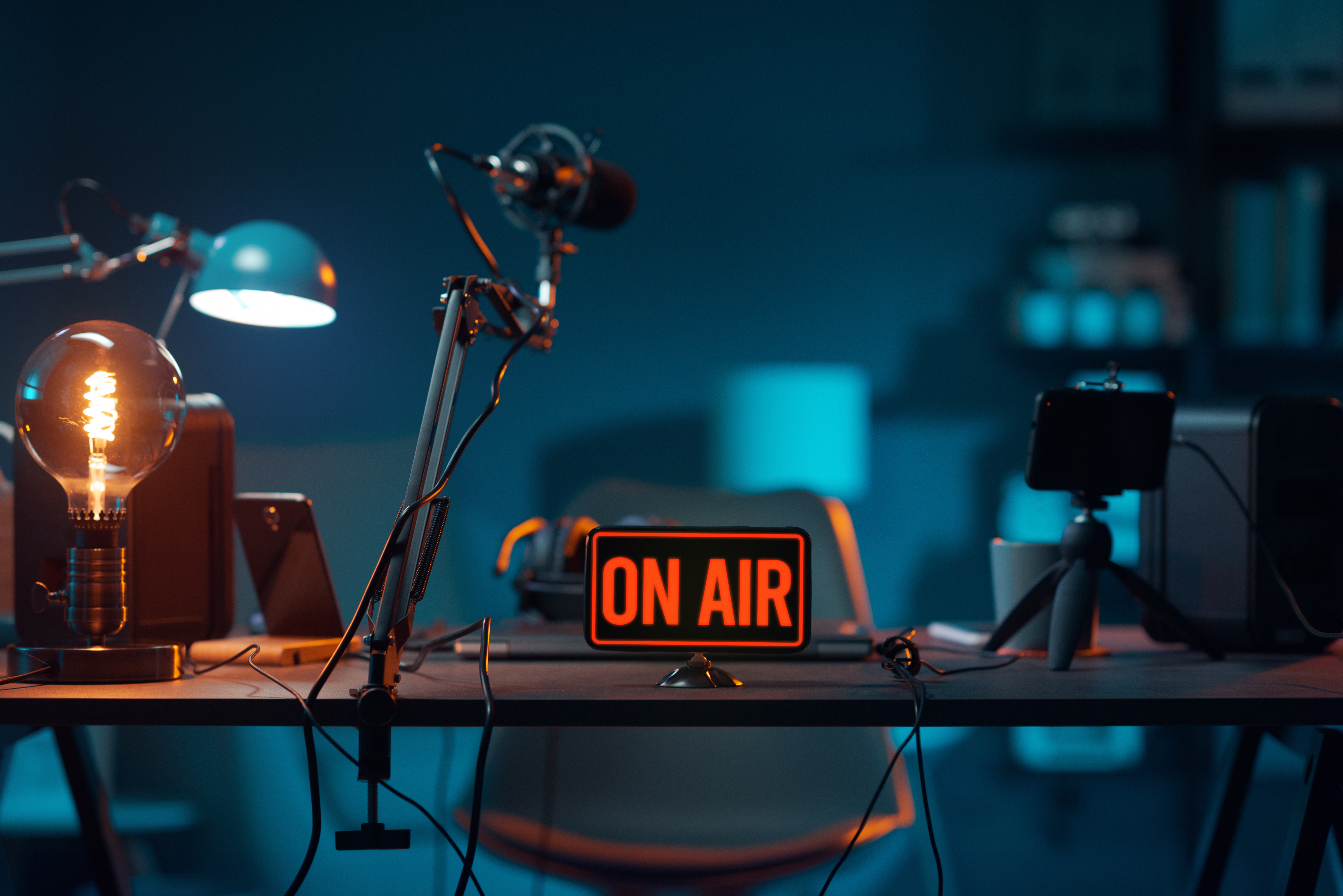
Audible reckoning:
unsubstantiated and false claims
In February 2021, Texas Senator Ted Cruz and his co-host Michael Knowles, a Daily Wire commentator, recorded a live episode of the Verdict With Ted Cruz podcast.1 In conversation about his former Senate race opponent Beto O’Rourke, the Texas Republican described his rival’s support base as primarily reporters who act like “groupies at a Rolling Stones concert throwing their underwear.” Offhandedly, he added, “If they wore underwear, yes.” With a smirk, he leaned into the microphone and asked Knowles, “Too edgy?” Knowles replied, “It’s a podcast: you can say whatever you want!”
Since the advent of the medium, podcasts have generally offered a space where, in the words of Knowles, ‘you can say whatever you want.’ Once written off as a dying medium, podcasting has undergone rapid growth and monetization, while largely avoiding content moderation and regulatory debates.2 Today, nearly 41% of Americans listen to podcasts monthly, and almost one in four Americans look to podcasts for their news.3 Globally, the medium is projected to reach an audience of 504.9 million by 2024, while ad revenue in the United States is expected to double between 2022 and 2024, jumping from $2 billion to $4 billion.4
Podcasts have generally offered a space where, in the words of Knowles, ‘you can say whatever you want.’
Due in large part to the say-whatever-you-want perceptions of the medium, podcasting offers a critical avenue through which unsubstantiated and false claims proliferate. As the terms are used in this report, the terms “false claims,” “misleading claims,” “unsubstantiated claims” or any combination thereof are evaluations by the research team of the underlying statements and assertions grounded in the methodology laid out below in the research design section and appendices. Such claims, evidence suggests, have played a vital role in shaping public opinion and political behavior.5 Despite these risks, the podcasting ecosystem and its role in political debates have received little attention for a variety of reasons, including the technical difficulties in analyzing multi-hour, audio-based content and misconceptions about the medium.6 Yet understanding the scope of this challenge is critically important.
Using a combination of analytical methods – including natural language processing, machine learning, and manual classifying – this research provided the first overarching assessment of the role that popular political podcasting plays in spreading unsubstantiated and false claims. These podcasters, who span the political spectrum, make up the mainstream of the medium and regularly boast audiences in the millions. Drawing on data collected from 36,603 episodes produced by 79 prominent political podcasters, 17,061 evaluations, and 184 key terms and phrases, this analysis found:7
- The spread of unsubstantiated and false claims across the popular political podcasting ecosystem was common: Nearly 70% of podcasters in the dataset shared one or more such claim, and at least one out of every 20 episodes (1,863 episodes) recorded by prominent podcasters featured an unsubstantiated or false claim. Due to the conservative nature of the research design, this is likely an undercount of the proliferation of this type of content across political podcasting.
- Unsubstantiated and false claims tied to the 2020 US presidential election spiked dramatically after the election and did not abate in the following months, despite multiple failed legal challenges: In the lead up to the January 6 attack on the US Capitol, political podcasters played a central and highly partisan role in sowing doubt about the security of the 2020 election. After Election Day, claims of election fraud rose by nearly 600%, to more than 28% of all episodes airing between the election and January 6, 2020, with the vast majority coming from conservative series that were among the most popular during this period.
- During the first two years of the coronavirus pandemic, unsubstantiated and false claims circulated widely, but they tended to be more nuanced and less partisan than election-related claims: Unsubstantiated and false claims tied to the pandemic featured in one out of every eight episodes examined during this period. These claims, however, tended to be less overtly partisan and were often rooted in misunderstandings of science, confusion over evolving public health guidelines, and a heavy reliance on non-peer reviewed preprints.
- 10 prominent podcasters were responsible for sharing the majority of false and misleading content: The 10 podcast series that shared unsubstantiated or false claims at the highest overall rates accounted for more than 60% of all the dataset’s unsubstantiated and false claims. These series, whose hosts lean conservative politically, had a combined reach of more than 28 million followers on Facebook and Twitter as of September 2022.8
Ten hosts are responsible for spreading more than 60% of the unsubstantiated or false claims identified in the data.
As podcasts continue to grow in popularity, addressing the diffusion of unsubstantiated and false content across the ecosystem will become increasingly important. To meet this challenge, tech companies, regulators, listeners, hosts, and researchers all have roles to play. Greater transparency across the board – in terms of content moderation practices, financial disclosures, and algorithmic amplification by podcasting apps – can help to shed light on what has so far been an opaque medium. Improvements to the architecture of podcast apps that allow for a more seamless user experience – including features that let users report and review episodes – would not only create a richer information environment but also effectively reincorporate ordinary listeners as vital contributors to the conversation. Researchers, too, can help to evaluate the substance and broader influence of what remains an understudied communications medium.
While these changes will likely not lead to a complete overhaul of the podcasting ecosystem, they will go a long way toward improving a space that until recently has received little attention from regulators, researchers, and the media, despite the growing influence of podcasting in political conversations.
The evolution of the podcasting medium
In 2004, journalist Ben Hammersley reported on a “new boom in amateur radio” and mused about what to call this space.9 “Audioblogging? Podcasting? GuerillaMedia?” he suggested, perhaps not realizing that he was coining a term for a now multi-billion-dollar industry.10 At the time, the term described serial audio content that could be played on demand through any MP3 device, such as the then-increasingly popular iPod.11 Listeners could take their audio with them, pause it as needed, and start it again at their convenience.12 Early adopters of the medium shared episodes through Really Simple Syndication (RSS) feeds, which users could consume by subscribing directly or indirectly.13 The RSS architecture facilitated the growth of a decentralized medium detached from regulatory oversight. It was a medium where “anyone can be a publisher, anyone can be a broadcaster,” according to one of podcasting’s pioneers.14
In the early to mid-2010s, true crime shows like Serial and nonfiction series like This American Life constituted the bulk of mainstream podcasting. In 2016, the U.S. presidential campaign and election of former president Donald Trump sparked an explosion of political punditry-style podcasts.15 According to relevant data, more than 1,000 political podcasts launched in the year after Trump took office, with most taking a liberal slant.10 Podcasting was even hailed as a “viable and technologically appropriate medium” for left-leaning pundits to “challenge the right’s dominance of American politics.”17 Soon after, conservative talk radio hosts, YouTube personalities, and websites like The Daily Wire and The Blaze launched their own podcast offerings.
Today, series focused on politics and current events regularly feature among the most popular podcasts, as measured by Apple Podcasts’ top 100 rankings.18 Until early 2022, many of these shows were regularly categorized as “news,” although they frequently veer into opinion programming that stretches the traditional definition of the term.19
Unsubstantiated and false claims in podcasting
Combining elements from social media and radio, podcasting has the potential to proliferate unsubstantiated and false claims widely for several reasons. Like Twitter, Facebook, and other social media sites, podcasting has few barriers to entry. As a result, anyone with a podcast can operate as an expert on a subject of their choosing, regardless of their credentials (or lack thereof).20 On social media, other users can push back on false or misleading claims by commenting directly on a public post or crowdsourcing labels that provide additional context.21 In podcasting, however, audience checks on the proliferation of unsubstantiated or false claims are not possible, particularly for individual episodes. Instead, the medium resembles a monologue, more like television or radio. Although some apps allow users to share reviews of podcast series as a whole, audiences are unable to directly react to or share their thoughts about single episodes, an arrangement that makes it easier for false, misleading, or unsubstantiated content to spread with little oversight.
Unlike turning on the radio, listening to a podcast is also more of a deliberate and decentralized act.22 The downloadable nature of a podcast episode means that listeners can begin and pause that episode whenever they want. A radio show, by contrast, happens at a specific time and on a specific station and may not repeat. This distinction is because podcast series are hosted across a wide range of websites – like Anchor, Libsyn, or BuzzSprout – and they can easily be downloaded to and saved on a slew of applications (including Spotify, Audible, or Apple Podcasts) for listening at a later date. As a result, podcasting offers a far more decentralized – and diverse – distribution mechanism than radio. This makes it far more difficult to implement uniform content moderation guidelines for podcasting.
These factors all contribute to the potential for unsubstantiated or false claims to spread widely and unencumbered through podcasts. Until recently, however, researchers have overlooked the medium. The audio-based nature of podcasting, which regularly features hours-long episodes, makes it difficult to monitor the ecosystem given existing research strategies, which until lately have relied more on web domains to help experts identify content deemed “low-quality.”23 Fortunately, natural language processing techniques provide useful tools for assessing non-domain based content, but these strategies require transcriptions of hours of content, which until not long ago could be prohibitively expensive.24 When transcripts are available, evaluating their content at scale remains a challenge: searching for a small claim within hours of text, while allowing for some modifications to that claim, is like searching for a needle in a haystack.
A misunderstanding of the medium by those best equipped to investigate it may also explain the limited attention paid to podcasting. Although it is uncommon for episodes to go viral – or spread rapidly across the digital information ecosystem – on popular podcasting apps, podcasters often have broad cross-platform appeal, making their content likely to reach large audiences. Furthermore, the intimacy and authority attached to the relationship between podcaster and audience may make unsubstantiated or false claims shared in this medium far more impactful than a passing viral tweet from a potentially anonymous account.25 And although podcasting is certainly home to prestige series, it has since expanded to new niches, including political punditry, where hosts from a variety of backgrounds offer regular, often highly polarizing, commentary on current events.
Searching for a needle in a haystack
How common is it for podcasting to spread unsubstantiated and false claims? Assessing this question at scale is challenging because, by some estimates, there are currently more than 75 million recorded podcast episodes.26 Furthermore, there are likely an infinite number of potential false or misleading claims that podcasters could share. This made identifying specific claims across the podcasting ecosystem akin to searching for a needle in a haystack: it would have been likely impossible to find any meaningful signal about podcasts at scale amid millions of hours of noise. As a result, it was important to set clearly defined guardrails that limited the scope of inquiry, while still allowing the researcher to draw meaningful, broad conclusions.
Delineating these guardrails and beginning to answer this question about the extent of false claims in podcasts required three components: (1) a dataset of relevant podcast episodes, (2) a dataset of false and misleading claims, and (3) a methodology for evaluating whether the former includes the latter. But these components were not enough to draw credible conclusions: as much as possible, it was also critical to avoid researcher bias and cherry-picked evidence.
An overview of the process for compiling this information is included below, with details on strategies deployed to limit what is known as “researcher degrees of freedom,” or the process by which seemingly arbitrary design and analysis choices may inflate the significance of any findings.27 More information on all parts of the research methodology can be found in Appendix 3.
Defining the dataset
Given the vast catalogue of podcast episodes and series, defining the bounds of which series to include was the first step. This research focused on unsubstantiated and false claims tied to political issues. As a result, the series of interest were primarily focused on political topics too. The nascent subgenre of political podcasting represents a small, if highly influential, sliver of the overarching podcasting ecosystem. However, it is this space where the spread of unsubstantiated or false claims may have particularly consequential implications for broader public opinion and political discourse. In light of the influence of this space, the analysis focused on a subset of podcasters, referred to as “prominent political podcasters.” These podcasters were identified through a systematic process designed to limit cherry-picking series most likely to contain unsubstantiated or false claims.
The sample of popular political podcasters drew on data from two of Apple Podcasts’ top 100 lists – the first from mid-November 2020 (just after the 2020 election) and the second from mid-April 2021 (in the middle of the coronavirus pandemic).28 The next step involved identifying all talk-show-style series from these two top 100 lists that used the words “policy,” “politics,” “current events,” or “news” in their description or ones that, at the time of data collection, featured an episode on the first page (listing the eight most recent episodes) discussing political topics. The final step involved identifying any political punditry podcasts from the “you might also like” recommendation section in the Apple Podcast app that appeared under the shows selected through the prior step and met the same inclusion criteria for political shows.29 This process avoided including series that would have reinforced researcher biases and created a corpus of series identified in a systematic way. For a full overview of the dataset by series, see Appendix 2.
