak, 2020). Arguably, the main impact of the USMCA measures was thus on the U.S. itself, which was the world’s leading proponent for unrestricted data flows. However, in the new multipolar context, in which the free flow of data is conditioned by trust, and in which the U.S. has powerful motivations to control the flow of certain data and technology, vis-à-vis China in particular, the USMCA takes on much greater significance and arguably makes North America a favored investment location.
Nowhere is policy uncertainty more acute than around digital technology where virtually every area of international interaction is under review (see, e.g., Kerry et al., 2023). Yet, this uncertainty for investors is not uniformly distributed.
Investment will clearly continue to flow to where the development talent is located but will also be influenced by considerations of diversification of sourcing, geopolitical risk, and regulatory risk. Clearly the risks of making investments in China for technology aimed at global markets have risen enormously, with spillover effects for countries more dependent on China economically. This includes Northeast Asia, which is geopolitically aligned with the U.S. but increasingly economically dependent on trade with China. This also includes Southeast Asia, which overtly balances this push and pull. India, which has absorptive capacity and has figured prominently in diversification discussions, will certainly attract supply chain diversification investment; however, its pursuit of strategic autonomy through an “internationalist” foreign policy that at times aligns with the West but at other times with the BRICS vision of multipolarity (Upadhyay, 2022) add to the various challenges of doing business that have historically limited inward flows of investment. In Europe, the combination of regulatory risk and the EU’s pursuit of its own version of strategic autonomy (Michel, 2020), which is partly manifested in its attempt to shape an independent policy towards China (see, e.g., Scholz, 2022; and von der Leyen, 2023, who described the approach to China as “de-risking” rather than “de-coupling”), will also inevitably influence investment decisions.
By comparison, policy uncertainty and its attendant costs for digital technology investment are much less in North America. In the first instance, this reflects the role that USMCA commitments play in creating a stable policy environment for data flows and digital technology investments as a result of the agreement’s digital trade chapter but also through other USMCA commitments, including on services, investment, telecommunications, and good regulatory practices, and its functioning dispute settlement mechanism. Secondly, the still-evolving U.S. response to geopolitical tensions with China (e.g., restrictions on U.S. nationals working in specified Chinese technology firms and export controls on a range of key technologies)—which create uncertainty for investments in China and related countries about ongoing access to U.S. technology assets due to national security concerns—do not apply to investments in North America.
Policy recommendations
In the context of the multipolar geopolitical landscape and its implications for trade in advanced technologies and the flow of data within the data-intensive innovation systems, the North American space promises to be the lowest risk zone for assured access to U.S. technology. Therefore, all else being equal, North America would become a prime destination for private investment in geopolitically sensitive sectors. The USMCA’s strong commitments on digital governance will support the development of an integrated North American digital realm, which will be essential for continued prosperity and security of the region.
There are several important policy recommendations for the future development of the USMCA. Insofar as North America becomes a low-risk investment destination for digital technologies and innovation poses geopolitical risks, the agreement could be enhanced in three ways.
First, explicit removal of Section 232 national security tariffs being applied to Canada and Mexico would enhance investment prospects for both countries and thus leverage the geopolitical risk premium.
The USMCA’s strong commitments on digital governance will support the development of an integrated North American digital realm, which will be essential for continued prosperity and security of the region.
Second, given the importance of governments as customers for new technologies, the attraction of supply chain investment into North America in the critical new technologies would benefit from the expansion of the North American procurement space. Buy American is a disadvantage in this context.
Third, the attraction of supply chain investment into North America in the critical new technologies space will inevitably require policies that attract important complementary assets, namely, skilled workers and clearly defined terms of engagement in research partnerships. Furthermore, North America needs a Sino- Pacific strategy in this regard that all three parties can buy into.
The context for the USMCA has changed since the agreement was negotiated, placing a new premium on the development of an integrated North American digital economy. This places the USMCA’s digital economy chapter in a new light and suggests the need for complementary policy reforms to enhance its impact in making North America a low-risk zone for critical new technologies.
John Maynard Keynes is known to have said, “When the facts change, I change my mind. What do you do, Sir?” The facts have indeed changed, and our policies and the narratives that build support for them need to change as well.