The analysis detailed in subsequent sections drew on all episode data available for these series through their RSS feeds. Microsoft Azure’s speech-to-text model, a service that provides batch transcriptions of audio files using natural language processing, provided transcriptions of all MP3 files downloaded from the RSS feed.30 These transcriptions, along with captions from some episodes uploaded directly to YouTube, formed the basis of this analysis.31 Although the transcription services occasionally struggled to correctly transcribe some proper nouns (for example, the name of former director of the National Institute of Allergy and Infectious Diseases, Dr. Anthony Fauci), they were generally well suited for the natural language processing methods used in this analysis. Where transcription errors did occur, these errors likely led to false negatives, an undercount of unsubstantiated or false claims, due to an inability to detect word matches and word or phrase similarities.
The report analyzes 36,603 episodes from 79 series, identified in a systematic way.
In total, the dataset included 36,603 episodes across 79 series that were identified in this systematic way. When possible, this included the entire series catalogue, but the data was limited to what podcasters shared on their RSS feeds or provided on their YouTube channels at the time data collection was finalized in late January 2022.
The shows featured in the dataset included conservative, moderate, and liberal podcasters, as well as podcasters of unknown political ideology.32 The sample did, however, include far more conservative podcasters than liberal ones, and even fewer moderate and unknown series.33 As figure 1 shows, conservative podcast episodes were far more common in the dataset, yet podcast series with a conservative host were less than two times more common than series with a liberal host. This is because the conservative podcasters produced more than 2.25 times as many episodes per series as the liberal podcasters did. On average, the conservative podcasters released 620 episodes per series while the liberal ones published only 275 episodes per series. Episodes with conservative hosts were also only slightly longer than episodes recorded by liberal hosts.34
FIGURE 1
Given this sampling strategy, it is also important to emphasize that the inclusion of more conservative political podcasters (or more conservative podcast episodes) in the dataset was not a deliberate choice, but rather a function of how popular these series are across the medium and how likely Apple is to recommend them. An overview of this data is shown below, including the total number of new episodes released each month over time (see figure 2) and the total number of episodes per series (see figure 3).35
FIGURE 2
FIGURE 3
As is evident, the series included in the dataset have primarily produced episodes over the last few years, a trend that demonstrates the recent evolution of the medium and growth in popularity of these shows (see figure 2). Despite this expansion in content, some podcasters are far more prolific than others (see figure 3). The podcast Bannon’s War Room, which began in October 2019, publishes up to four episodes per day, five to six days a week. Other shows, like Pod Save America, which began in January 2017, publish new content roughly two times a week. Thirteen series in the dataset have recorded over 1,000 episodes each. All but two of these thirteen series have conservative hosts.
Because data on series and episode reach is largely unavailable, the Twitter and Facebook follower counts of podcast hosts and/or series serve as a proxy for reach.36 In the dataset, the average podcast host has 1.6 million followers on Facebook and 2 million followers on Twitter across their personal and/or podcast series pages as of September 2022, indicating that these shows have a wide reach.37 Two measures of listeners’ receptivity to a given show were used. First, I compiled data from Apple Podcast reviews, using the platform’s five-star rating system. Second, I assessed the sentiment of the review text using the Valence Aware Dictionary and sEntiment Reasoner (VADER), a rule- and lexicon-based tool for sentiment analysis that is particularly attuned to social media data.38
Cataloguing unsubstantiated and false claims
In addition to systematically limiting the podcast series examined in this analysis, it was also important to restrict the number of unsubstantiated and false claims searched for across podcast episode transcripts. To do this, the research relied on claims compiled in two different ways. The first was the text of claims or statements “fact-checked” as false by either PolitiFact or Snopes, two independent fact-checking organizations whose “fact checks” are widely relied upon by the public and members of the media and academia as credible and well-substantiated. The second was a dictionary of keywords developed using qualitative knowledge and news reports of misleading claims for two case studies focused on the 2020 elections and the coronavirus pandemic.
In total, the dataset of evaluated claims included 11,087 PolitiFact and 5,974 Snopes fact checks. The evaluations focused on a wide range of topics, though elections, non-election politics, health and science, and crime and guns were among the most popular.39 Importantly, both PolitiFact and Snopes provide exact quotes of the unsubstantiated, misleading, or false claims they fact-check, which is ideal for the analysis detailed below.40
The keyword dictionaries for the 2020 election and the pandemic case studies, meanwhile, drew on qualitative knowledge, existing research, a wide range of cross-checks (including ones from The Washington Post, the Associated Press, and Reuters), and general terms used by politicians and public commentators. Additional details on each dictionary are included in Appendix 3. This dictionary approach allowed misleading claims to be explored beyond the specific language of the fact checks, particularly given that fact-checking organizations tend to focus on existing claims made by political figures and more well-known conspiracies already in wide circulation.
Identifying unsubstantiated and false claims in podcast episodes
The final step of the research methodology involved using a two-pronged strategy to identify and classify where unsubstantiated and false claims appear across podcast episodes. This step was particularly challenging due to the vast amounts of podcast content and the evolving nature of the information ecosystem. As a result, searching for specific claims and understanding the intention behind sharing the claims (endorsing versus refuting, for instance) can be difficult. Overcoming these obstacles required a variety of natural language processing techniques to identify specific parts of episodes that matched unsubstantiated or false claims. After this automated process, at least two coders manually reviewed any matches to assess whether the episode was sharing or refuting an unsubstantiated or false claim.
Cosine similarity approach using fact checked text
The first strategy used to identify when specific claims fact-checked as false appeared in podcast transcripts was a text similarity measure called the “cosine similarity.” The cosine similarity is a measurement technique that calculates the relationship between two documents (or text snippets) by encoding each document as a vector, with each word of the document representing a coordinate in the vector. The cosine similarity is then calculated using the cosine of the angle between the two vectors.41 The resulting output is a score from zero to one, with a higher number representing texts that are more similar.42 I calculated the cosine similarity between every fact-checked claim and all the podcast transcripts. A team of coders then manually reviewed the matches that scored above 0.5. This threshold meant that part of the transcript and the fact-checked claim were at least somewhat related.
Dictionary-based approach
In addition to calculating the cosine similarity between transcripts and fact-checked claims, the analysis also involved searching episode transcripts for a list of keywords associated with different claims tied to either electoral fraud or the coronavirus pandemic. This included both generic terms such as “stolen election” and “phony pandemic” as well as references to specific conspiracy theories or misleading claims, such as “Sharpies” or “microchip.”43 At least two researchers then manually reviewed matches between the dictionary of terms and the transcripts.
Review processes
As mentioned above, for any episode flagged as a match with either the dictionary or cosine similarity approach, at least two coders independently reviewed the transcript text and associated fact checked claim or dictionary term match. If the initial two coders agreed in their evaluation that the post appeared to contain unsubstantiated or false claims, it was evaluated as such. Where the two coders disagreed, a third coder reviewed the transcript and associated claim. In total, just 3% of all reviewed claims required a third coder.
This last step, which involved hand-coding and manual review, means it is unlikely that the dataset included false positives (meaning instances where an episode was coded as containing a false or misleading claim when it should not have been), but the dataset may have included false negatives (meaning instances where a transcript was coded as not containing a false or misleading claim when it did contain one). This is because a podcast host or guest may have made a false claim that did not trigger a keyword or fact-check match. Importantly, coders considered shifts in knowledge in their classifications and generally did not code opinions as unsubstantiated or false. Episodes were only evaluated as sharing unsubstantiated or false content if a host wholly endorsed a claim, shared it in the context of broader positive commentary, or allowed a guest to share a claim without challenging its veracity.
Podcasting as a primary vector for spreading unsubstantiated and false claims
On July 2, 2021, conservative podcaster Michael Savage, whose radio show in the mid-2000s drew up to 8.25 million listeners a week, reflected on the presidency of Donald Trump using a blend of conspiracy tropes and fiery rhetoric.44 As a “lapsed Jew,” he was disappointed by the recent rise of “antisemitic conspiracy theories,” such as a narrative that impeachment represented a “Jew coup.”45 Referring to the impeachment as an attempt to “crucify Trump without any evidence,” he rejected the characterization of the supposed coup attempt as wholly led by Jews. Instead, he noted how many of the politicians pursuing Trump, “like [Adam] Schiff [and Jerry] Nadler,” were not representative of the Jewish perspective. And he asked his listeners to remember that the so-called mastermind behind this supposed plot was former House speaker Nancy Pelosi who, in Savage’s view, was “using Jewish people to do her dirty work.”45
Savage’s rhetoric represents a potent example of the type of unsubstantiated and misleading claims that spread across political podcasting, which often build on the past tactics of leading talk radio hosts (many of whom have since become prominent podcasters).47 And for podcasters, the largely ungoverned space offered by this new media medium means that this type of content often flies under the radar if it is addressed at all.
Drawing on data from 36,603 episodes produced by 79 prominent political podcasters, 17,061 evaluations, and 184 key terms and phrases, this research documented the spread of unsubstantiated or false claims across the political podcasting ecosystem. The analysis found that:
- One out of every 20 episodes (1,863 episodes) in the dataset shared at least one unsubstantiated or false claim.
- More than 70% of all the podcasters (56 series) in the sample shared at least one unsubstantiated or false claim; 15% (12 series) shared 50 or more such claims.
- Even after accounting for the potential partisan skew of fact checkers (detailed below), conservative podcasters were 11 times more likely than liberal podcasters to share claims fact-checked as false or unsubstantiated.
Unsubstantiated and false content over time
Strikingly, nearly 2,000 episodes in the dataset, more than one out of every 20 episodes, shared at least one piece of false or unsubstantiated content. This is particularly notable given that prior research has found the sharing of such content to be a rare event, with engagement rates of less than a minute per day, according to one estimate.48 Furthermore, given the strict research strategy for evaluating these claims, this likely represents the lowest possible floor for the prevalence of such unverified claims in the popular political podcast ecosystem, which (importantly) only includes mainstream podcasters.
FIGURE 4
Figure 4 highlights the total number of podcast series in the dataset that featured unsubstantiated or false content, broken down by the partisan leaning of the hosts. According to the analysis, 56 of the 79 series (71%) shared at least one false or unproven claim, 29 shared more than five claims (37%), 12 shared more than 50 claims (15%), and two shared more than 200 claims (2.5%). Of the series that shared five or more unsubstantiated or false claims, nearly all had hosts who were ideologically conservative.
FIGURE 5
At least one in every 20 episodes featured one or more unsubstantiated or false claims.
Although several shows included unsubstantiated or false claims in more than 100 episodes, many of these shows produced thousands of episodes throughout the period of analysis. Furthermore, several shows that shared unsubstantiated or false claims did so at a higher rate, due to a smaller number of total episodes. Figure 5 plots the shows that shared unsubstantiated or false content as a percentage of total episodes, as well as whether those shows entered the dataset due to their popularity or Apple’s recommendation system. Bannon’s War Room, which produced both a high number of episodes and shared the most unsubstantiated or false claims in the dataset, topped the list, with close to 20% of all episodes assessed during this period including claims that Snopes and Politifact fact-checkers or the terms dictionary flagged as false or unsubstantiated. Seven other shows featured false or unsubstantiated claims in more than 10% of all episodes. Of these shows, just two did not feature in Apple Podcasts’ top 100 in April 2021 or November 2020.
Collectively, the hosts of the shows that shared the highest percentage of unsubstantiated or false claims reached an audience of more than 28 million followers on Facebook and Twitter as of September 2022, though the reach per show varied significantly, from around 180,000 followers to more than 10 million.49 They also shared more than 60% of all claims flagged as unsubstantiated or false in the podcast dataset.
Importantly, Apple’s algorithm seemed to only play a small role in funneling users toward content with unsubstantiated or false claims. Of the approximately 2,000 claims tallied in this report, nearly 1,500 appeared in series featured in Apple Podcasts’ top 100 shows (7.5% of all Top 100 episodes) and 500 appeared in series identified through Apple’s recommendation section (2.7% of all recommended episodes). While Apple’s recommendation algorithm may drive users to more extreme content, the first layer of recommendations, at least in this study, contributed less to overall exposure to unsubstantiated and false claims than did shows identified based on their popularity.
Accounting for fact checker bias in partisan disparities on sharing false claims
Notably, the perceived or real possibility of partisan bias by fact checkers does not explain differences in why conservative podcasters shared false and unsubstantiated claims more often than liberal hosts did.50 A total of 1,182 unique fact-checked claims appeared in the podcast episodes, as hosts either refuted such claims or shared them. These claims cut across the political spectrum but were skewed toward statements made by conservative public figures: conservative figures accounted for 928 fact-checked statements and liberal figures accounted for 254.51 The represents a nearly four-to-one disparity in the partisan bent of fact-checked claims.
As sizable as this partisan disparity is, the right side of figure 6 shows podcast hosts endorsed or uncritically shared 42 times as many false statements made by conservative figures (a count of nearly 700 versus merely 16). Given the four-to-one partisan disparity in fact-checked statements, podcasters could in theory have shared four times as many false statements made by conservative figures due to this imbalance. Despite accounting for this disparity (by, for instance, multiplying 16—the number of false claims shared across podcasts that were made by liberal figures—times 4—to factor in the four-to-one difference in fact-checked claims of conservative and liberal figures), podcast hosts were still 11 times more likely to share claims by conservative figures fact-checked as false than such claims by liberal figures. And podcasts with conservative hosts shared the vast majority of these statements.
FIGURE 6
Notably, conservative podcast hosts were also more likely to refute claims fact checked as false (as shown on the left side of figure 6), with hosts in 20 episodes citing PolitiFact or Snopes directly to bolster their commentary. Across all podcast series, conservative podcaster Ben Shapiro, host of The Ben Shapiro Show, was both the most likely to directly refute a false claim (nearly 50 episodes) and the most likely to refute a false claim made by a public figure from his own partisan perspective (around twenty episodes).
As previously mentioned, the fact checks shared in the dataset focused on a wide range of topics, though elections, non-election politics, health and science, and crime and guns were among the most common subjects for false claims (see figure 7). The tallies detailed here are broader in focus and do not incorporate the two keyword dictionary case studies (COVID-19 and election fraud), instead focusing on other specific statements circulating widely in public discourse. Given that by some counts Trump made more than 30,000 false or misleading claims during his presidency, it is perhaps unsurprising that podcast hosts were more likely to both refute and share claims made by the former president that were fact-checked as false (see figure 8).52 Podcasters also commonly refuted false claims made by President Joe Biden, though they were not among the top ten most shared.