Annex: Technological transitions define new economic eras
This annex describes the historical transitions from the mature industrial era to a knowledge-based economy (KBE) and then to a data-driven economy (DDE) and identifies the technological breakthroughs that triggered these transitions. It then sketches out the technological developments that augur a new era of machine knowledge capital (MKC), and the implications for U.S.-China competition (for elaborations of these transitions see Ciuriak, 2022).
Knowledge-based economy (1980-2010)
- Key technological developments:
- Awareness of importance of innovation and intellectual property for the U.S. economy signaled by Bayh-Dole (1980).
- IBM personal computer (1981) enables widespread application of computer, especially when coupled with CAD-CAM PC software released by John Walker’s Autodesk (1982).
- Key outcome: These innovations enabled the industrialization of R&D, accelerating the pace of innovation.
- Key indicator: The steep upturn in the pace of U.S. and Patent Cooperation Treaty patenting in the early 1980s.
- U.S.-China competition: China entered the knowledge-based era around 2010 some 30 years behind the U.S. through a concerted push to upgrade its innovation system, including explicit strategies targeting the acquisition of IP. Yet, despite impressive progress, China still trails the U.S. by a wide margin on international IP receipts. This reflects the depth of the established asset position of the U.S., which is also the base of its geoeconomic clout in terms of technology sanctions.
Data-driven economy (2010-the present)
- Key technological developments:
- Deep learning neural nets developed by Geoffrey Hinton (2006) (Kelly, 2014).
- Release of iPhone (2007) ushers in the mobile era with a steep increase in the amount of data flowing into the cloud (Molla, 2017).
- Application of GPUs to neural nets by Stanley Ng’s team at Stanford (2009) powers the performance of neural nets (Kelly, 2014).
- Key outcome: These innovations enabled the industrialization of learning, further accelerating the pace of innovation.
- Key indicator: The steep upturn in the amount of data captured at the beginning of the 2010.
- U.S.-China competition: China entered the DDE contemporaneously with the U.S. and, despite the U.S. having a lead out of the gate, has lapped it:
- 52 percent global share of e-commerce vs. U.S.’s 19 percent by one estimate.
- Shopify estimates China’s share at 46.3 percent in 2022 ($2.8 trillion) compared to U.S. 14 percent share ($905 billion).
- China’s data scale advantage is huge: Ericsson reports China mobile data traffic = 26 exabytes/month compared to 6 in North America.
- Huawei’s 5G advance triggered U.S. reaction in 2018 that started the tech war proper.
Machine knowledge capital (the present and forward)
- Key technological developments:
- Specialized AI computer chip development has witnessed a “Cambrian explosion” in scale and number of alternative designs (Knight, 2021).
- Cerebras Systems announced its Wafer Scale Engine (WSE) in 2019, accelerating the training speed of neural nets by orders of magnitude (Moore, 2020); in 2021, it announced WSE-2, which features 2.6 trillion transistors and effectively doubled every metric in WSE-1 (Moore, 2021).
- In February 2020, Microsoft announced a 17 billion parameter language model, claimed to be the largest of its kind (Rosset, 2020); in May 2020, OpenAI published a paper on its 175 billion parameter GPT-3 model (Heaven, 2020); in January 2021, Google announced it had trained a 1.6 trillion parameter large language model (Wiggers, 2021).
- Months after Alibaba announced its M6 model with 10 billion parameters, it announced the expansion of M6 to 1 trillion and then to 10 trillion parameters while cutting energy requirements to 1 percent of the energy required by OpenAI’s 175 billion parameter GPT-3 (Houweling, 2021; Romero, 2021).
- In 2022, a Chinese team developed a system, BaGuaLu, which it claims can train a 14.5 trillion parameter system and has the capability to train a 174 trillion parameter “brain scale” model (Ma et al., 2022; Wang, 2022).
- Power requirements to run AI chips are being reduced dramatically through a variety of approaches and design innovations (e.g., Moore, 2020; Dhar, 2022, Hampson, 2022; Yirka, 2022; Romero, 2021).
- Meanwhile, the power of AI systems is being increased by orders of magnitude. For example, Amazon’s 20 billion parameter Alexa Teacher Model, announced in August 2022, is claimed to have matched benchmark performance of systems with hundreds of billions of parameters (Soltan et al., 2022) with its “sequence-to-sequence” training methodology. Similarly, Microsoft’s DeepSpeed system announced in January 2022 reported improvements to the “Mixture of Experts (MoE)” models that promise to reduce training costs by a factor of five (DeepSpeed Team et al., 2022).
- Key outcome: Reflecting the multiple dimensions along which gains are being made in terms of scale and efficiency of AI systems, training times are plunging (Moore, 2022). Specialized AI systems are now routinely breaking through human benchmarks (Stanford University, 2022). AI systems have now been awarded patents (Naidoo, 2021). AI-piloted fighter jets have out-dueled human-piloted jets in dogfights (Mizokami, 2020). The Beijing Winter Olympics showcased a suite of service robots deployed to address pandemic-related concerns (Ma, 2022). And OpenAI’s recently released ChatGPT (OpenAI, 2022) is turning heads with its ability to respond with plausible intelligence to questions in conversational mode.
- Key indicator: To be determined.
- U.S.-China competition: At the dawn of the era of MKC, U.S.-China competition is highly asymmetric. The U.S. has the world’s leading supporting environment for startups in general and in AI in particular: By a recent count, the U.S. has 13,398 AI startups to 1,936 in China— tracxn); and for scaling up: The U.S. has 470 Unicorns to 170 for China, which is, however, second best in the world. The U.S. controls key technologies in the development ecosystem and has moved to deny them to China. China, on the other hand, has unparalleled infrastructure for deployment of AI in productive capacities, especially in services, where China has major societal needs:
- In the past decade, China has filed 389,571 patents in the area of AI, or 74.7 percent of the world total WIPO.
- China has about 50 percent of the world total of installed industrial robots (44 percent growth in 2021—IFR) — this positions China to deploy smart robots into an existing robot-using production system.
- China has 2.2 million 5G base stations as of 2022, 60 percent of the world total– RCRWireless.
- DJI holds approximately 70 percent of the global drone market share—this positions China in the deployment of low-level autonomous devices.
- 180,000 companies are using Baidu AI development platform—presumably mostly Chinese.
- China displayed its ability to deploy service robot capabilities at the Beijing Winter Olympics.
Endnotes
- 1. In 2021, China’s international earnings on its stock of in-force IP assets amounted to less than US$ 12 billion in 2021 compared to US$ 124 for the United States (which is understated as it does not include the $14 billion in IP earnings allocated to Ireland, which mostly represent receipts by US firms that domicile their IP assets in Ireland for tax advantages). Source: World Bank Indicators, Charges for the use of intellectual property.
- 2. Ericsson (2022) reports China mobile data traffic at about 26 exabytes/ month in 2022 compared to 6.0 in North America.
References
Acquisti, Alessandro, Curtis Taylor, and Liad Wagman. 2016. “The Economics of Privacy,” Journal of Economic Literature 54(2): 442–492. http://dx.doi.org/10.1257/jel.54.2.442
Baker, Scott, Nicholas Bloom, and Steven Davis. 2019. “The extraordinary rise in trade policy uncertainty,” Vox. eu, 17 September. https://www.wita.org/wp-content/ uploads/2019/09/The-extraordinary-rise-in-trade-policy-uncertainty-_-VOX-CEPR-Policy-Portal.pdf
Blanchard, Emily 2019. “Trade Wars in the Global Value Chain,” in Meredith A. Crowley, “Trade War: The Clash of Economic Systems Threatening Global Prosperity,” VoxEU.org, 30 May. https://cepr.org/publications/books-and-reports/trade-war-clash-economic-systems-threatening-global-prosperity.
Bloom Nick, Stephen Bond, and John Van Reenen. 2007. “Uncertainty and Investment Dynamics.” Review of Economic Studies 74: 391-415.
Ciuriak, Dan. 2022. “The Data-Driven Economy Raises New Challenges for Global Governance,” Opinion, Centre for International Governance Innovation, 03 October 2022. cigionline
Ciuriak, Dan. 2020. “The Trade and Economic Impact of the USMCA: Making Sense of the Alternative Estimates,” C.D. Howe Institute Working Paper, 30 June. https://www.cdhowe.org/ public-policy-research/trade-and-economic-impact-cusma-making-sense-alternative-estimates.