FIGURE 7
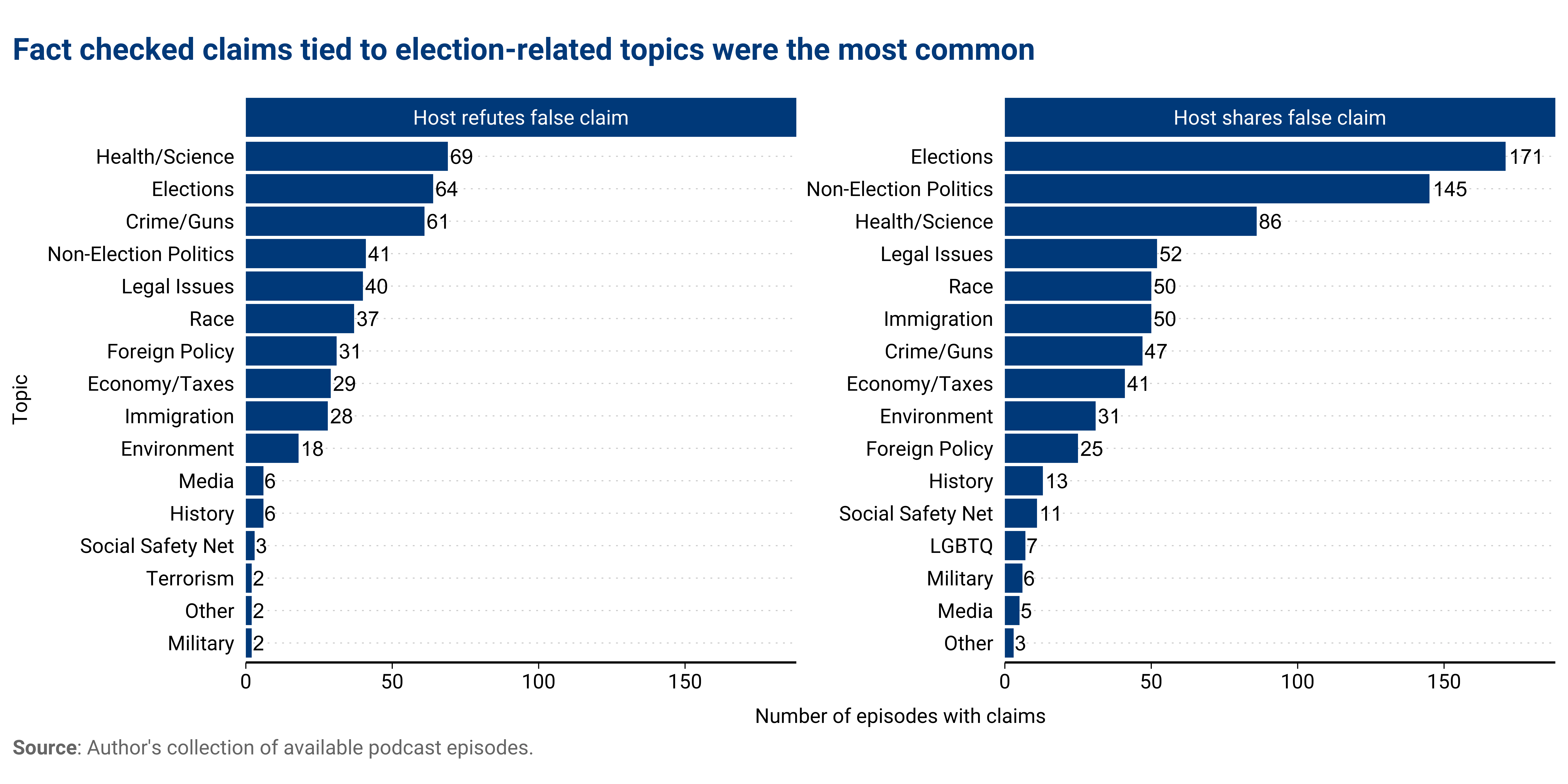
FIGURE 8
User sentiments toward podcasts with false or unsubstantiated claims
Can podcast listeners distinguish between series that shared false or unsubstantiated information and those that did not? To begin to answer this question, I collected all text reviews from the podcast series in the dataset. I assessed both the average scores for Apple Podcast reviews, on a scale of 1 to 5, and the average substance of those reviews, on a scale of –1 to 1, with –1 being very negative and 1 being very positive. To calculate the sentiment of the review text, I used VADER for sentiment analysis on both the body and subject of the reviews.53 The average rating for series in the dataset was overwhelmingly positive: for Apple it was 4.36 and for VADER it was 0.48. These rating measures were also both highly correlated: a show with a higher average VADER score also tended to have a higher average Apple rating. Yet what one person rates a 3 on Apple might be another person’s 1, despite the similar tenor of their reviews. As a result, I relied on both measures to assess user engagement with popular podcast series.
FIGURE 9
Based on figure 9, the results are mixed. By some measures, users may be able to distinguish between series that share false or unsubstantiated information and those that do not, but only some of the data seems to show this. While the VADER method showed an association between the proportion of episodes sharing false or unsubstantiated content and more negative reviewer sentiment (meaning that shows that shared a higher proportion of false and unsubstantiated claims tended to receive more negative reviewer comments), using Apple’s score as a measure of reviewer support was inconclusive. Furthermore, these results were based off simple correlations, and several confounding variables may complicate this relationship. Nevertheless, these results point to a potential area of future research and highlight the possible benefits of allowing users to engage with content more thoroughly by reviewing and rating individual episodes.
Prominent political podcasters played a key role in spreading the ‘Big Lie’
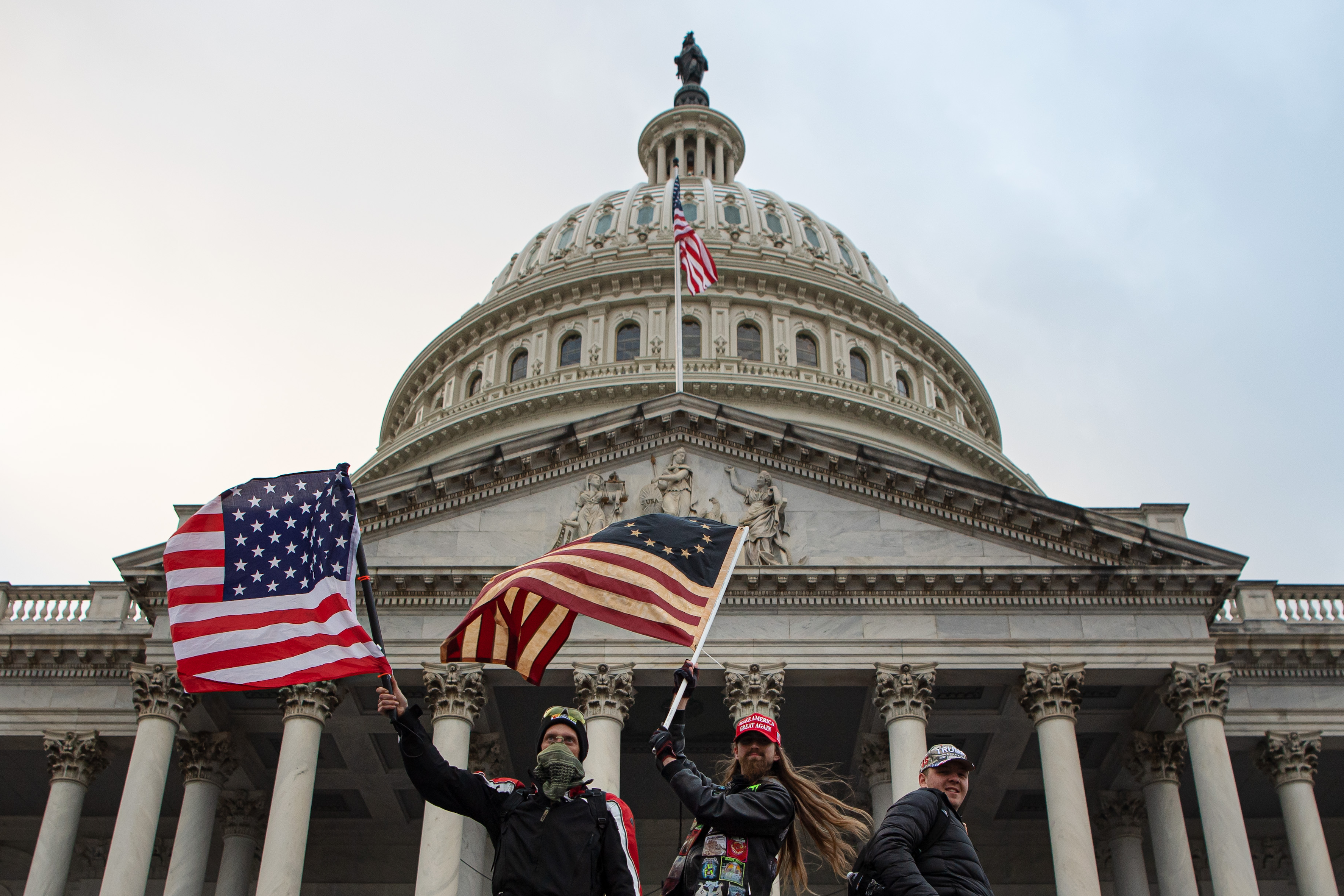
In an episode that aired on January 6, 2021, titled “For the Republic,” former Trump administration chief strategist Steve Bannon encouraged his audience to keep the faith: “We’re coming in right over target . . . This is the point of attack we always wanted . . . today is the day we can affirm the massive landslide on November 3.”54
In hindsight, Bannon’s podcast episode offers a chilling example of the types of unsubstantiated and false claims spread by prominent political podcasters after the 2020 election. After voters cast their ballots, podcast hosts and guests regularly promoted the lie that the election had been stolen from Trump, relying on debunked evidence, hearsay, and blatant conspiracy tropes to bolster their claims.55 Following the attack on the Capitol, social media platforms such as YouTube, Facebook, and Twitter faced more scrutiny for their contributions to spreading these narratives.56 At the time, podcasts barely registered as a concern.
To better understand the role podcasts played, this research evaluated the content of 3,916 podcast episodes that aired between the first major party convention on August 20, 2020, and the storming of the Capitol on January 6, 2021. The analysis found that:
- One out of every seven episodes endorsed unsubstantiated or false election claims, with most of these instances coming from more conservative series that were among the most popular at the time.
- For the most popular series at the time, election fraud claims featured in 27% of all episodes, as compared to 5% of episodes for shows that featured in the “you might also like” section or only were listed on Apple Podcasts’ top 100 list in April 2021.
- After the election, claims of election fraud rose by 600%, from 3% of all episodes between August 20 and November 2, 2020, to more than 28% of all episodes between November 3, 2020, and January 6, 2021.
- For shows in the Apple Podcasts’ top 100 at the time, this number reached over 50% of all episodes in the period after the election.57
- A total of 29 of 79 (37%) different shows shared at least one false or misleading election narrative, with nine shows sharing 25 or more claims or devoting more than 50% of their post-election episodes to election fraud.
These findings suggest that, prior to the January 6 attack, political podcasters – particularly the most popular ones at the time – served as a critical and underappreciated vector for the spread of unsubstantiated and false claims tied to the election. With 2020 election deniers still running for elected office at high rates in 2022 and Trump’s announcement that he plans to seek the 2024 Republican presidential nomination, the role of new media, including political podcasting, in framing these conversations requires additional research and scrutiny.58
The ‘Big Lie’ in the mainstream
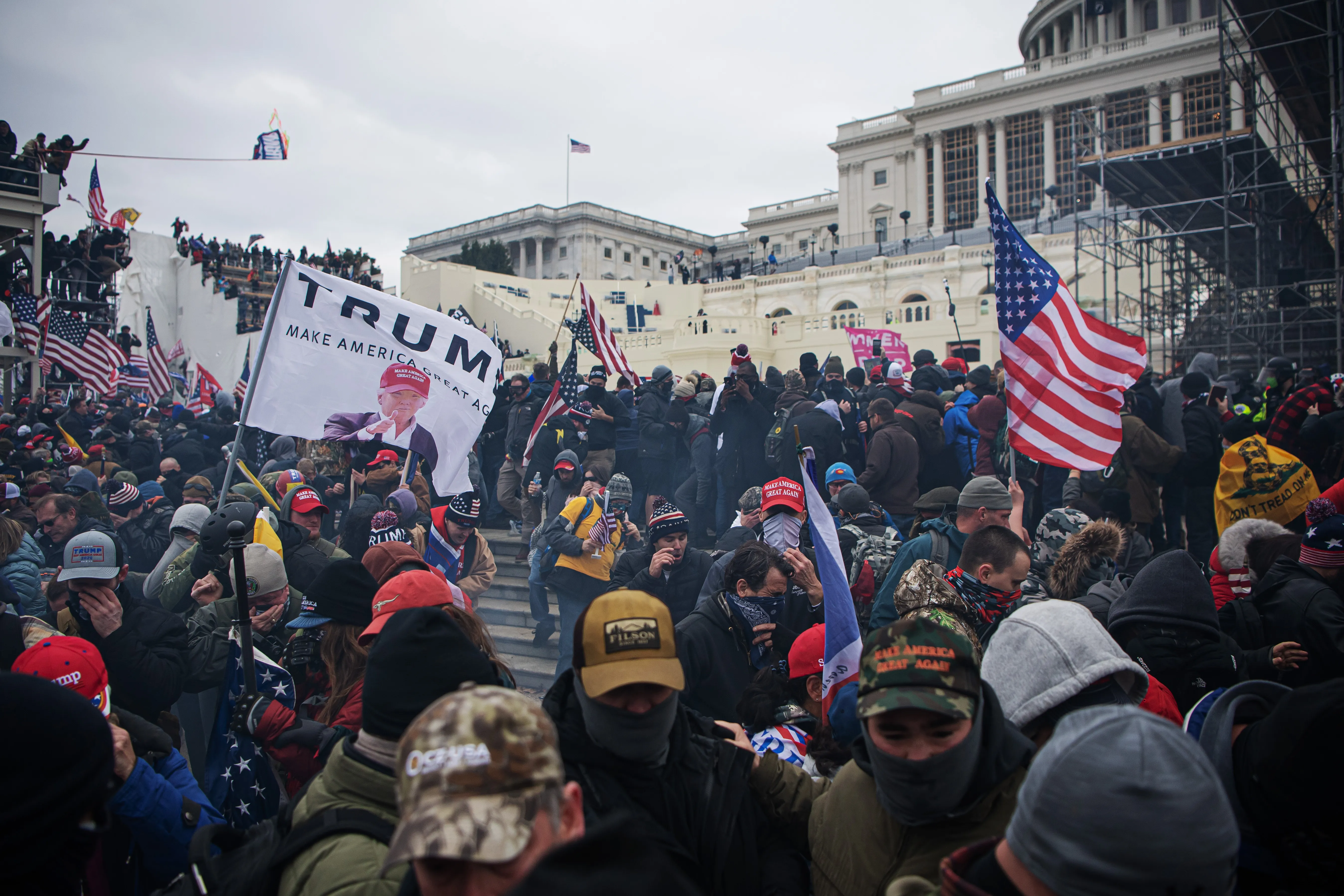
As figure 10 reveals, there was a massive post-election increase in podcast episodes that endorsed unsubstantiated allegations of voter fraud and related narratives, with 88% of all claims about election fraud occurring after the 2020 election.59 Although podcasters occasionally raised concerns about electoral integrity before the election, the percentage of all episodes that shared unsubstantiated or false claims about election fraud remained low before Election Day. After the election, the number of claims spiked dramatically. In some weeks, podcasters shared nearly 10 claims per day. Among the political shows in Apple Podcasts’ top 100 during this period, claims of election fraud featured in over 50% of all episodes, suggesting that hosts with the largest audiences at the time drove the perpetuation of these narratives across podcasting.
FIGURE 10
After the election, claims of election fraud on political podcasting rose by 600%.
Strikingly, the post-election spike in misleading electoral claims also proved to be remarkably durable. After the Electoral College’s “safe-harbor” deadline on December 8, 2020 – when states are supposed to have finished resolving election-related disputes – and countless failed court cases, including a case rejected by the Supreme Court on December 11, observers might expect a dramatic decline in fraud-related claims across the podcasting ecosystem.60 Although the data registers a modest decline around the holidays, in the week prior to the Capitol assault, around five episodes per day endorsed election fraud narratives, despite these substantial legal defeats.