Ciuriak, Dan and Patricia Goff. 2021. “Economic Security and the Changing Global Economy,” Centre for International Governance Innovation Series: Reimagining a Canadian National Security Strategy Report No. 8. 13 December. https:// www.cigionline.org/publications/economic-security-and-the-changing-global-economy/
Crowley, Meredith, Oliver Exton, and Lu Han. 2018. “Renegotiation of Trade Agreements and Firm Exporting Decisions: Evidence from the Impact of Brexit on UK Exports,” Working paper, Available at https://papers.ssrn.com/sol3/papers. cfm?abstract_id=3211689.
Crowley, Meredith A. 2019. “Trade War: The Clash of Economic Systems Threatening Global Prosperity,” VoxEU.org, 30 May. https://cepr. org/publications/books-and-reports/trade-war-clash-economic-systems-threatening-global-prosperity
DeepSpeed Team and Andrey Proskurin. 2022. “DeepSpeed: Advancing MoE inference and training to power next-generation AI scale,” Blog, Microsoft, 19 January. https://www.microsoft.com/en-us/ research/blog/deepspeed-advancing-moe-inference-and-training-to-power-next-generation-ai-scale/
Dhar, Payal. 2022. “New AI Chip Twice as Energy Efficient as Alternatives,” IEEE Spectrum, 29 August. https://spectrum.ieee.org/ai-chip
Ericsson. 2022. “Ericsson Mobility Report,” November. https://www. ericsson.com/en/reports-and-papers/mobility-report/reports/ november-2022
Fritz, Johannes and Simon Evenett. 2019. “Misdirection and the trade war malediction of 2018: Scaling the US–China bilateral tariff hikes,” Vox EU, 1 July. https://cepr.org/voxeu/columns/misdirection-and-trade-war-malediction-2018-scaling-us-china-bilateral-tariff-hikes
Graziano, Alejandro, Kyle Handley, and Nuno Limão. 2018. “Brexit Uncertainty and Trade Disintegration,” NBER Working Paper 25334. http://www.nber.org/papers/w25334
Hampson, Michelle. 2022. “This AI Chip Hits New Ultralow Power Lows,” IEEE Spectrum, 15 June. https://spectrum.ieee.org/low-power-ai-spiking-neural-net
Handley, Kyle and Nuno Limão. 2015. “Trade and investment under policy uncertainty: Theory and firm evidence,” American Economic Journal: Economic Policy 7, 189-222.
Heath, J. Benton. 2021. “Making Sense of Security.” Working Paper https://ssrn.com/abstract=3949923.
Heaven, Will Douglas. 2020. “OpenAI’s new language generator GPT-3 is shockingly good—and completely mindless.” MIT Technology Review, 20 July https://ssrn.com/abstract=3949923.
Houweling, Elles. 2021. “Alibaba-backed institute achieves “another Sputnik moment” in China’s battle for AI supremacy,” Verdict, 9 November. https://www.verdict.co.uk/alibaba-backed-institute-china-battle-ai-supremacy/
Jacks, David and Dennis Novy. 2019, “Trade Wars May ‘Bloc Up’ World Trade,” in Meredith A. Crowley, “Trade War: The Clash of Economic Systems Threatening Global Prosperity,” VoxEU.org, 30 May. https://cepr.org/publications/books-and-reports/trade-war-clash-economic-systems-threatening-global-prosperity
Kelly, Kevin. 2014. “The Three Breakthroughs That Have Finally Unleashed AI on the World,” Wired, 27 October. https://www.wired. com/2014/10/future-of-artificial-intelligence/
Kerry, Cameron F., Joshua P. Meltzer, and Matt Sheehan. 2023. “Can democracies cooperate with China on AI research? Rebalancing AI research networks,” Brookings Institute, 9 January. https:// www.brookings.edu/research/can-democracies-cooperate-with-china-on-ai-research/
Knight, Will. 2021. “A New Chip Cluster Will Make Massive AI Models Possible,” Wired, 24 August. https://www.wired.com/story/ cerebras-chip-cluster-neural-networks-ai/
Ma, Jess. 2022. “Winter Olympics: the marvellous machines keeping the Games moving in the right direction.” South China Morning Post, 7 February https://www.scmp.com/sport/china/ article/3166079/winter-olympics-marvellous-machines-keeping-games-moving-right.