The spread of election fraud narratives on political podcasts during this period was important for the continued perpetuation of these claims, particularly as social media platforms and mainstream media outlets took action to stem their reach. For example, after the safe-harbor deadline passed, YouTube launched efforts to remove content alleging that “widespread fraud or errors changed the outcome of the 2020 U.S. Presidential election,” and the video-hosting website continued linking users searching for election-related information to third-party fact checkers and authoritative content.61 Facebook took preemptive action to stem the reach of political content prior to the election, implementing what they called “break-the-glass” measures that restricted the viral spread of content from political groups where election falsehoods tended to thrive.62 After the election, Facebook banned a viral “Stop the Steal” campaign group, though some employees and onlookers criticized these measures as too lax and temporary.63 Twitter also took action by flagging posts from Trump and others who violated its policies related to election fraud claims and adding warning labels and more context to election-related tweets.64
Even mainstream conservative media outlets distanced themselves from Trump’s claims that the election had been stolen. Just after the election, some Fox News hosts cut away from election fraud briefings by Trump administration officials, and outlets owned by media mogul Rupert Murdoch encouraged Trump to concede.65 In response to a legal threat from the election software company Smartmatic, Fox News also aired a news segment debunking various allegations of election fraud about the 2020 election, including some shared by its own hosts.66 Although the restrictions put in place failed to wholly halt the spread of election fraud claims across social and mainstream media, the false allegation that the election had been stolen spread on conservative podcasts unencumbered and with little scrutiny.
As figure 11 illustrates, much of the content tied to election fraud was driven by a few shows that aired many episodes and frequently shared misleading narratives tied to election integrity. Although 29 podcast series shared at least one unsubstantiated or false election-related claim, nine series shared either 25 or more claims or devoted more than 50% of their post-election episodes to election fraud. Of note is Bannon’s War Room. In keeping with his Trump-era media strategy of “flooding the zone” with inflammatory information, Bannon not only devoted more than 130 episodes to promoting election fraud narratives but also did so on nearly 80% of all episodes recorded during this period.67
FIGURE 11
A number of these podcasters devoted significant attention to making election fraud claims seem credible by pointing out the legal consequences of signing a false affidavit, and they highlighted the careers of Trump’s lawyers, including Rudy Giuliani (who “took down the Mafia in New York City”) and Sidney Powell (who “worked for Michael Flynn”) as a signal of their prestige.68 They also regularly shared some of the most implausible election fraud claims, including those that linked deceased Venezuelan leader Hugo Chavez to election fraud and blamed Biden’s victory in Arizona on the distribution of Sharpie pens.69
Due to podcasts’ decentralized nature, and because podcasters often cross-post on other websites or rebroadcast over terrestrial radio, it is difficult to grasp the overall size of the audience for these episodes. Despite this challenge, other data points can at least provide a glimpse into their possible reach. In March 2022, Bannon’s War Room, for example, claimed to have been downloaded over 135 million times.70 The Verdict with Ted Cruz reportedly had at least 20 million downloads and YouTube views in 2020.71 In the dataset, hosts that shared unsubstantiated or false content had a total of 58 million followers on Twitter and 51 million Facebook followers. As of September 2022, the average number of followers across Twitter and Facebook for hosts sharing content on election fraud was nearly 4 million, and the median host enjoyed a reach of 2 million across both platforms.72 As social media platforms and mainstream media outlets cracked down on claims of election fraud in late 2020, it was clear that these narratives found a home among a subset of prominent political podcasters who continue to enjoy widespread reach.
How podcasters spread unsubstantiated or false claims during the COVID-19 pandemic
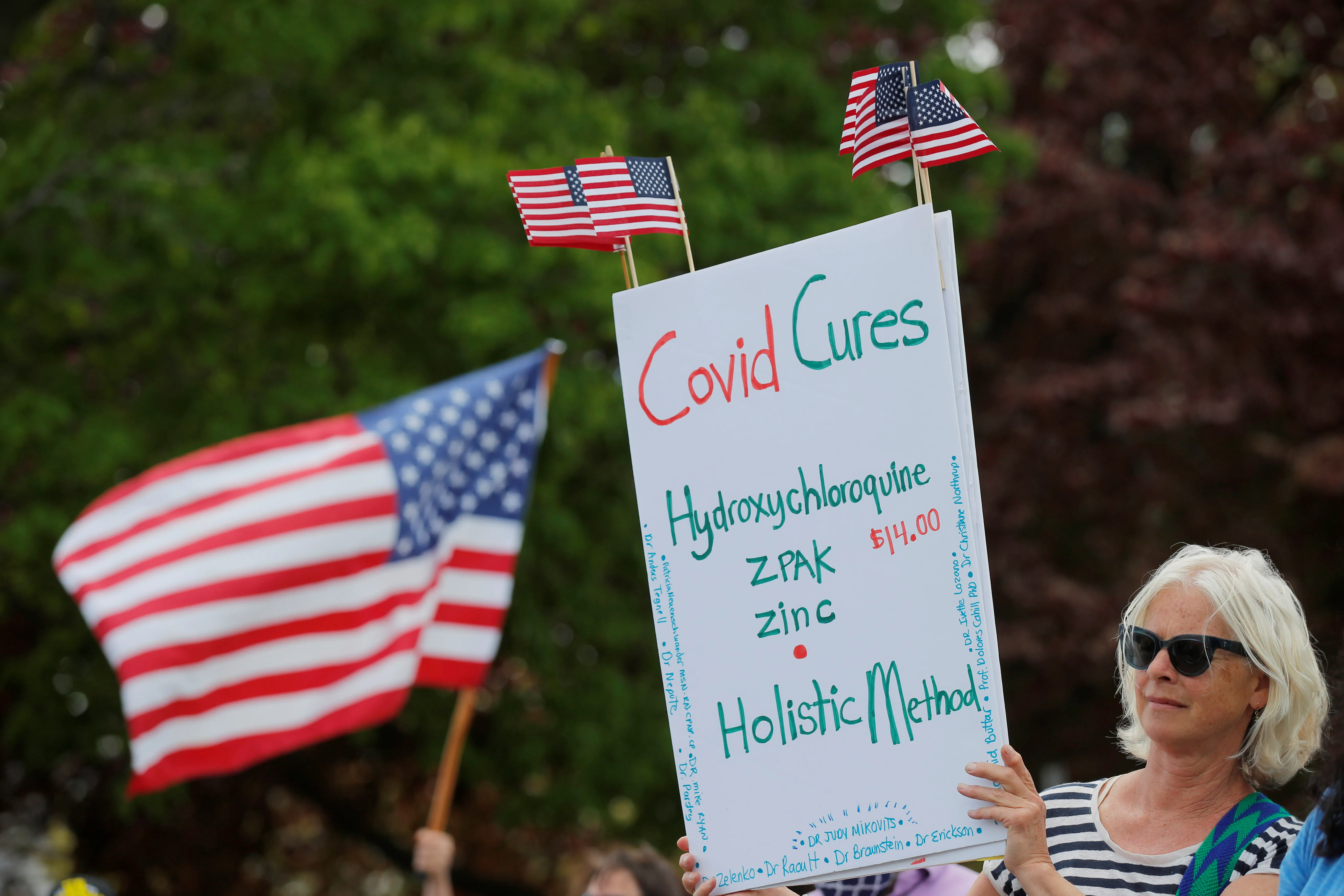
Days before the first coronavirus death struck the United States in late February 2020, podcaster Glenn Beck, founder and CEO of The Blaze, warned his listeners of the virus’s potential impact should it spread: “the coronavirus is going to hammer the global economy if it becomes a pandemic.”73 But the looming threat of a global pandemic did not register for many podcasters. As coronavirus infections and deaths soared in New York City in April and May 2020, offering a stark warning for the rest of the nation, podcaster Daniel Horowitz, whose podcast promises listeners to “explore the politically right way to think about the issues,” downplayed the severity of the disease: “if you take out New York City, which is unique, how many people is that? You’re talking about getting struck by lightning. You’re talking about risk levels that are not elevated above any other activity associated with your daily lives.”74 Similarly, a guest on the show hosted by John Solomon, a former Fox News contributor and executive and editor-in-chief at The Washington Times, argued that the coronavirus “does exactly what all other respiratory disease epidemics during the flu season do: they come for two weeks, they stay at the peak, they go, and it’s over.”75
Perhaps more so than any other issue, the pandemic has illustrated both the many devastating, well-documented consequences of – and the challenges with classifying – unsubstantiated or false claims.76 According to the Department of Health and Human Services, the “infodemic” of unsubstantiated and false content tied to the pandemic “has led people to decline vaccines, reject public health measures, and use unproven treatments. Health misinformation has also led to harassment and violence against health workers, airline staff, and other frontline workers tasked with communicating evolving public health measures.”77
Understanding how and why these claims spread across popular information platforms is of vital importance. Since the pandemic began, social media companies like Meta, Twitter, and Google have played a leading role in moderating these conversations, with mixed success.78 Podcasting remains absent from these discussions, despite some referring to the unregulated medium as the “Wild West” for unsubstantiated or false claims related to COVID-19.79 Yet apart from public backlash against podcast host Joe Rogan for promoting unproven COVID-19 treatments, the spread of unsubstantiated or false claims tied to the pandemic through popular political podcasting has not received significant attention.80
To understand how podcasters have shaped conversations about the pandemic, this research restricts the original data frame to episodes that aired after January 19, 2020, when the country’s first coronavirus cases appeared in Washington state and analyzed the contents of more than 5,000 episodes.81 This analysis relied exclusively on the COVID-19 dictionary (see Appendix 3 for more details). The analysis found that:
- Unsubstantiated or false claims tied to COVID-19 were common, featuring in one out of every eight COVID-focused episodes examined during this period.
- These claims primarily focused on unproven, alternative treatments and preventions, with more than three times as many episodes promoting these claims as the next most common category tied to vaccine efficacy and side effects.
- The official transition from the Trump administration to the Biden administration did not visibly alter the spread of unsubstantiated or false claims tied to the coronavirus.
- Spikes in unsubstantiated or false coronavirus-related claims can be clearly tied to specific events throughout the pandemic. For example, the single most consequential event for content tied to alternative treatments and prevention was a press conference held by a controversial group called America’s Frontline Doctors that touted the benefits of hydroxychloroquine in front of the Supreme Court building in July 2020.
- Fringe conspiracy theories, like the claim that COVID-19 vaccines contain microchips, were mostly absent from coronavirus discussions among popular political podcasters. Instead, unsubstantiated and false claims were far more nuanced and rooted in misunderstandings of science, confusion over evolving public health guidelines, and a heavy reliance on non-peer reviewed preprints.
A focus on unproven treatments
Podcasters helped perpetuate this infodemic of false or unsubstantiated claims about the pandemic, eroding public trust in healthcare professionals and hindering the country’s pandemic response.82 In total, one in every eight episodes contained claims not backed by scientific consensus. Thirteen series in the dataset shared 10 or more unproven claims tied to the pandemic. Although audience statistics for these series are unavailable, collectively, their hosts reached nearly 40 million followers across Twitter and Facebook as of September 2022.83
Claims about alternative treatments and preventions were more common than all other type of COVID-19 related claims combined.
As figure 12 demonstrates, these claims most commonly focused on promoting alternative treatments for and prevention of COVID-19.84 Among these claims were the beliefs that hydroxychloroquine (an anti-malaria drug) and ivermectin (an anti-parasitic drug) could treat or prevent COVID-19 altogether. In the early days of the pandemic, Trump and other public figures speculated about the efficacy of hydroxychloroquine, and the Food and Drug Administration (FDA) listed the drug as a potential treatment and prophylaxis for hospitalized COVID-19 patients.85 Following reports of cardiac adverse events in COVID-19 patients who had received hydroxychloroquine treatments, the FDA issued statements cautioning people against using the drug outside of a hospital or clinical trial. The president, however, continued to tout its purported efficacy. By June 2020, the FDA had already revoked hydroxychloroquine’s emergency use authorization (EUA).86 The Trump administration called the move a “Deep State blindside by bureaucrats” and continued to push the medication into the summer of 2020, even as many public health officials deemed it “unlikely to be effective in treating COVID-19 for the authorized uses.”86 Podcast claims about the efficacy of hydroxychloroquine, which researchers only began coding after the FDA revoked its EUA, skyrocketed in the summer of 2020 and remained a constant feature throughout the period of analysis.
FIGURE 12
Podcast conversations about using ivermectin to treat or prevent COVID-19 emerged more organically than talk of hydroxychloroquine did, as part of a pundit-led process instead of government-driven politicization. The first reference to ivermectin in the dataset was in August 2020, when a guest on prominent political pundit Charlie Kirk’s podcast included it on a list of drugs that “should also be available to doctors.”88 In early September 2021, podcast host Joe Rogan revealed that he tested positive for COVID-19 and had been taking ivermectin to mitigate some of the virus’s worst symptoms.89 Although the Centers for Disease Control and Prevention (CDC) had warned that ivermectin was “not authorized or approved by [the] FDA for prevention or treatment of COVID-19,” the drug quickly gained mainstream attention, though conversations about it had been circulating in political podcasting since early 2021.90
Importantly, the presidential transfer of power from Trump to Biden did little to affect the trajectory of the coronavirus infodemic, with many unsubstantiated and false claims spreading both before and after the election. Figure 13 shows the course of these claims over time. Although these unsubstantiated and false claims have become a more regular feature during the Biden presidency, this trend also coincided with the early stages of the COVID-19 vaccine’s public rollout, which offered a new focus for these claims not previously available to podcasters during much of Trump’s presidency, although claims about alternative treatments and preventions still remained a common feature of podcast episodes.