Michel, Charles. 2020. “Strategic autonomy for Europe —the aim of our generation.” Speech by European Council President Charles Michel to the Bruegel think tank.” European Council, September 28. www.consilium.europa.eu/en/press/press-releases/2020/09/28/l-autonomie-strategique-europeenne-est-l-objectif-de-notre-generation-discours-du-president-charles-michel-au-groupe-de-reflexion-bruegel/.
Molla, Rani. 2017. “How Apple’s iPhone changed the world: 10 years in 10 charts,” Vox, 26 June. https://www.vox. com/2017/6/26/15821652/iphone-apple-10-year-anniversary-launch-mobile-stats-smart-phone-steve-jobs
Moore, Samuel K. 2020. “Low-Power AI Startup Eta Compute Delivers First Commercial Chips,” IEEE Spectrum, 13 February. https://spectrum.ieee.org/lowpower-ai-startup-eta-compute-delivers-first-commercial-chips
Moore, Samuel K. 2021. “Cerebras’ New Monster AI Chip Adds 1.4 Trillion Transistors,” IEEE Spectrum, 20 April. https://spectrum.ieee.org/cerebras-giant-ai-chip-now-has-a-trillions-more-transistors
Moore, Samuel K. 2022. “We’re Training AI Twice as Fast This Year as Last,” IEEE Spectrum, 30 June. https://spectrum.ieee.org/mlperf-rankings-2022
Naidoo, Meshandren. 2021. “In a world first, South Africa grants patent to an artificial intelligence system, The Conversation, 5 August. https://theconversation.com/in-a-world-first-south-africa-grants-patent-to-an-artificial-intelligence-system-165623
Novy, Dennis and Alan M. Taylor. 2020. “Trade and Uncertainty,” The Review of Economics and Statistics 102(4): 749–765. Doi: https:// doi.org/10.1162/rest_a_00885
Mizokami, Kyle. 2020. “AI vs. Human Fighter Pilot: Here’s Who Won the Epic Dogfight,” Popular Mechanics, 25 August. https://www. popularmechanics.com/military/aviation/a33765952/ai-vs-human-fighter-pilot-simulated-dogfight-results/
OpenAI. 2022. ChatGPT. https://chat.openai.com/chat
Park, Suzy E. 2019. “Technological Convergence: Regulatory, Digital Privacy, and Data Security Issues,” Congressional Research Service, Report to Congress R45746, 30 May. https://crsreports. congress.gov.
Romero, Alberto. 2021. “Meet M6 — 10 Trillion Parameters at 1% GPT- 3’s Energy Cost.” Towards Data Science, 10 November https:// towardsdatascience.com/meet-m6-10-trillion-parameters-at-1- gpt-3s-energy-cost-997092cbe5e8.
Rosset, Corby. 2020. “Turing-NLG: A 17-billion-parameter language model by Microsoft,” Blogpost, Microsoft, 13 February. https:// www.microsoft.com/en-us/research/blog/turing-nlg-a-17-billion-parameter-language-model-by-microsoft/
Scholz, Olaf. 2022. “We don’t want to decouple from China, but can’t be overreliant,” Politico, 3 November. https://www.politico.eu/article/ olaf-scholz-we-dont-want-to-decouple-from-china-but-cant-be-overreliant/
Smith, Craig S. 2022. “Baidu’s PaddlePaddle Spins AI up to Industrial Applications,” IEEE Spectrum, 04 August. https://spectrum.ieee.org/paddlepaddle-baidu
Soltan, Saleh, Shankar Ananthakrishnan, Jack FitzGerald, Rahul Gupta, Wael Hamza, Haidar Khan, Charith Peris, Stephen Rawls, Andy Rosenbaum, Anna Rumshisky, Chandana Satya Prakash, Mukund Sridhar, Fabian Triefenbach, Apurv Verma, Gokhan Tur, and Prem Natarajan. 2022. “AlexaTM 20B: Few-Shot Learning Using a Large- Scale Multilingual Seq2Seq Model,” arXiv:2208.01448, posted 2 August. https://arxiv.org/abs/2208.01448
Stanford University. 2022. “Artificial Intelligence Index Report 2022,” Stanford University Human-Centered Artificial Intelligence. https:// aiindex.stanford.edu/wp-content/uploads/2022/03/2022-AI-Index-Report_Master.pdf
Upadhyay, Shreya. 2022. “BRICS, Quad, and India’s Multi-Alignment Strategy,” South Asian Voices, 12 July. https://southasianvoices. org/brics-quad-and-indias-multi-alignment-strategy/
USITC. 2019. “U.S.-Mexico-Canada Trade Agreement: Likely Impact on the U.S. Economy and on Specific Industry Sectors,” Washington: United States International Trade Commission, Publication Number: 4889, Investigation Number: TPA 105-003, April 2019.
von der Leyen. 2023. “Special Address by President von der Leyen at the World Economic Forum,” Davos, 17 January. https://ec.europa.eu/ commission/presscorner/detail/en/speech_23_232
Wang, Brian. 2022. “China Sunway Supercomputer Team Claims 174 Trillion Parameter AI Model,” Next Big Future, 23 June. https:// www.nextbigfuture.com/2022/06/china-sunway-supercomputer-team-claims-174-trillion-parameter-ai-model.html
Wheeler, Tom and David Simpson. 2022. “5G is smart, now let’s make it secure,” Report, Brookings Governance Studies, December. http://www.brookings.edu/research/5g-is-smart-now-lets-make-it-secure/
Wiggers, Kyle. 2021. “Google trained a trillion-parameter AI language model,” Venture Beat, 12 January. https://venturebeat.com/ai/ google-trained-a-trillion-parameter-ai-language-model/
Yirka, Bob. 2022. “Neuromorphic chip dramatically reduces power requirements for rolling robot,” Tech Xplore, 16 June. https:// techxplore.com/news/2022-06-neuromorphic-chip-power-requirements-robot.html
Viewpoints
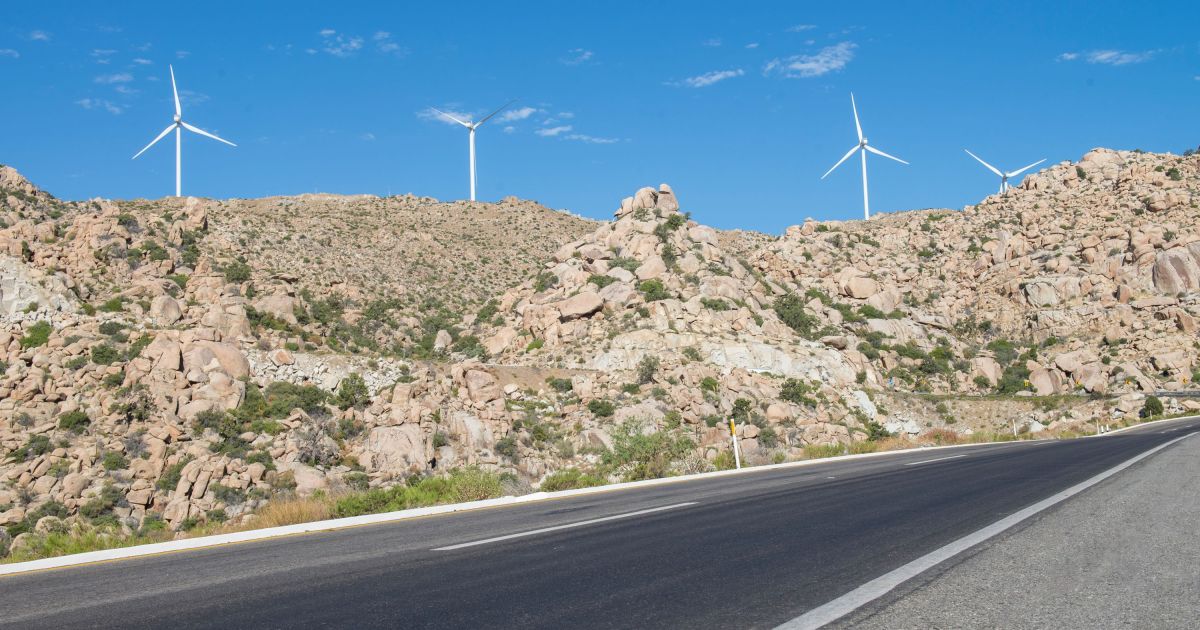
Valeria Moy argues that Canada, the U.S., and Mexico need an integrated and low-emissions North American energy plan in order to develop the digital economy.