FIGURE 13
Instead of the election, key moments and events in the evolution of the pandemic seem to have driven surges in this type of content. For example, the single most consequential event tied to alternative treatment claims was a press conference held outside the Supreme Court building in late July 2020 by America’s Frontline Doctors, a controversial group of medical professionals linked to a conservative group known as the Tea Party Patriots.91 At this rally, Dr. Stella Immanuel, a Houston-based medical practitioner and religious leader, proclaimed, “America, there is a cure for COVID. All this foolishness does not need to happen. . . . There is a cure for COVID . . . called hydroxychloroquine. It’s called zinc. It’s called Zithromax.”92 Social media users quickly picked up the video, which led platforms to crack down on its distribution, prompting backlash against perceived censorship and spurring broader distribution, including across the podcasting ecosystem. Within two weeks of Immanuel’s press conference, at least 42 podcast episodes shared claims about hydroxychloroquine’s efficacy (around three claims per day). In these episodes, podcasters like Mark Levin quoted from and discussed Immanuel’s press conference at length, highlighting support for hydroxychloroquine from “professors from the finest institutions” and “exquisitely educated and experienced doctors from the finest hospital.”93
Similarly, the release of Dr. Fauci’s emails on June 2, 2021, via a Freedom of Information Act request, marked the single most consequential event for conspiracy-related content. His emails included exchanges with domestic and foreign government and public health officials, journalists, and everyday Americans regarding daily briefings and, notably, speculation during the early stages of the pandemic about the efficacy of masks and the “lab leak” theory that the virus was human-made or manufactured and accidentally escaped from the Wuhan Institute of Virology in China. Following the release of these emails, podcasts like Steve Bannon’s War Room seized the opportunity to push theories that Fauci knew early on that this was a “genetically engineered bioweapon” and “decide[d] not to tell the president” and speculation that Fauci and others “stood to profit if this turned into a pandemic.”94
Following the widespread rollout of the vaccine in early 2021, vaccine-related claims remained steady over time, with a slight spike when vaccine-induced immunity began to wane during the height of the Omicron wave in early 2022, necessitating the need for booster shots. During this period, podcasters questioned the safety of vaccines by highlighting research that seemed to claim that “death after the vaccine at any age group is more likely than actually taking your chances with COVID-19.”95 In one recorded episode of the Charle Kirk Show, guest Vladimir Zelenko compared the vaccine to a “bomb inside you” and stated, “what we really have is a poison death shot—it’s not a vaccine.”96
Science in the face of uncertainty
Researchers coded few instances of prominent political podcasters promoting fringe theories and ideas. For example, not one podcaster in the dataset endorsed claims about microchips in vaccines or injecting bleach as a COVID-19 repellent, among other more far-fetched claims commonly discussed on social media and in the news. The most frequently shared conspiratorial claim was that COVID-19 resulted from a Chinese Communist Party–led (CCP) plot to stop Trump’s reelection. As figure 14 shows, Bannon’s podcast alone accounted for nearly three-quarters of all conspiracy-related content on political podcasts (see the right side of the figure) and more than one-quarter of all COVID-related claims (see the figure’s left side). In one episode, Bannon’s War Room lauded efforts to reveal “the truth about the coronavirus being made and re-engineered by the CCP and intentionally released to the whole world as part of their evil plan.”97 In another guest Peter Navarro, who served in a number of positions in the Trump administration, added, “I was looking at the classified information, and all hell was going to break loose, and I’m thinking is this a bioweapon that they’re gonna take President Trump down with?”98
FIGURE 14
Yet apart from these conspiracy theories, unsubstantiated or false claims shared at this time were far more nuanced and primarily involved misunderstandings of science. Several prominent podcasters carved out positions of authority during this period of high uncertainty. For example, Heather Heying and Bret Weinstein relied on their backgrounds in evolutionary biology to amass a wide audience for their unconventional coronavirus advice. The pair repeated unproven claims about the prophylactic use of various treatments, including ivermectin and hydroxychloroquine, and cast doubt on vaccine safety.99
Throughout their episodes, Heying and Weinstein tried to bolster perceptions of their credentials by using technical terms. In one episode, Weinstein offered a “little brain teaser,” saying that “correlation is in fact evidence of causation if there was a causal hypothesis pre-existing the observation of the correlation.”100 While it’s unclear exactly what he is referencing, perhaps the most basic tenet of statistics emphasizes that correlation does not equal causation and is instead susceptible to confounders.101 Although researchers can control for potential known confounders, there may be infinitely more unknown confounding variables driving any observed relationship. Rather than protecting researchers against confounding, a pre-existing hypothesis may instead make them more prone to confirmation bias. Weinstein, however, may have deployed this language to appear to burnish the scientific bona fides of the hosts for a nontechnical audience. These efforts, which were a common feature in many episodes, certainly bore fruit. Weinstein’s reach on Twitter grew from around 278,000 in May 2020 to over 735,000 by September 2022, with weekly follower gains accelerating rapidly in the early days of the pandemic.102 Heying’s reach also accelerated in parallel, from around 86,000 followers in May 2020 to more than 200,000 as of September 2022.103
Some podcasters also cited non-peer reviewed preprints and data, which often contained faulty statistical analysis or poor research designs, to bolster their prior beliefs (on the efficacy of ivermectin, for instance) while summarily dismissing similar non-peer reviewed studies whose conclusions ran contrary to their preferences (on the spread of COVID-19 as a result of the August 2020 Sturgis Motorcycle Rally, for example).104 Podcasters even cited “medRxiv” as a publisher, even though this website is not a peer-reviewed journal but rather a broadly available, free repository of preprinted manuscripts with limited vetting procedures.105 The website explicitly states, “Preprints . . . should not be relied on to guide clinical practice or health-related behavior and should not be reported in news media as established information.”106
In podcasting and elsewhere, a widespread reliance on preprints as evidence was one of the most pervasive trends of the pandemic. Recent research found a huge spike in the sharing of preprinted journal articles in the early stages of the pandemic, with the largest increase in preprint shares on social media coming from conservative users.107 These preprinted studies have also formed the basis of faulty policy, such as the dispersal of ivermectin in medical kits in Mexico.108 Although preprints play a vital role in disseminating research to audiences during what may normally be a lengthy peer review process, in times of scientific and medical uncertainty, they can be detrimental in shaping public opinion among audiences who may lack the credentials to critically evaluate each study on its technical merits.
Podcasters also often expressed frustration with the mixed and shifting messages from policymakers and public health officials. Attempts by policymakers to reassure an anxious public amid uncertainty by projecting a high degree of certainty were often met with backlash later, as the trajectory of the pandemic evolved.109 For example, in an interview with “60 Minutes” on March 8, 2020, Dr. Fauci tried to reassure the public about the severity of the emerging virus by saying, “there’s no reason to be walking around with a mask.”110 This interview predated the CDC’s updated April 3 recommendation, which mandated face coverings and masks in public settings as scientific understanding of the virus evolved. But later in the year, Fauci’s early remarks began to reappear across podcasts and on social media, as political commentators questioned the necessity of widespread masking.111 More broadly, shifting recommendations based on evolving scientific knowledge led some podcast hosts and guests to question the nature of scientific inquiry altogether. For example, a guest on Sarah Gonzales’s show, “The News and Why It Matters,” criticized scientists’ shifting assessment of hydroxychloroquine: “So the science changed. I thought science doesn’t change.”112
More broadly, classifying content as “false or misleading” during the pandemic – particularly when uncertainty was exceptionally high – proved to be a complicated and occasionally counterproductive exercise. What might have been considered true at one point (such as the claim that the Pfizer vaccine is 95% effective at preventing infection) might prove to be less accurate down the road, as knowledge accumulated or as new variants emerged.113 Yet the consequences of missing the mark or changing guidelines as the pandemic’s trajectory has shifted may be even more consequential, leading to an erosion of trust or a total rejection of scientific consensus.114 This was the case following a crackdown on the lab leak theory about the virus’s origins. Facebook removed content endorsing the theory and even permanently banned some users for perpetuating it.115 When further research failed to wholly rule out the possibility that the virus had lab-based origins, the social media platform backtracked on its previous ban. This incident may have damaged Facebook’s ability to prevent the spread of other types of claims that ran contrary to scientific consensus by leading some users to believe that the platform operated with a partisan agenda.116
As researchers from the nonprofit research institution Data & Society note, framing debates over ongoing scientific inquiry, such as hydroxychloroquine usage for coronavirus treatment, “as a battle between legitimate scientific information vs. dangerous misinformation” overlooks more nuanced discussions about the role of evidence in medical decision making and the amount required for medical professionals to make a recommendation in accordance with their oath to “do no harm.”117 This suggests lessons for fact checkers and social media companies working to create a safe information ecosystem in the face of an unprecedented flow of potentially deadly information, while simultaneously guarding against absolute policies in a fluid external environment.
Policy recommendations for the podcasting medium
In the aftermath of a once-in-a-generation pandemic and the violence that followed the 2020 elections, social media companies have grappled with how best to balance the necessities of content moderation with the importance of free expression.118 The spread of false and unsubstantiated claims, rumors, and other misleading content in the decentralized podcasting ecosystem poses a unique challenge. Unlike social media platforms, which directly host user-generated content, podcasts are hosted across a wide range of websites. Most podcasting apps, like Apple Podcasts or Google Podcasts, do not host this content. Instead, in the words of podcast commentator Nick Quah, they act more as “the impartial steward (and enforcer) of the open podcast ecosystem.”119 It is unclear where responsibility lies for podcast content. Due to the diffusion of responsibilities inherent to the ecosystem, improvements to the space will require the input of a range of actors – including tech companies, governments, and podcast hosts and listeners – who all help shape the quality of conversations across the medium.
The role of podcasting apps and tech companies
Since the early years of podcasting, apps have taken a hands-off approach to moderating content and have provided a sparse architecture to facilitate distribution. As the medium continues to evolve and expand, the tech companies that develop podcasting apps can help shape the information ecosystem in important ways by crafting more robust content moderation guidelines and practices, promoting greater transparency, and improving the in-app experience for users.
Content moderation
One aspect of technology companies’ role is transparency in content moderation. The major tech companies that develop podcasting apps – such as Apple, Spotify, and Google – until recently have avoided wading into content moderation debates across the podcasting ecosystem. Despite being the primary link between content creators and their audiences, these apps do not directly host the content themselves. Instead, they act more like search engines. However, rather than cataloging content across the web, they organize available content across a diverse network of RSS feeds unique to each podcast series. Podcasting apps have compared themselves to search engines to avoid a more active role in the ecosystem, but many popular search engines have also established guidelines for the content of their search results.
As the medium grows in popularity and based on the European Union’s (EU) recently enacted Digital Services Act (DSA) – which sets forth the contours of digital content moderation across the EU – podcasting apps may soon be forced to reckon with how they handle content that falls into the so-called “lawful-but-awful” domain, such as hate speech, misinformation, and targeted harassment.120 These policies will have to balance a desire to limit the real-world harm that can stem from the mass dissemination of objectionable content, with a vital necessity not to curtail speech too aggressively or inconsistently. To address these challenges, tech companies should:
- Provide more detailed, transparent guidelines for the content that podcasters can share on their apps. Podcasting apps tend to take a passive approach to content moderation, with minimal guidelines detailing what they will and will not share with listeners. Apple’s guidelines, for example, prohibit “defamatory, discriminatory, or mean-spirited” content but provide little explanation for how these terms are defined.121 Clearly delineated, consistently enforced guidelines would help podcasting apps make content moderation decisions more systematically. In addition, clearer guidelines would help podcasters understand what kinds of content may lead to episode removal across these platforms. In tandem with more transparent content moderation guidelines, podcast apps should provide a means for hosts to contest decisions based on these guidelines.
- Develop detailed, transparent guidelines for the content that apps will promote or recommend. In addition to guidelines detailing what content tech companies will host, some platforms have developed separate guidelines for detailing the types of content their recommendation algorithms will promote.122 Apart from word-of-mouth recommendations, users often discover new podcasts through in-app recommendation systems, like featured “most popular” lists or personalized recommendations.123 Podcasting apps like Spotify and Apple Podcasts should develop similarly detailed policies on the types of content they may choose not to amplify to their users via recommendation systems even if the apps still decide to host it.124
App architecture
Despite the growing influence of the medium, the architecture that underpins podcasting apps has not evolved significantly. As the podcasting ecosystem continues to grow, tech companies could consider updates to their podcasting architecture. Changes to the app ecosystem will not only create a better information environment across the industry by facilitating user-level participation but may also improve user experiences and foster greater in-app engagement. The tech companies that develop podcasting apps should:
- Improve reporting processes for individual podcast episodes. Podcast apps have not yet developed sophisticated, real-time systems to identify harmful content at scale. Without more robust investment in data-driven algorithms or in-house moderation teams, these apps must rely on user reports to identify content that should potentially be removed. Yet these systems can be poorly designed, difficult to find, or altogether absent across major podcasting apps. Until recently, Apple only allowed user reports focused on just four categories: “concern with a show or episode,” “broken feature,” “feature request,” or “other.” They have since expanded these categories to highlight specific concerns and distinguish between issues with an entire series or a specific episode. Other apps, however, have yet to make these adjustments. In the Google Podcasts app, for example, users can simply “send feedback” online, and Spotify provides no obvious means for users to report episodes or series. To leverage the proverbial wisdom of the crowd, podcast apps should include clear in-app icons that guide users through the reporting process and categories for different violations in accordance with content moderation guidelines.
- Design an app architecture that allows for richer community engagement and a more dynamic information environment. Podcasting apps could experiment with a variety of in-app features for these purposes, including voting and commenting systems and additional features at the episode level. With the development of open-source transcription models like OpenAI’s Whisper, adding transcripts to episodes has never been easier. Building on ad hoc, decentralized contributions from fans, users, and hosts could also be a way to add show notes and references to podcast episodes.125 Moreover, podcasting apps could experiment with upvoting and downvoting features for these contributions, drawing inspiration from community-driven websites, such as Reddit and Stack Overflow, or features like Twitter’s Community Notes, which offer additional crowd-sourced context on tweets.126 Although these approaches would need to guard against attempts to game the system (like voting restrictions for so-called serial voters or temporary bans on users who abuse certain features), they could help to build a more information-rich in-app experience.127 These efforts could also help to solve one of podcasting’s core challenges by transforming the ecosystem from a series of monologues, driven by hosts exclusively speaking to their audiences, into more of a series of conversations among listeners (and, perhaps, creators too).
The role of government
Regulators, policymakers, and other government officials also can help improve the podcast ecosystem. Doing so, however, requires that they strike a delicate balance between maintaining rights to freedom of expression and address well-documented harms like harassment and discrimination. In seeking to accomplish this, policymakers and regulators should neither seek to prohibit legal speech nor put pressure on podcast apps to ban content with which they disagree. Yet as is evident from the EU’s DSA and ongoing debates among U.S. policymakers, government officials can help push for greater transparency across the internet, including in the podcasting ecosystem.128 This is particularly true for apps with a large user base; the DSA, for instance, defines “very large online platforms” as those with an average of at least 45 million monthly active users. While it may be prohibitively cumbersome to press for oversight across the entire medium, for larger players in this space, policymakers should encourage greater transparency on:
- Content guidelines and policies. Regulators should require apps to clearly delineate their content moderation policies, with appropriate examples or clarifications for vague wording like “mean-spirited.” Without clearly defined, transparent guidelines, podcasting apps’ attempts at content moderation would likely appear ad hoc and undermine the trust of users.
- Moderation practices and an appeals process. Regulators should require podcasting apps to provide high-level public disclosures about content moderation practices. Policymakers should also make apps publish clear guidelines on how to dispute content moderation decisions (such as episode or series bans) and provide appellants with a transparent appeals process.
- Recommendation algorithms. Policymakers should require podcasting apps to provide high-level details about their recommendation algorithms and the process by which certain content is amplified over others. This is particularly important given the role that these algorithms play in helping listeners find new series.
- Podcaster funding. Despite the importance of advertisement and sponsorship revenue to the podcasting ecosystem, there are no obvious requirements for financial disclosures beyond those agreed upon between a given series and its sponsors. Although popular podcasting apps require ads to comply with “applicable laws,” it is unclear how apps determine if and when a violation has occurred.129 As a result, anyone could fund a podcast with a wide and influential reach without any required disclosure of this financial relationship. Much like financial disclosures in the radio industry or for social media influencers, regulators could help shed light on the nature of these financial relationships – and their opaque business models – by making podcasters report major streams of funding for their series.130 Due to the size of the ecosystem, these requirements might only be applied to shows that generate a certain amount of revenue or that have an audience above a certain threshold.
The role of users and podcasters
Along with tech companies and policymakers, podcast listeners and hosts also are instrumental in shaping the podcasting ecosystem. Although apps may build out new features to improve their infrastructure, podcasts still need users willing to contribute to the conversation. For popular shows with highly engaged fans, like the “Joe Rogan Experience,” this would likely be of little concern. However, less popular shows may draw fewer fan contributions, making the hosts of such podcasts critical partners in these efforts. To this end, podcasters could provide transcripts, highlight key episode topics and themes, or link to external references, for example. Apps could potentially reward creators for helping to create a richer information ecosystem by boosting their content.
Additionally, since podcast audiences often attribute a certain level of esteem to the hosts of their favorite series, popular podcasters should recognize their positions of authority and use this status wisely. It is critical that podcast hosts qualify their statements with the appropriate level of uncertainty, challenge guests who fail to do the same, and bolster seemingly factual statements with evidence, perhaps by sharing relevant links. An example of this dynamic was on display during a September 2022 episode of “The Joe Rogan Experience,” when Rogan was fact-checked in real time, both by a guest and later by external sourcing.131
For unsubstantiated and false health-related claims, it is critical for podcasters, and all media figures, to recognize the limitations of their expertise and refrain from sharing research and resources that have not gone through rigorous peer review, unless they wholly understand these papers’ methods and statistical processes (along with their shortcomings and assumptions). This phenomenon is not unique to one end of the partisan spectrum or to podcasting as a medium. Yet sharing preprints that have not withstood peer review may pose significant costs to the country’s public health response to the pandemic (or some future crisis) and may even lead people to make decisions that put their lives at risk or cause hysteria that ignores scientific consensus.
The future of podcasting
Podcasts are only beginning to shape public opinion and political debates in vital ways. How the industry evolves from here has implications for the tasks and responsibilities of tech companies, regulators, listeners, and hosts. These shifts will also factor prominently in the diffusion of unsubstantiated or false content.
Spotify’s model of exclusive content relationships built around a single, larger platform represents one such avenue for expansion. In this model, podcast apps in many ways have transformed from merely distributors to both distributors and publishers.132 As podcasting becomes even more popular, it is possible that the industry could move further toward the Spotify model. In many ways, this could make content moderation challenges more straightforward by eliminating issues with information diffusion across numerous platforms and consolidating responsibility for content creation (and moderation) into the hands of a single (or a few) publisher(s).
Other platforms are also expanding into podcasting with a model that involves sharing exclusive content with subscribers, often for a small fee. Substack, a digital newsletter platform, has recently entered the podcasting sector as a rival to Patreon, a platform that allows creators to provide subscribers with additional paywalled content.133 In both cases, putting exclusive content behind a paywall would make monitoring the medium more difficult, but it may also restrict the unfettered reach (and potential accidental discovery) of lawful-but-awful content to only those who are willing to pay for it directly.
Despite these clear shifts designed to bring more revenue to podcasting, it is still unlikely that the medium will stop being decentralized entirely. For most podcast series, openness will likely remain a central, if not defining, feature. As a result, many of the challenges detailed throughout this report will probably remain relevant.
Until recently, the spread of unsubstantiated or false content throughout popular political podcasts has been underexplored due to both technical limitations and misconceptions of the medium. However, it is not uncommon for these types of claims to proliferate across popular political podcasts – affecting more than one out of every 20 episodes and nearly three-quarters of all podcast series examined. Given the conservative research approach in this report, the findings detailed here likely represent the lowest possible floor for the proliferation of unsubstantiated or false content in popular political podcasts. Moving forward, it will be critical for researchers to continue to monitor this medium, particularly as podcasting becomes even more influential in shaping public discourse.
Unlike the scrutiny that social media platforms have received for spreading misleading content, the similar role played by political podcasts at pivotal moments during the 2020 election campaign and the pandemic has gone largely undetected, despite their potential to spread unsubstantiated or even false claims and contribute to real-world harms. Incorporating podcasting into these ongoing debates will go a long way toward improving this increasingly important information environment. Tech companies, regulators, users, and researchers all have roles to play.
DOWNLOAD:
Endnotes
- 1. Verdict With Ted Cruz, “Live From Miami at YAF’s Freedom Conference, Ep. 65,” YouTube, February 11, 2021, https://www.youtube.com/watch?v=0Hg1ABSg4L8.
- 2. Andrew J. Bottomley, “Podcasting: a decade in the life of a ‘new’ audio medium: introduction,” Journal of Radio and Audio Media 22, no. 2 (July 2015): 164–169.
- 3. “Audio and podcasting fact sheet,” Pew Research Center, June 29, 2021, https://www.pewresearch.org/journalism/fact-sheet/audio-and-podcasting/; and Mason Walker, “Nearly a Quarter of Americans Get News from Podcasts,” Pew Research Center, February 15, 2022, https://www.pewresearch.org/fact-tank/2022/02/15/nearly-a-quarter-of-americans-get-news-from-podcasts/.
- 4. “Number of Podcast Listeners Worldwide 2024,” Statista, https://www.statista.com/statistics/1291360/podcast-listeners-worldwide/?utm_campaign=adtech.podnews.net%3A2022-07-19&utm_medium=email&utm_source=adtech.podnews.net; and “U.S. Podcast Advertising Revenue Report: FY 2021 Results & 2022-2024 Growth Projections,” IAB, May 9, 2022, https://www.iab.com/insights/u-s-podcast-advertising-revenue-report-fy-2021-results-2022-2024-growth-projections/.
- 5. “Unsubstantiated and false claims” include either claims not backed by evidence at the time they were shared or those that are wholly false. Shannon Bond, “How Alex Jones Helped Mainstream Conspiracy Theories Become Part of American Life,” NPR, August 6, 2022, https://www.npr.org/2022/08/06/1115936712/how-alex-jones-helped-mainstream-conspiracy-theories-into-american-life; Dominik Stecula and Matt Motta, “Analysis: Joe Rogan Told His Millions of Listeners Not to Take His Anti-Vaccine Advice Seriously. Is It Too Late?,” The Washington Post, May 3, 2021, https://www.washingtonpost.com/politics/2021/05/03/joe-rogan-told-his-millions-listeners-not-take-his-anti-vaccine-advice-seriously-is-it-too-late/; and Grace Dean, “Joe Rogan Said on His Podcast That Healthy Young People Should Avoid COVID-19 Vaccines. Spotify Reportedly Has No Plans to Remove the Episode,” Business Insider, April 28, 2021, https://www.businessinsider.com/joe-rogan-experience-spotify-podcast-covid-vaccine-coronavirus-children-2021-4.
- 6. Bottomley, “Podcasting: a decade in the life of a ‘new’ audio medium: introduction”; and Valerie Wirtschafter, “The challenge of detecting misinformation in podcasting,” The Brookings Institution, August 25, 2021, http://www.brookings.edu/techstream/the-challenge-of-detecting-misinformation-in-podcasting/.
- 7. Unless otherwise noted, the figures and statistics about unsubstantiated or false claims made in podcasts are taken from my study of the set of podcast episodes as described in this report. See the methodology section and the appendixes for more information.
- 8. Data on Twitter followers was manually collected from podcast hosts’ Twitter profiles. Data on Facebook followers was manually collected from CrowdTangle. CrowdTangle Team (2020). CrowdTangle. Facebook, Menlo Park, California, United States, 1725781.
- 9. Ben Hammersley, “Audible Revolution,” The Guardian, February 12, 2004, https://www.theguardian.com/media/2004/feb/12/broadcasting.digitalmedia.
- 10. Ibid.
- 11. Ibid.
- 12. Richard Berry, “Will the iPod kill the radio star? profiling podcasting as radio,” Convergence: The International Journal of Research Into New Media Technologies 12, no. 2 (May 2006): 143–162, https://doi.org/10.1177/1354856506066522.
- 13. For more background on the RSS feed, see Appendix 1.
- 14. Hammersley, “Audible Revolution.”
- 15. Alex Thompson, “Podcasts Are Becoming the Left’s Right-Wing Talk Radio,” Vice, August 31, 2017, https://www.vice.com/en/article/a3jadk/podcasts-are-becoming-the-lefts-right-wing-talk-radio.
- 16. Ibid.
- 17. “How a new generation of left-wing podcasters are dethroning Rush Limbaugh and right-wing talk radio,” The Week, September 13, 2017, https://theweek.com/articles/723980/how-new-generation-leftwing-podcasters-are-dethroning-rush-limbaugh-rightwing-talk-radio.
- 18. See “Top 100 US Podcasts (Apple Podcasts Top Charts),” Podcast Insights, https://www.podcastinsights.com/top-us-podcasts/.
- 19. Rebecca Iannucci, “News or Opinion? Online, It’s Hard to Tell,” Poynter, August 16, 2017, https://www.poynter.org/ethics-trust/2017/news-or-opinion-online-its-hard-to-tell/.
- 20. For more on new media’s role in expanding the scope of who can “be a media outlet,” see Clay Shirky, Here Comes Everybody: The Power of Organizing Without Organizations (New York: Penguin, 2008), 55.
- 21. Twitter, “Community Notes Guide,” https://twitter.github.io/communitynotes/overview/.
- 22. Richard Berry, “Podcasting: considering the evolution of the medium and its association with the word ‘radio,’” Radio Journal: International Studies in Broadcast and Audio Media 14, no. 1 (2016): 7–22.
- 23. Andrew M. Guess, Brendan Nyhan, and Jason Reifler, “Exposure to untrustworthy websites in the 2016 US election,” Nature Human Behaviour 4, no. 5 (May 2020): 472–480, https://doi.org/10.1038/s41562-020-0833-x.; Nir Grinberg, Kenneth Joseph, Lisa Friedland, Briony Swire-Thompson, and David Lazer, “Fake news on Twitter during the 2016 US presidential election,” Science 363, no. 6425 (2019): 374–378; and Mathias Osmundsen, Alexander Bor, Peter Bjerregaard Vahlstrup, Anja Bechmann, and Michael Bang Petersen, “Partisan polarization is the primary psychological motivation behind political fake news sharing on Twitter,” American Political Science Review 115, no. 3 (2021): 999–1015.
- 24. Alec Radford, “Introducing Whisper,” OpenAI, September 22, 2022, https://openai.com/blog/whisper/.
- 25. Berry, “Podcasting”.
- 26. James Cridland, “How Many Podcasts Are There?” Podnews, March 10, 2022, https://podnews.net/article/how-many-podcasts.
- 27. Jelte M. Wicherts, Coosje LS Veldkamp, Hilde EM Augusteijn, Marjan Bakker, Robbie Van Aert, and Marcel ALM Van Assen, “Degrees of freedom in planning, running, analyzing, and reporting psychological studies: A checklist to avoid p-hacking,” Frontiers in Psychology (2016): 1,832.
- 28. For apps that rely on RSS feeds exclusively, Apple Podcasts claims the highest market share of podcast listeners. As a result, despite preferencing iPhone users, Apple’s Podcasts’ top 100 lists form the basis for the series selection. Despite its popularity, The Joe Rogan Experience is not included in the data because it currently is a Spotify exclusive series and not distributed via public RSS feed; Brad Adgate, “2022 was another record Year for podcasts,” Forbes, January 25, 2023, https://www.forbes.com/sites/bradadgate/2023/01/25/2022-was-another-record-year-for-podcasts/.
- 29. The decision to use the “you-might-also-like” feature to expand the sample of episodes was designed to simulate how users might find new series, in a process often driven by the recommendation systems of podcasting apps.
- 30. On average, Azure reported an accuracy rate of 75% to 80% in their transcriptions, with most errors attributed to the spelling of proper nouns like names or locations. Spiceworks, “Top 10 Speech Recognition Software and Platforms in 2022,” Spiceworks, https://www.spiceworks.com/tech/artificial-intelligence/articles/speech-recognition-software/.
- 31. “Captions: YouTube Data API.” Google Developers, https://developers.google.com/youtube/v3/docs/captions.
- 32. To identify the political ideology of a show, I relied on a method that used choices about who users follow on Twitter as a measure of political ideology. More details about this method can be found in Pablo Barberá, “Birds of the same feather tweet together: Bayesian ideal point estimation using Twitter data,” Political Analysis 23, no. 1, (2015): 76–91. The R package to implement this process is available here: https://github.com/pablobarbera/twitter_ideology. The underlying assumption is that Twitter users often follow accounts that reflect their political interest, which can then be used to develop a measure of their political ideology. In cases when I was unable to identify a host’s political ideology based on this method (because the host doesn’t have a Twitter account or he or she follow too many or too few people for the information to be meaningful), I used either the figure’s known partisan affiliation or classified the show host’s ideology as “unknown.” See Appendix 2 for a breakdown of shows by partisan leaning.
- 33. For series with multiple hosts, I used the average partisan affiliation of all hosts that had available data.
- 34. This calculation assumes that hosts speak at a speed of 130 words per minute.
- 35. Figure 2 does not plot data for January 2022, since data collection ended on the 22nd, prior to the end of the month. These episodes, however, are included in the analysis and all subsequent figures.
- 36. “Twitter by the Numbers (2022): Stats, Demographics and Fun Facts,” Omnicor Agency, February 22, 2022, https://www.omnicoreagency.com/twitter-statistics/.
- 37. See endnote 8 for details on social media follower data collection.
- 38. C.J. Hutto and E.E. Gilbert, “VADER: A parsimonious rule-based model for sentiment analysis of social media text,” Eighth International Conference on Weblogs and Social Media, Ann Arbor, Michigan, June 2014.
- 39. The “non-election politics” category included politically motivated claims that targeted individual politicians or political parties as opposed to policies or issues. These included claims that former president Barack Obama is a socialist, that Senator Bernie Sanders is a Communist, and that Trump bullied a child at an Easter Egg roll, among other more targeted claims. “Election-related claims” focused primarily on campaign falsehoods, election fraud, and downplaying the January 6, 2020, Capitol attack. Abortion, healthcare, and COVID-19 were notable themes found in the “health/science” category. Meanwhile, claims in the “crime/guns” category focused on discussions about gun procurement laws, violence, and defunding the police.
- 40. For PolitiFact, I used claims rated as “pants on fire,” “false,” or “mostly false.” These classifications are described by PolitiFact as follows: (1) mostly false – “The statement contains an element of truth but ignores critical facts that would give a different impression”; (2) False – “The statement is not accurate”; and (3) Pants on Fire – “The statement is not accurate and makes a ridiculous claim.” See Angle Drobnic Holan, “The Principles of the Truth-O-Meter: PolitiFact’s Methodology for Independent Fact-Checking,” PolitiFact, February 12, 2018, https://www.politifact.com/article/2018/feb/12/principles-truth-o-meter-politifacts-methodology-i/. For Snopes, I used only fact checks categorized as “false,” “mostly false” or “scam.”
- 41. Jiawei Han, Micheline Kamber, and Jian Pei, “Getting to Know Your Data,” in Data Mining: Volume 2, (Boston: Morgan Kaufmann, 2012), 39–82. This strategy builds on research conducted with coauthors and detailed in the following working paper: Frederico Pereira et al., “Detecting misinformation: The spread of false news by political leaders in the Global South,” 2022.
- 42. The challenge with this method for assessing podcasts was that the documents were extremely unequal in length, which made vector comparisons difficult. On average, the fact-checked claims were 17 words, whereas the transcripts were 9,734 words. As a result, I devised a strategy to scan through the entire transcript using text windows at a length that was 1.3 times the length of a fact-checked claim. I used a slightly expanded window to allow for similar but not identical texts to be identified in the transcripts. Essentially, the strategy created a moving window across the transcript and calculated the cosine similarity across different windows of a transcripted text until it reached the end of the episode.
- 43. David Mikkelson, “SharpieGate: Were Trump Ballots Marked With Sharpies Disqualified in Arizona?,” Snopes, November 4, 2020, https://www.snopes.com/fact-check/sharpie-trump-ballots-arizona; and Samantha Putterman, “Video of a microchip reader finding a chip in a vaccinated woman’s arm was posted as a joke,” PolitiFact, July 1, 2021, https://www.politifact.com/factchecks/2021/jul/01/instagram-posts/video-microchip-reader-finding-chip-vaccinated-wom. See Appendix 3 for details on the dictionary of terms.
- 44. Michael Savage, “‘Trump’s Legacy and Why the Left Wing Gang Is Out to Crucify Him,’” The Michael Savage Show,” The Savage Nation, Friday July 2, 2021, http://michaelsavage.com/trumps-legacy-why-the-left-wing-gang-is-out-to-crucify-him-the-michael-savage-show-friday-july-2-2021/; and Michael Savage, “Savage Archives: Will Impeachment Cause a Rise of Antisemitism in the Country?,” The Savage Nation, January 22, 2020, https://michaelsavage.com/savage-will-impeachment-cause-a-rise-of-anti-semitism-in-the-country.
- 45. Ibid.
- 46. Ibid.
- 47. “The Top Talk Radio Audiences,” Talkers Magazine, November 14, 2006, https://web.archive.org/web/20061114200439/http://www.talkers.com/main/index.php?option=com_content&task=view&id=17&Itemid=34.; Sarah Sobieraj and Jeffrey M. Berry, “From incivility to outrage: Political discourse in blogs, talk radio, and cable news,” Political Communication 28, no. 1 (2011): 19–41.
- 48. Andrew Guess, Jonathan Nagler, and Joshua Tucker, “Less than you think: Prevalence and predictors of fake news dissemination on Facebook,” Science advances 5, no. 1 (2019); Nir Grinberg, Nir, Kenneth Joseph, Lisa Friedland, Briony Swire-Thompson, and David Lazer, “Fake news on Twitter during the 2016 US presidential election,” Science 363, no. 6425 (2019): 374-378; and Jennifer Allen, Baird Howland, Markus Mobius, David Rothschild, and Duncan J. Watts, “Evaluating the fake news problem at the scale of the information ecosystem,” Science advances 6, no. 14 (2020).
- 49. See endnote 8 for details on social media follower data collection.
- 50. Chloe Lim, “Checking how fact-checkers check,” Research and Politics 5, no. 3 (2018): 1–7; Sakari Nieminen and Valtteri Sankari, “Checking PolitiFact’s fact-checks,” Journalism Studies 22, no. 3 (2021): 358–378; Dallas Card, Lucy H. Lin, and Noah A. Smith, “PolitiFact language audit,” working paper, 2018; Charles Louis-Sidois, “Both judge and party? An analysis of the political leaning of fact-checkers,” Social Science Research Network (2022); and R. Kelly Garrett, and Robert M. Bond, “Conservatives’ susceptibility to political misperceptions,” Science Advances 7, no. 23 (2021).
- 51. To identify the partisan affiliation of the target of a fact check, two coders researched the individuals’ partisan ties based on news articles and other online sources.
- 52. Glenn Kessler, Salvador Rizzo, and Meg Kelly, “Trump’s False or Misleading Claims Total 30,573 Over 4 Years,” The Washington Post, January 24, 2021, https://www.washingtonpost.com/politics/2021/01/24/trumps-false-or-misleading-claims-total-30573-over-four-years/.
- 53. Hutto and Gilbert, “VADER: A parsimonious rule-based model for sentiment analysis of social media text.”
- 54. Steve Bannon, “EP 635- Pandemic: For The Republic (w/ Brian Kennedy, Ben Bergquam, John Fredericks, and Phil Klein),” Bannon’s War Room, January 6, 2021. https://warroom.org/2021/01/06/ep-635-pandemic-w-brian-kennedy-ben-bergquam-john-fredericks-and-phil-klein/.; A version of this analysis was featured in research posted on Brookings’ TechStream blog, but has been updated to include additional episodes that I was able to collect after the initial publication of the research and episodes from other podcasters who were not in Apple’s Top 100 at the time of the 2020 election but are analyzed throughout this report: Valerie Wirtschafter and Chris Meserole, “Prominent political podcasters played a key role in spreading the Big Lie,” Brookings Institution, January 6, 2022, http://www.brookings.edu/techstream/prominent-political-podcasters-played-key-role-in-spreading-the-big-lie/.
- 55. Andrew C. Eggers et al. “No Evidence for Systematic Voter Fraud: A Guide to Statistical Claims about the 2020 Election.” Proceedings of the National Academy of Sciences 118, no. 45 (November 2021); Saranac Hale Spencer, “Nine election fraud claims, none credible,” FactCheck.org, December 11, 2020, https://www.factcheck.org/2020/12/nine-election-fraud-claims-none-credible; and also see Wirtschafter and Meserole (2022).
- 56. “The Carter Center Releases ‘The Big Lie and Big Tech,’” The Carter Center, October 6, 2021, https://www.cartercenter.org/news/pr/2021/democracy-big-lie-big-tech-report-100621.html; Shannon Bond and Bobby Allyn, “How the ‘Stop the Steal’ Movement Outwitted Facebook Ahead of the Jan. 6 Insurrection,” NPR, October 22, 2021, https://www.npr.org/2021/10/22/1048543513/facebook-groups-jan-6-insurrection; Steven Levy, “A Trump Ban Is Easy. Fixing Facebook and Twitter Will Be Hard,” Wired, January 8, 2021, https://www.wired.com/story/plaintext-trump-ban-easy-fixing-facebook-twitter-hard/; Gowri Ramachandran, “Twitter Is a Cauldron of Misinformation About the Arizona 2020 Vote Audit,” Slate, May 14, 2021, https://slate.com/technology/2021/05/maricopa-county-arizona-2020-vote-recount-misinformation.html; Yaël Eisenstat, “How to Hold Social Media Accountable for Undermining Democracy,” Harvard Business Review, January 11, 2021. https://hbr.org/2021/01/how-to-hold-social-media-accountable-for-undermining-democracy; Craig Silverman, Ryan Mac, and Jane Lytvynenko, “Facebook Knows It Was Used To Help Incite The Capitol Insurrection,” Buzzfeed News, April 22, 2021, https://www.buzzfeednews.com/article/craigsilverman/facebook-failed-stop-the-steal-insurrection; and Nate Rattner, “Trump’s Election Lies Were Among His Most Popular Tweets,” CNBC, January 13, 2021, https://www.cnbc.com/2021/01/13/trump-tweets-legacy-of-lies-misinformation-distrust.html.
- 57. These numbers include additional episode data I was able to collect after the publication of Wirtschafter and Meserole (2022).
- 58. Adrian Blanco et al. “Tracking Which 2020 Election Deniers Are Winning, Losing in the Midterms,” The Washington Post, https://www.washingtonpost.com/politics/interactive/2022/election-deniers-midterms/; and Danielle Kurtzleben, “Trump Announces 2024 Presidential Run,” NPR, November 15, 2022, https://www.npr.org/2022/11/15/1137052704/trump-2024-president-campaign.
- 59. For background on the origins of the term “The Big Lie” in an election-related context, see: Melissa Block, “The clear and present danger of Trump’s enduring ‘Big Lie’”, NPR, December 23, 2021, https://www.npr.org/2021/12/23/1065277246/trump-big-lie-jan-6-election.
- 60. Rachel Orey, “Key December Dates for the Electoral College,” Bipartisan Policy Center, December 2, 2020, https://bipartisanpolicy.org/blog/december-electoral-dates/; and Nina Totenberg and Barbara Sprunt, “Supreme Court Shuts Door on Texas Suit Seeking to Overturn Election,” NPR, December 11, 2020, https://www.npr.org/2020/12/11/945617913/supreme-court-shuts-door-on-trump-election-prospects.
- 61. ”Supporting the 2020 U.S. Election.” YouTube Official Blog, December 9, 2020. Accessed October 17, 2022. https://blog.youtube/news-and-events/supporting-the-2020-us-election/.
- 62. Bond and Allyn, “How the ‘Stop the Steal’ Movement Outwitted Facebook Ahead of the Jan. 6 Insurrection.”
- 63. Sheera Frenkel, “The Rise and Fall of the ‘Stop the Steal’ Facebook Group,” The New York Times, November 5, 2020, https://www.nytimes.com/2020/11/05/technology/stop-the-steal-facebook-group.html.
- 64. Neil Vigdor, ”Twitter Flags Posts by Trump That Made Premature Claims of Victory or Baseless Ones About Election Fraud,” The New York Times, November 4, 2020, https://www.nytimes.com/2020/11/04/us/politics/trump-twitter-labels.html; and Vijaya Gadde and Kayvon Beykpour, “An Update on Our Work Around the 2020 US Elections,” November 12, 2020, https://blog.twitter.com/en_us/topics/company/2020/2020-election-update.
- 65. Helen Sullivan, “‘Whoa’ – Fox News Cuts Off Kayleigh McEnany for ‘Illegal Votes’ Spiel,” The Guardian, November 9, 2020, https://www.theguardian.com/us-news/2020/nov/10/whoa-fox-news-cuts-off-kayleigh-mcenany-for-votes-spiel; and Tom McCarthy, “Rupert Murdoch-Owned US Outlets Turn on Trump, Urging Him to Act With ‘Grace,’” The Guardian, November 7, 2020, https://www.theguardian.com/us-news/2020/nov/07/rupert-murdoch-owned-us-outlets-turn-on-trump-urging-him-to-concede-with-grace.
- 66. “Smartmatic Demands Justice for Defamation.” Smartmatic, December 14, 2020, https://www.smartmatic.com/us/media/article/smartmatic-demands-justice-for-defamation/; and Kevin Breuninger, “Election Tech Company Smartmatic Demands Retractions From Fox, Newsmax, OAN Over Conspiracy Theories,” CNBC, December 14, 2020, https://www.cnbc.com/2020/12/14/smartmatic-demands-retractions-from-fox-newsmax-oan.html.
- 67. Sean Illing, “‘Flood the Zone with Shit’: How Misinformation Overwhelmed Our Democracy,” Vox, January 16, 2020, https://www.vox.com/policy-and-politics/2020/1/16/20991816/impeachment-trial-trump-bannon-misinformation.
- 68. Steve Bannon, “Ep 544 – Pandemic: Weather the Storm (w/ Jack Posobiec, Rudy Giuliani, Col. Doug Mastriano, and Raymond Ibrahim),” Bannon’s War Room, November 28, 2020, https://warroom.org/2020/11/28/ep-544-pandemic; Sean Hannity, “Fraud in North Carolina,” The Sean Hannity Show (Apple Podcasts), https://podcasts.apple.com/us/podcast/fraud-in-north-carolina/id1112194905?i=1000498555808; Michael Knowles, “What’s Kraken? , Ep. 650,” YouTube, November 23, 2020, https://www.youtube.com/watch?v=Jwj5g-CwOhw. https://www.youtube.com/watch?v=Jwj5g-CwOhw; and The Megyn Kelly Show, “Dave Rubin on Voting For Trump, Gay Marriage and COVID Hypocrisy,” YouTube, November 28, 2020, https://www.youtube.com/watch?v=JBNwFbUcYh4.
- 69. Michael Knowles, “Schrödinger’s POTUS (And Joe Biden’s Cat), Ep. 653,” YouTube, November 30, 2020. https://www.youtube.com/watch?v=QKcHeiOpOjk; “The Rush Limbaugh Show Podcast – Nov 20 2020,” The Clay Travis and Buck Sexton Show (IHeart), November 20, 2020, https://www.iheart.com/podcast/1119-travis-and-sexton-57927691/episode/the-rush-limbaugh-show-podcast–74229834/; “The Holiday Leftovers You Can’t Ignore | Guest: Justin Barclay,” The Glenn Beck Program (Apple Podcasts), https://podcasts.apple.com/qa/podcast/the-holiday-leftovers-you-cant-ignore-guest-justin-barclay/id620967489?i=1000500504374; and Michael Knowles, “How to Steal an Election, Ep. 641,” YouTube, November 5, 2020, https://www.youtube.com/watch?v=fiIl8iFoSbw.
- 70. A feature on Bannon’s website that purported to track downloads has now been disabled, but as of March 2022, the number stood at 135 million downloads. Jessica Brandt, Valerie Wirtschafter, and Adya Danaditya, “Popular podcasters spread Russian disinformation about Ukraine biolabs,” The Brookings Institution, March 23, 2022, http://www.brookings.edu/techstream/popular-podcasters-spread-russian-disinformation-about-ukraine-biolabs/.
- 71. David M. Drucker, In Trump’s Shadow: The Battle for 2024 and the Future of the GOP (New York: Grand Central Publishing, 2021), https://www.google.com/books/edition/In_Trump_s_Shadow/jpgTEAAAQBAJ?hl=en&gbpv=1&dq=how+many+downloads+per+show+for+ben+shapiro&pg=PT135&printsec=frontcover.
- 72. See endnote 8 for details on social media follower data collection.
- 73. Glenn Beck, “Bernie’s Communist Revolution Is Coming | Guest: Giancarlo Sopo | 2/24/20,” The Glenn Beck Program (Apple Podcasts), https://podcasts.apple.com/us/podcast/bernies-communist-revolution-is-coming-guest-giancarlo/id620967489?i=1000466539909.
- 74. Daniel Horowitz, “Ep 643 | The Coronavirus Distortions Are a National Scandal,” Conservative Review With Daniel Horowitz (Apple Podcasts), https://podcasts.apple.com/us/podcast/ep-643-the-coronavirus-distortions-are-a-national-scandal/id1065050908?i=1000475232258;
- 75. John Solomon, “Biomedical statistician challenges narrative on benefits of social distancing,” John Solomon Reports (Apple Podcasts), https://podcasts.apple.com/us/podcast/biomedical-statistician-challenges-narrative-on-benefits/id1495251160?i=1000471473047.
- 76. Adam M. Enders, Joseph E. Uscinski, Casey Klofstad, and Justin Stoler, “The Different Forms of COVID-19 Misinformation and Their Consequences,” The Harvard Kennedy School Misinformation Review, November 2020, https://dash.harvard.edu/handle/1/37366466; and Gaurav Verma, Ankur Bhardwaj, Talayeh Aledavood, Munmun De Choudhury, and Srijan Kumar, “Examining the impact of sharing COVID-19 misinformation online on mental health,” Scientific Reports 12, no. 1 (May 16, 2022): https://doi.org/10.1038/s41598-022-11488-y.
- 77. “Health Misinformation — Current Priorities of the U.S. Surgeon General,” Department of Health and Human Services, https://www.hhs.gov/surgeongeneral/priorities/health-misinformation.
- 78. Raina M. Merchant and Nicole Lurie, “Social media and emergency preparedness in response to novel coronavirus,” Journal of the American Medical Association, March 23, 2020, https://jamanetwork.com/journals/jama/fullarticle/2763596; Matteo Cinelli et al. “The COVID-19 social media infodemic,” Scientific Reports 10, no. 1 (2020): https://ncbi.nlm.nih.gov/pmc/articles/PMC7538912/; Joan Donovan, “Here’s How Social Media Can Combat the Coronavirus ‘Infodemic,’” MIT Technology Review, March 17, 2020, https://www.technologyreview.com/2020/03/17/905279/facebook-twitter-social-media-infodemic-misinformation/; and Jon-Patrick Allem, “Social Media Fuels Wave of Coronavirus Misinformation as Users Focus on Popularity, Not Accuracy,” The Conversation, April 6, 2020, https://theconversation.com/social-media-fuels-wave-of-coronavirus-misinformation-as-users-focus-on-popularity-not-accuracy-135179.
- 79. Tiffany Hsu and Marc Tracy, “On Podcasts and Radio, Misleading Covid-19 Talk Goes Unchecked,” The New York Times, November 12, 2021, https://www.nytimes.com/2021/11/12/business/media/coronavirus-misinformation-radio-podcasts.html.
- 80. Shannon Bond, ”What the Joe Rogan Podcast Controversy Says About the Online Misinformation Ecosystem,” NPR, January 21, 2022, https://www.npr.org/2022/01/21/1074442185/joe-rogan-doctor-covid-podcast-spotify-misinformation. A recent report by the Tech Transparency Project examined false claims tied to COVID-19 and monkeypox in podcasting but evaluated episodes featuring some of the most severe spreaders of false content. See “COVID Misinformation, Dangerous Treatments Thrive on Apple Podcasts,” Washington, DC: Campaign for Accountability’s Tech Transparency Project, https://www.techtransparencyproject.org/sites/default/files/Apple-Podcast-Report.pdf.
- 81. Michelle L. Holshue et al. “First Case of 2019 Novel Coronavirus in the United States,” New England Journal of Medicine 382, no. 10 (March 2020): 929–936, https://doi.org/10.1056/NEJMoa2001191.
- 82. “Infodemic,” World Health Organization, https://www.who.int/health-topics/infodemic#tab=tab_1; and “The COVID-19 infodemic,” The Lancet 20, no. 8 (July 2020), https://www.thelancet.com/journals/laninf/article/PIIS1473-3099(20)30565-X/fulltext.
- 83. See endnote 8 for details on social media follower data collection.
- 84. For this section, two coders also classified unsubstantiated or false claims into one of six different categories: (1) alternative treatments/prevention (such as the notion that hydroxychloroquine prevents COVID, after the drug’s EUA was revoked in June 2020); (2) conspiratorial claims (such as the idea that the vaccine is a bioweapon that was intentionally released); (3) vaccine efficacy/side effects (like the claim that the vaccine kills more people than COVID); (4) disease severity (such as the notion that COVID is no more dangerous than a cold, after lockdowns began across the United States in mid-March 2020); (5) the government policy/response (including the idea that the government would put unvaccinated people into internment camps); and (6) testing (including the claim that COVID testing can’t tell the difference between COVID and the flu).
- 85. Libby Cathey, “Timeline: Tracking Trump Alongside Scientific Developments on Hydroxychloroquine,” ABC News, August 8, 2020, https://abcnews.go.com/Health/timeline-tracking-trump-alongside-scientific-developments-hydroxychloroquine/story?id=72170553.
- 86. Ibid.
- 87. Ibid.
- 88. Charlie Kirk, “The Charlie Kirk Show: 3 Doctors Speak Out — The Update You Have Been Waiting For,” YouTube, August 28, 2020, https://youtu.be/Amp4mbm2UIA?t=3352.
- 89. Andrea Michaelson and Kieran Press-Reynolds, “Spotify Airs Joe Rogan Podcast Touting Ivermectin as Part of His COVID-19 Treatment, Despite the FDA Calling It ‘Dangerous,’” Business Insider, September 8, 2021, https://www.businessinsider.com/joe-rogan-experience-ivermectin-spotify-covid19-treatment-19-2021-9.
- 90. “Rapid Increase in Ivermectin Prescriptions and Reports of Severe Illness Associated With Use of Products Containing Ivermectin to Prevent or Treat COVID-19,” Centers for Disease Control and Prevention, August 26, 2021, https://emergency.cdc.gov/han/2021/pdf/CDC_HAN_449.pdf.
- 91. Daniel Funke, “PolitiFact – Who Are the Doctors in the Viral Hydroxychloroquine Video?” PolitiFact, July 29, 2020, https://www.politifact.com/article/2020/jul/29/who-are-doctors-viral-hydroxychloroquine-video/.
- 92. Madison Dapcevich, “Who Are ‘America’s Frontline Doctors’ and Dr. Stella Immanuel?,” Snopes, July 30, 2020, https://www.snopes.com/news/2020/07/30/americas-frontline-doctors/; and “America’s Frontline Doctors Scotus Press Conference Transcript,” Rev, July 28, 2020, https://www.rev.com/blog/transcripts/americas-frontline-doctors-scotus-press-conference-transcript.
- 93. Mark Levin, “Mark Levin Audio Rewind – 7/28/20,” Mark Levin Podcast (Audacy), July 29, 2020, https://www.audacy.com/podcasts/mark-levin-podcast-41762/mark-levin-audio-rewind-72820-320221775.
- 94. Steve Bannon, “Episode 1,040 – The Daszak Files (w/ Natalie Winters, Dr. Peter Navarro),” Bannon’s War Room, June 22, 2021, https://warroom.org/episode-1040-the-daszak-files-w-natalie-winters-dr-peter-navarro; and Steve Bannon “Episode 1,004 – War-Time Footing . . . How MAGA Candidates and the America First Agenda Will Save the USA,” Bannon’s War Room, June 7, 2021, https://warroom.org/2021/06/07/episode-1004-war-time-footing-how-maga-candidates-and-the-america-first-agenda-will-save-the-usa/.
- 95. Bret Weinstein, “Covid: The Path Not Taken – DarkHorse Podcast With Dr. Peter McCullough,” YouTube, December 6, 2021, https://youtu.be/-zg1j7Zquoc?t=1574.
- 96. Charlie Kirk, “MRNA, Microsoft, and the Mark of the Beast? COVID Thought Crimes With Dr. Vladimir Zelenko,” The Charlie Kirk Show, (Omny.fm), December 17, 2021, https://omny.fm/shows/the-charlie-kirk-show/mrna-microsoft-and-the-mark-of-the-beast-covid-tho?t=25m16s.
- 97. Steve Bannon, “Episode 1,081 – We are Watching the ’Great Realignment’ (w/ Joe Mobley, Renee, Jack Posobiec),” Bannon’s War Room, July 8, 2021. https://warroom.org/2021/07/08/episode-1081-we-are-watching-the-great-realignment/.
- 98. Steve Bannon, “Episode 1,430 – Waukesha Terrorism And Austria Totalitarianism (w/ Ben Harnwell, Matthew Tyrmand, Naomi Wolf, Debora Blake, Peter Navarro),” Bannon’s War Room, November 22, 2021, https://warroom.org/2021/11/22/episode-1430-waukesha-terrorism-and-austria-totalitarianism/.
- 99. Weinstein, “Covid: The Path Not Taken”, https://youtu.be/-zg1j7Zquoc?t=1574; Bret Weinstein, “Bret and Heather 87th DarkHorse Podcast Livestream: We Must Drive this Virus to Extinction”, YouTube, July 13, 2021, https://www.youtube.com/watch?v=QsUvr8s0qEk&t=3472s; Bret Weinstein, “Your Questions Answered – Bret and Heather 109th DarkHorse Podcast Livestream”, YouTube, December 18, 2021, https://www.youtube.com/live/7m1PcdeS-p8?feature=share&t=906.
- 100. Bret Weinstein, “Bret and Heather 66th DarkHorse Podcast Livestream: All Biology Is Evolutionary Biology,” YouTube, February 6, 2021, https://youtu.be/wndotTtBTZQ?t=4150.
- 101. Nathan Green, “Correlation Is Not Causation,” The Guardian, January 6, 2012, https://www.theguardian.com/science/blog/2012/jan/06/correlation-causation; For example, some podcasters highlighted a theory that COVID-19 was less common in African countries because people took hydroxychloroquine for malaria. While this could indicate a clear link between hydroxychloroquine and the prevention of COVID-19, there are many alternative explanations, including the inflow and outflow of people that could also explain an observed relationship.
- 102. “Bret Weinstein,” Social Blade, https://socialblade.com/twitter/user/bretweinstein/monthly.
- 103. “Heather E. Heying,” Social Blade, https://socialblade.com/twitter/user/heathereheying/monthly.
- 104. Bret Weinstein. “Bret and Heather 83rd DarkHorse Podcast Livestream: Doing Science in an Emergency,” YouTube, June 5, 2021, https://www.youtube.com/live/pQiv8I9Peqk?feature=share&t=2867; and The First, “The Buck Sexton Show | Full Hour 2 | 09-10-20,” YouTube, September 10, 2020, https://youtu.be/EWtyYaf5h1Q?t=612.
- 105. Candace Owens, “Those Deemed ‘Conspiracy Theorists’ Have Been Right All Along,” YouTube, June 15, 2021, https://youtu.be/SSDZjosGh1Y?t=202.
- 106. “Submission Guide,” medRxiv, https://www.medrxiv.org/submit-a-manuscript.
- 107. Aleksandra Urman et al. “The politicization of medical preprints on Twitter during the early stages of COVID-19 pandemic,” Journal of Quantitative Description: Digital Media 2 (February 2022): https://journalqd.org/article/view/3007/2447.
- 108. Philip N. Cohen, “Mexico City’s Program to Distribute Ivermectin to 200,000 COVID-19 Patients, and the Bad Science That Propped It Up.” Family Inequality, February 7, 2022, https://familyinequality.wordpress.com/2022/02/06/mexico-citys-program-to-distribute-ivermectin-to-200000-covid-19-patients-and-the-bad-science-that-propped-it-up/.
- 109. Sarah Kreps and Douglas Kriner, “Model uncertainty, political contestation and public trust in science: evidence from the COVID-19 pandemic,” Science Advances 6, no 43 (October 2020): https://www.science.org/doi/10.1126/sciadv.abd4563.
- 110. “Fact check: Outdated video of Fauci saying ‘there’s no reason to be walking around with a mask,’” Reuters, October 8, 2020, https://www.reuters.com/article/uk-factcheck-fauci-outdated-video-masks/fact-checkoutdated-video-of-fauci-saying-theres-no-reason-to-be-walking-around-with-a-mask-idUSKBN26T2TR.
- 111. Ibid.
- 112. BlazeTV, “IMPEACHMENT: Libs Don’t Need FACTS When They Have FEELINGS | The News & Why It Matters | Ep 713,” YouTube, February 9, 2021, https://www.youtube.com/watch?v=l8DwIb_jC5I.
- 113. Fernando P. Polack, Stephen J. Thomas, Nicholas Kitchin, Judith Absalon, Alejandra Gurtman, Stephen Lockhart, John L. Perez, et al. “Safety and efficacy of the BNT162b2 MRNA Covid-19 vaccine,” New England Journal of Medicine 383, no. 27 (December 31, 2020): 2603–2615, https://doi.org/10.1056/NEJMoa2034577.
- 114. Kreps and Kriner. “Model uncertainty, political contestation and public trust in science: evidence from the COVID-19 pandemic.”
- 115. Guy Rosen, “An Update on Our Work to Keep People Informed and Limit Misinformation About COVID-19,” Meta, April 16, 2020, (Last updated: May 26, 2021), https://about.fb.com/news/2020/04/covid-19-misinfo-update/#removing-more-false-claims.
- 116. Editorial Board, “Facebook’s Lab-Leak About-Face,” The Wall Street Journal, May 27, 2021, https://www.wsj.com/articles/facebooks-lab-leak-about-face-11622154198.
- 117. Smitha Khorana and Kellie Owens, “Understanding medical uncertainty in the hydroxychloroquine debate,” The Brookings Institution, July 23, 2020, http://www.brookings.edu/techstream/understanding-medical-uncertainty-in-the-hydroxychloroquine-debate/.
- 118. These recommendations built on those detailed in Valerie Wirtschafter and Chris Meserole, “Policy recommendations for addressing content moderation in podcasts,” The Brookings Institution, April 18, 2022, http://www.brookings.edu/techstream/policy-recommendations-for-addressing-content-moderation-in-podcasts/.
- 119. Nicholas Quah, “Apple might be getting into the podcast-making business. Is its reign as the industry’s benevolent overlord coming to an end?,” Nieman Lab, July 16, 2019, https://www.niemanlab.org/2019/07/apple-might-be-getting-into-the-podcast-making-business-is-its-reign-as-the-industrys-benevolent-overlord-coming-to-an-end/.
- 120. “Content Policies for Google Search,” Google Search Help, https://support.google.com/websearch/answer/10622781?hl=en; “Search Services,” Yahoo, https://legal.yahoo.com/us/en/yahoo/privacy/products/searchservices/index.html; and “Bing Webmaster Guidelines,” Microsoft Bing, https://www.bing.com/webmasters/help/webmaster-guidelines-30fba23a; and Daphne Keller, “Lawful but Awful? Control over Legal Speech by Platforms, Governments, and Internet Users,” The University of Chicago Law Review Online, June 28, 2022, https://lawreviewblog.uchicago.edu/2022/06/28/keller-control-over-speech/.
- 121. “Apple Podcasts Content Guidelines,” Apple Podcasts for Creators, https://podcasters.apple.com/support/891-content-and-subscription-guidelines.
- 122. “Our Ongoing Work to Tackle Hate,” YouTube, June 5, 2019, https://blog.youtube/news-and-events/our-ongoing-work-to-tackle-hate.
- 123. “Podcast Statistics and Data (September 2022),” Buzzsprout, October 4, 2022, https://www.buzzsprout.com/blog/podcast-statistics.
- 124. Daphne Keller, “Amplification and its discontents,” Knight First Institute at Columbia University, June 8, 2021, https://knightcolumbia.org/content/amplification-and-its-discontents.
- 125. “Podcast Notes: The Best Ideas From the World’s Best Podcasts in Minutes,” Podcast Notes, https://podcastnotes.org/.
- 126. Jay Peters, “Reddit is Adding New Real-Time Features Including a Live Upvote Count,” The Verge, December 1, 2021, https://www.theverge.com/2021/12/1/22810850/reddit-real-time-live-upvote-reading-typing-indicator-comment; and “Vote Up,” Stack Overflow, https://stackoverflow.com/help/privileges/vote-up.
- 127. Bryan Menegus, “Reddit Is Tearing Itself Apart,” Gizmodo, November 29, 2016, https://gizmodo.com/reddit-is-tearing-itself-apart-1789406294; and “Wikipedia: Blocking Policy,” Wikipedia, December 8, 2022, https://en.wikipedia.org/w/index.php?title=Wikipedia:Blocking_policy&oldid=1126307979.
- 128. “The Digital Services Act: Ensuring a Safe and Accountable Online Environment,” European Commission, https://commission.europa.eu/strategy-and-policy/priorities-2019-2024/europe-fit-digital-age/digital-services-act-ensuring-safe-and-accountable-online-environment_en; and John Perrino, “The Platform Accountability and Transparency Act: take two,” Tech Policy Press, December 22, 2022, https://techpolicy.press/the-platform-accountability-and-transparency-act-take-two/.
- 129. “Content prohibited on Spotify,” Spotify, https://support.spotifyforpodcasters.com/hc/en-us/articles/360047226672-Content-prohibited-on-Spotify; “Content Requirements: Apple Podcasts content guidelines,” Apple Podcasts, https://podcasters.apple.com/support/891-content-and-subscription-guidelines.
- 130. “Sponsorship Identification Rules,” Federal Communications Commission, January 13, 2021, https://www.fcc.gov/consumers/guides/sponsorship-identification-rules; and “FTC Proposes to Strengthen Advertising Guidelines Against Fake and Manipulated Reviews,” Federal Trade Commission, May 19, 2022, https://www.ftc.gov/news-events/news/press-releases/2022/05/ftc-proposes-strengthen-advertising-guidelines-against-fake-manipulated-reviews.
- 131. Brianna Keilar, “Video: Joe Rogan Gets Fact-Checked in Real Time,” CNN Business, https://www.cnn.com/videos/media/2022/01/14/joe-rogan-fact-check-podcast-covid-19-vaccine-newday-vpx.cnn.
- 132. Jonathan Glick, “Rise of the Platishers,” Vox, February 7, 2014, https://www.vox.com/2014/2/7/11623214/rise-of-the-platishers.
- 133. Jacob Kastrenakes, “Substack Makes a Pitch for Your Podcasts,” The Verge, April 5, 2022, https://www.theverge.com/2022/4/5/23011365/substack-podcasts-daniel-ek-spotify-parcast-union-negotiations.
Acknowledgments:
I would like to thank Chris Meserole, Jessica Brandt, Elias Groll, Derek Belle and two anonymous reviewers for their invaluable support of this project, and Melody Chen, Adya Danaditya, Dylan Hanson, Sharanya Majumder, and Isabelle Sharon for their exceptional research assistance. Thanks also to Aaron Rudkin for the initial spark to kickstart the data collection process vital to this project, Chad Hazlett for technical guidance, and Andy Bailor for the data pipeline support. Eric Abalahin, Ryan DeVries, Alex Dimsdale, and Paula Kostiuk were instrumental in publishing this report, and Molly Escobar and Shavanthi Mendis designed and programmed the beautiful interactive figures that help bring the data to life. All errors are my own.